Self-supervised Depth Estimation from Spectral Consistency and Novel View Synthesis
IEEE International Joint Conference on Neural Network (IJCNN)(2022)
摘要
Single image depth estimation is a critical issue for robot vision, augmented reality, and many other applications when an image sequence is not available. Self-supervised single image depth estimation models target at predicting accurate disparity map just from one single image without ground truth supervision or stereo image pair during real applications. Compared with direct single image depth estimation, single image stereo algorithm can generate the depth from different camera perspectives. In this paper, we propose a novel architecture to infer accurate disparity by leveraging both spectral-consistency based learning model and view-prediction based stereo reconstruction algorithm. Direct spectral-consistency based method can avoid false positive matching in smooth regions. Single image stereo can preserve more distinct boundaries from another camera perspective. By learning confidence maps and designing a fusion strategy, the two disparities from the two approaches are able to be effectively fused to produce the refined disparity. Extensive experiments and ablations indicate that our method exploits both advantages of spectral consistency and view prediction, especially in constraining object boundaries and correcting wrong predicting regions.
更多查看译文
关键词
depth estimation,view,spectral consistency,self-supervised
AI 理解论文
溯源树
样例
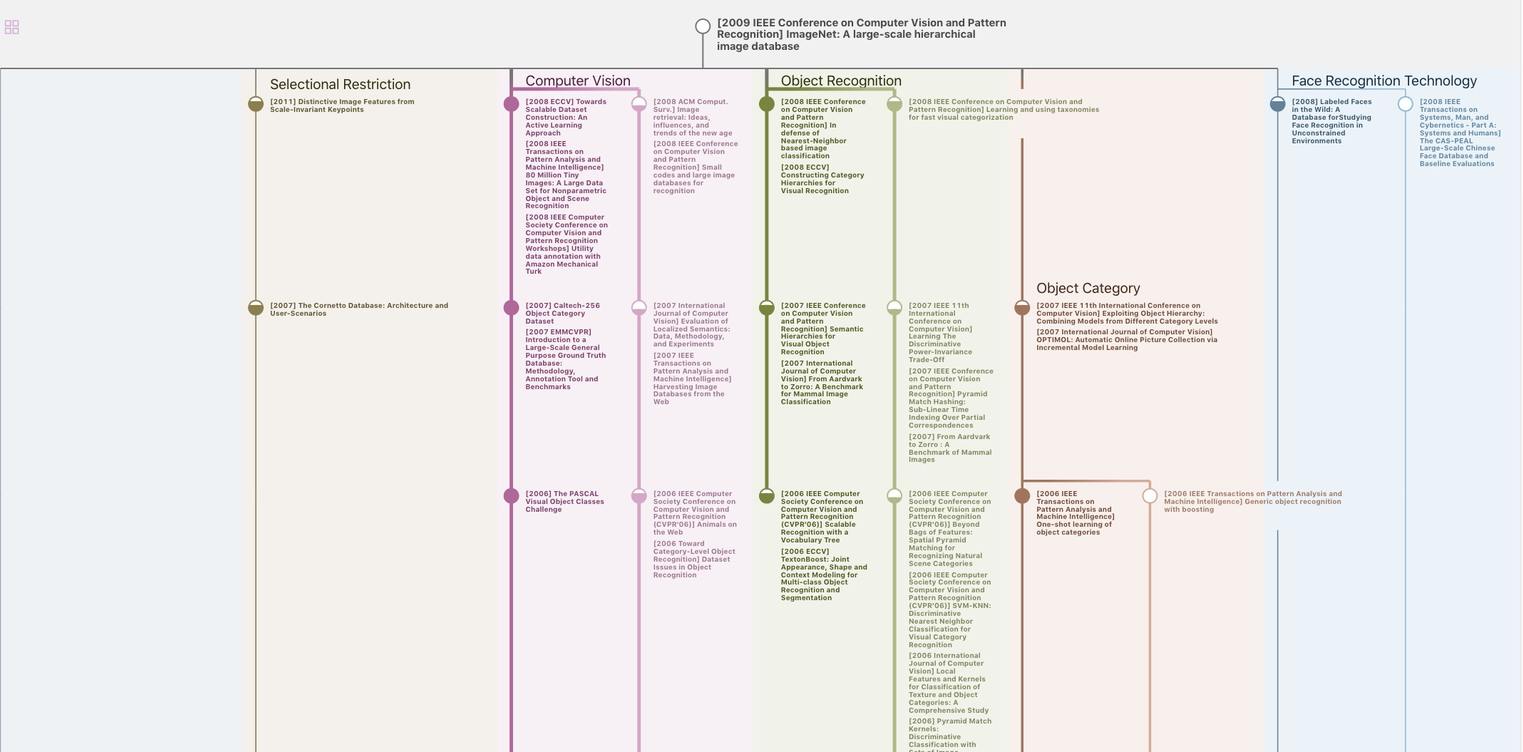
生成溯源树,研究论文发展脉络
Chat Paper
正在生成论文摘要