DeepCADD: A Deep Learning Architecture for Automatic Detection of Coronary Artery Disease
2022 International Joint Conference on Neural Networks (IJCNN)(2022)
摘要
Cardiovascular disease (CVD) is one of the main causes of death in the world. Coronary artery disease (CAD) is one of the CVD most common disorders. CAD is mainly caused by restrictions in the heart muscle blood flow supply, called atherosclerosis. The assessment of atherosclerosis is challenging and is currently primarily achieved with angiography, which is the gold standard for geometrical assessment. The angiography is completely dependent on the physician's lesion identification and visual assessment. In this paper, we tackle this problem by proposing an architecture for automatic lesion detection, called DeepCADD. DeepCADD proposes the use of instance segmentation, replaces an original Mask R-CNN's backbone, and trains the classification layer with an angiography dataset. The backbone was replaced by a ResNet-50 pre-trained with a dataset with 2,000 images of coronary artery segments. Powered by the skip connections from ResNet-50 network which propagates small image features to deeper layers. DeepCADD is comparable with the gold standard and we discussed the performance with similar studies. Moreover, we performed a validation with specialists to understand the architecture's real performance. Our results show that DeepCADD presents a good performance in the lesion identification, reducing the false negatives and reaching a sensitivity of approximately 0.89. These pieces of evidence suggest that DeepCADD can be used as a screening tool, contributing to the narrowed lesions identification, playing a crucial role in the angiography protocol automation.
更多查看译文
关键词
angiography,Mask R-CNN,coronary artery
AI 理解论文
溯源树
样例
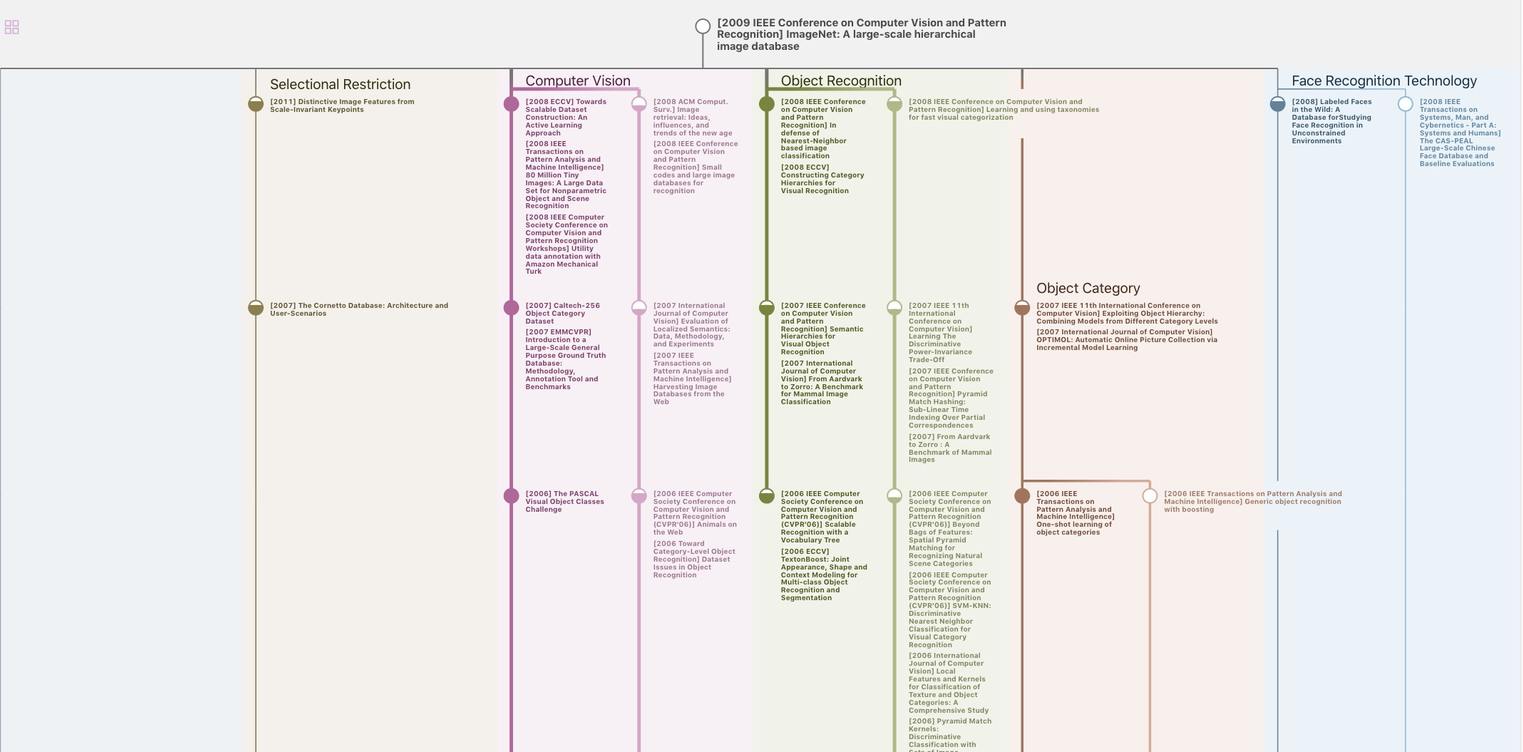
生成溯源树,研究论文发展脉络
Chat Paper
正在生成论文摘要