Data Analysis for Predictive Maintenance Using Time Series and Deep Learning Models—A Case Study in a Pulp Paper Industry
Proceedings of IncoME-VI and TEPEN 2021(2022)
Abstract
Predictive maintenance is fundamental for modern industries, in order to improve the physical assets availability, decision making and rationalize costs. That requires deployment of sensor networks, data storage and development of data treatment methods that can satisfy the quality required in the forecasting models. The present paper describes a case study where data collected in an industrial pulp paper press was pre-processed and used to predict future behavior, aiming to anticipate potential failures, optimize predictive maintenance and physical assets availability. The data were processed and analyzed, outliers identified and treated. Time series models were used to predict short-term future behavior. The results show that it is possible to predict future values up to ten days in advance with good accuracy.
MoreTranslated text
Key words
Data analysis, Autoregressive models, ARIMA, Deep Learning, Time series forecasting, Predictive maintenance
AI Read Science
Must-Reading Tree
Example
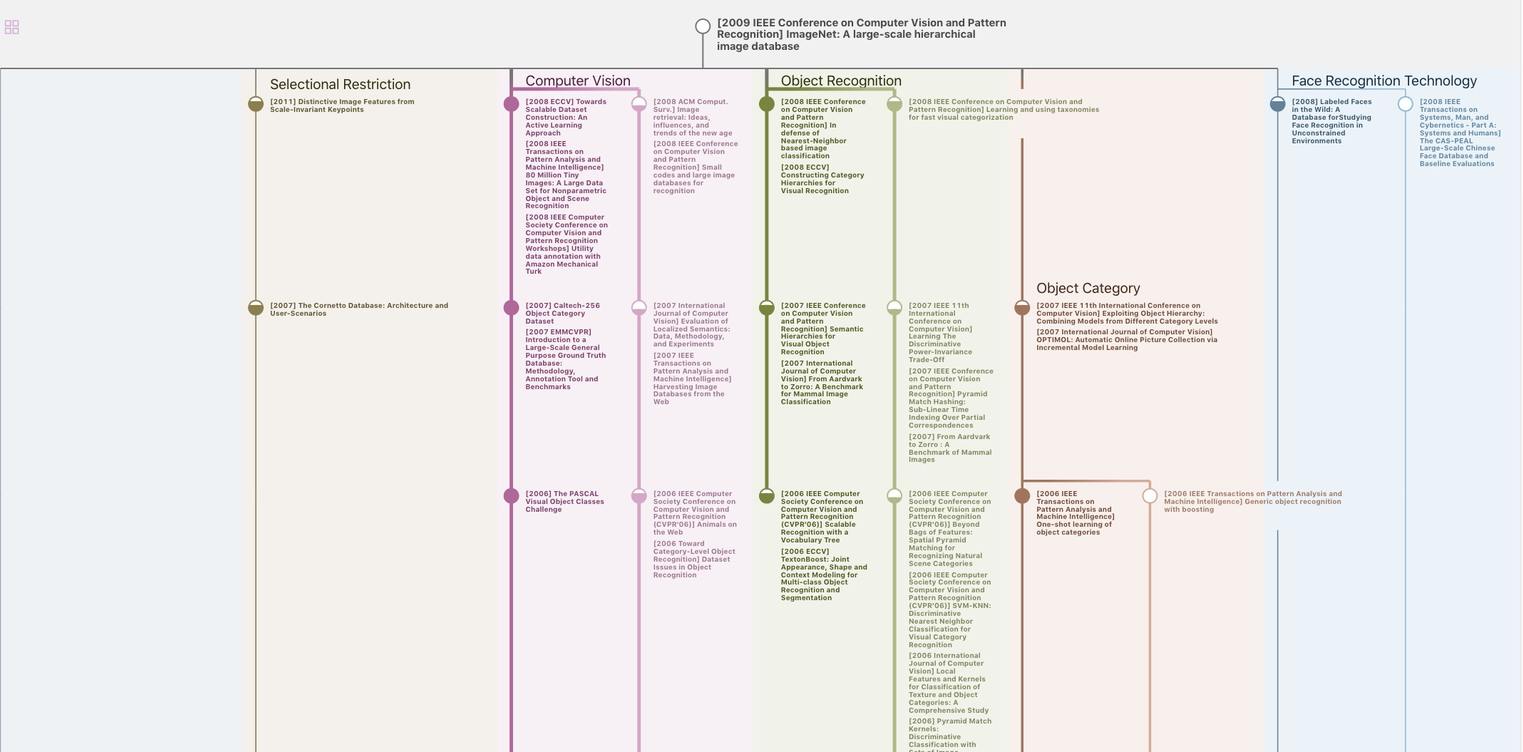
Generate MRT to find the research sequence of this paper
Chat Paper
Summary is being generated by the instructions you defined