Learning Knowledge Graph Embedding with Batch Circle Loss
2022 International Joint Conference on Neural Networks (IJCNN)(2022)
Abstract
Knowledge Graph Embedding (KGE) is the process to learn low-dimension representations for entities and relations in knowledge graphs. It is a critical component in Knowledge Graph (KG) for link prediction and knowledge discovery. Many works focus on designing proper score function for KGE, while the study of loss function has attracted relatively less attention. In this paper, we focus on improving the loss function when learning KGE. Specifically, we find that the frequently used margin-based loss in KGE models seeks to maximize the gap between the true facts score f
p
and the false facts score f
n
and only cares about the relative order of scores. Since its optimization objective is f
p
- f
n
= m, increasing f
p
is equivalent to decreasing f
n
. Its optimization objective creates an ambiguous convergence status which impairs the separability of positive and negative facts in embedding space. Inspired by the circle loss that offers a more flexible optimization manner with definite convergence targets and is widely used in computer vision tasks, we further extend it into the KGE model with the presented Batch Circle Loss (BCL). BCL allows multiple positives to be considered per anchor (h, r) (or (r, t)) in addition to multiple negatives (as opposed to a single positive sample as used before in KGE models). By comparing with other approaches, the obtained KGE models using our proposed loss function and training method shows superior performance.
MoreTranslated text
Key words
knowledge graph embedding,link prediction,loss function
AI Read Science
Must-Reading Tree
Example
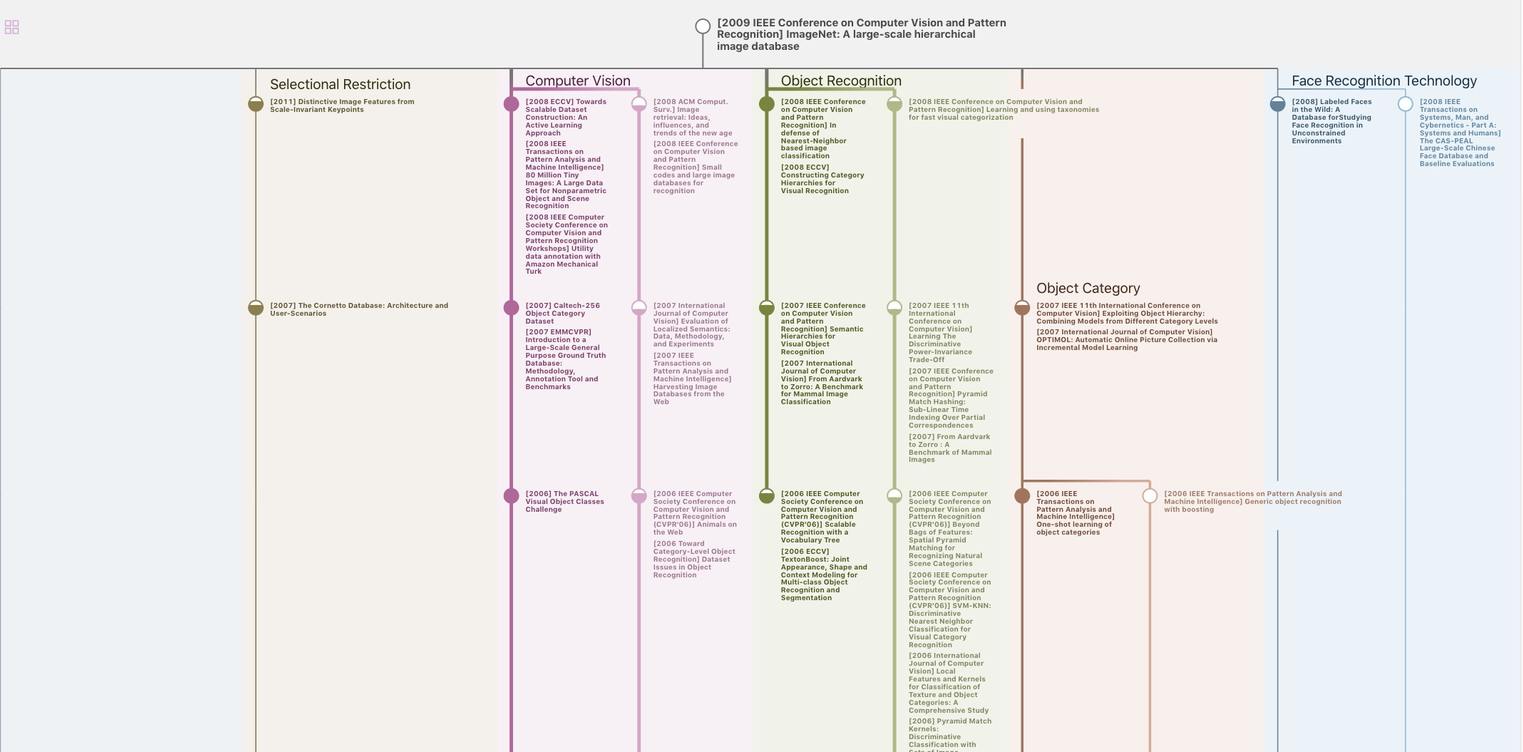
Generate MRT to find the research sequence of this paper
Chat Paper
Summary is being generated by the instructions you defined