Adaptive Shapelets Preservation for Time Series Augmentation
IEEE International Joint Conference on Neural Network (IJCNN)(2022)
摘要
Time series augmentation is an essential technique in training deep learning models for time series, especially achieving remarkable results in tackling the overfitting problems. However, existing methods fail to specifically protect discriminative features that contribute significantly to the classification results, so these features may be destroyed during the augmentation process. This leads to lower fidelity of augmented time series, which ultimately interferes with classification decisions during inference. To address this issue, we propose an adaptive shapelets preservation approach, named ASP. First, we exploit the saliency map to detect shapelets on the original time series that contain discriminative features. Second, we preserve them during augmentation and assign a proprietary label to each time series. It improves the fidelity of augmented time series and the confidence of their labels, thereby avoiding the risk of interfering with classification decisions. Experimental results on 128 datasets of the UCR2018 archive show that our method ASP outperforms that without augmentation on 98 datasets, and helps the classifier achieve the average accuracy improvement from 71.26% to 75.46%, which is far better than the state-of-the-art approaches.
更多查看译文
关键词
Shapelets Preservation,Time Series Augmentation,Time Series Classification,Cross Entropy,Adaptive,CAM
AI 理解论文
溯源树
样例
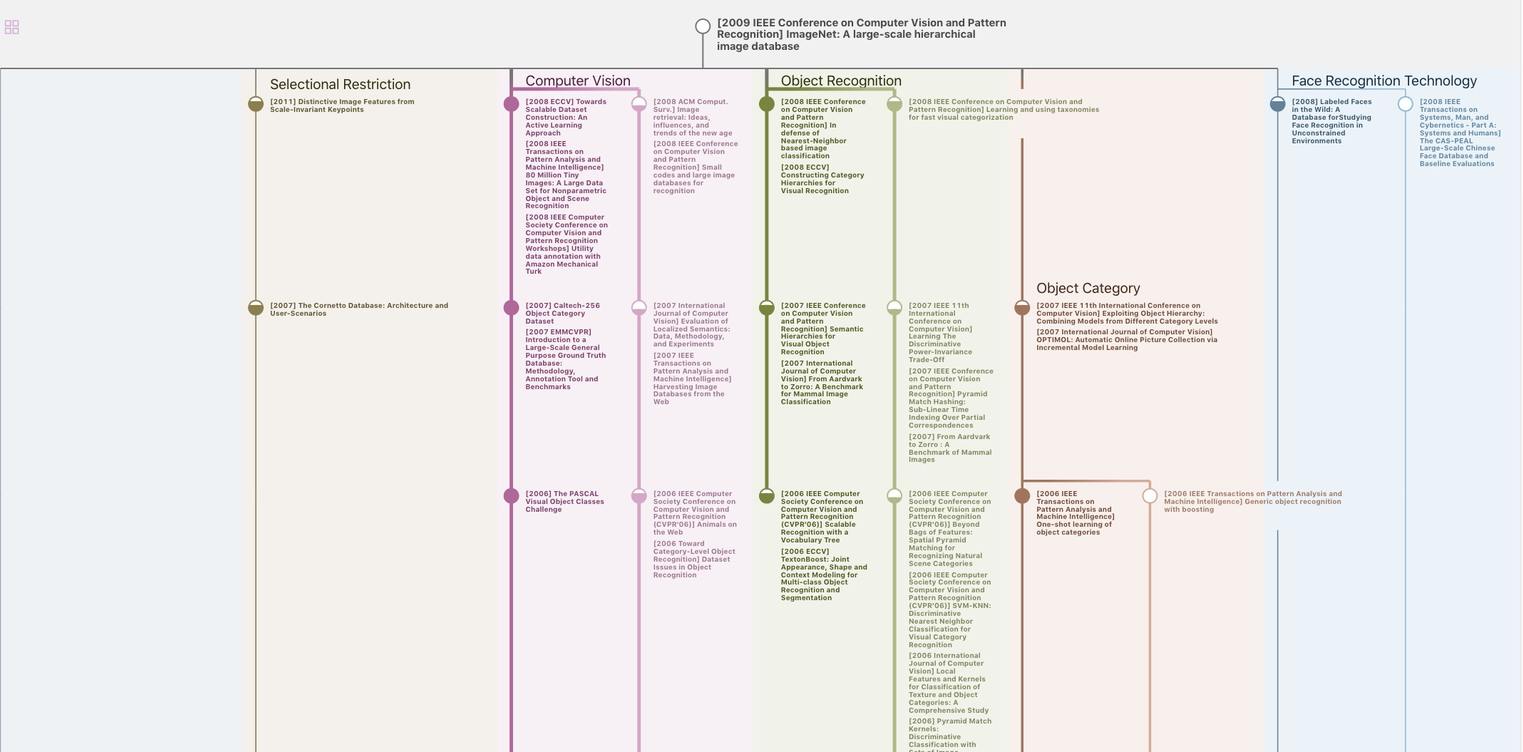
生成溯源树,研究论文发展脉络
Chat Paper
正在生成论文摘要