Semantic VL-BERT: Visual Grounding via Attribute Learning
IEEE International Joint Conference on Neural Network (IJCNN)(2022)
摘要
In recent years, Smart Home Assistants have expanded into tens of thousands of devices and transformed from a voice only assistant to a much more versatile smart assistant, that uses a connected display to provide a multi-modal customer experience. In order to further improve on the multi-modality experience, comprehension systems need models that can work with multisensory inputs. We focus on the problem of visual grounding, which allows customers to interact with and manipulate items displayed on a screen via voice. We propose a novel learning approach that improves upon a lightweight single stream transformer architecture by adjusting it to better align the visual input features with the referring expressions. Our approach learns to cluster parts of the image along spatial and channel dimensions based on descriptive attributes in the query, and takes advantage of the information in separate clusters more efficiently, as demonstrated by a 1.32% absolute accuracy improvement on a public dataset over the baseline. Given that modern-day Smart Home Assistants have very stringent memory and latency requirements, we restrict our focus to a family of lightweight single stream transformer architectures - our focus is not to beat the ever improving state-of-the-art in visual grounding but to improve upon a lightweight transformer architecture which leads to a model that is easy to train and deploy while having improved semantic awareness.
更多查看译文
关键词
vision,language,multi-modality,single stream transformer
AI 理解论文
溯源树
样例
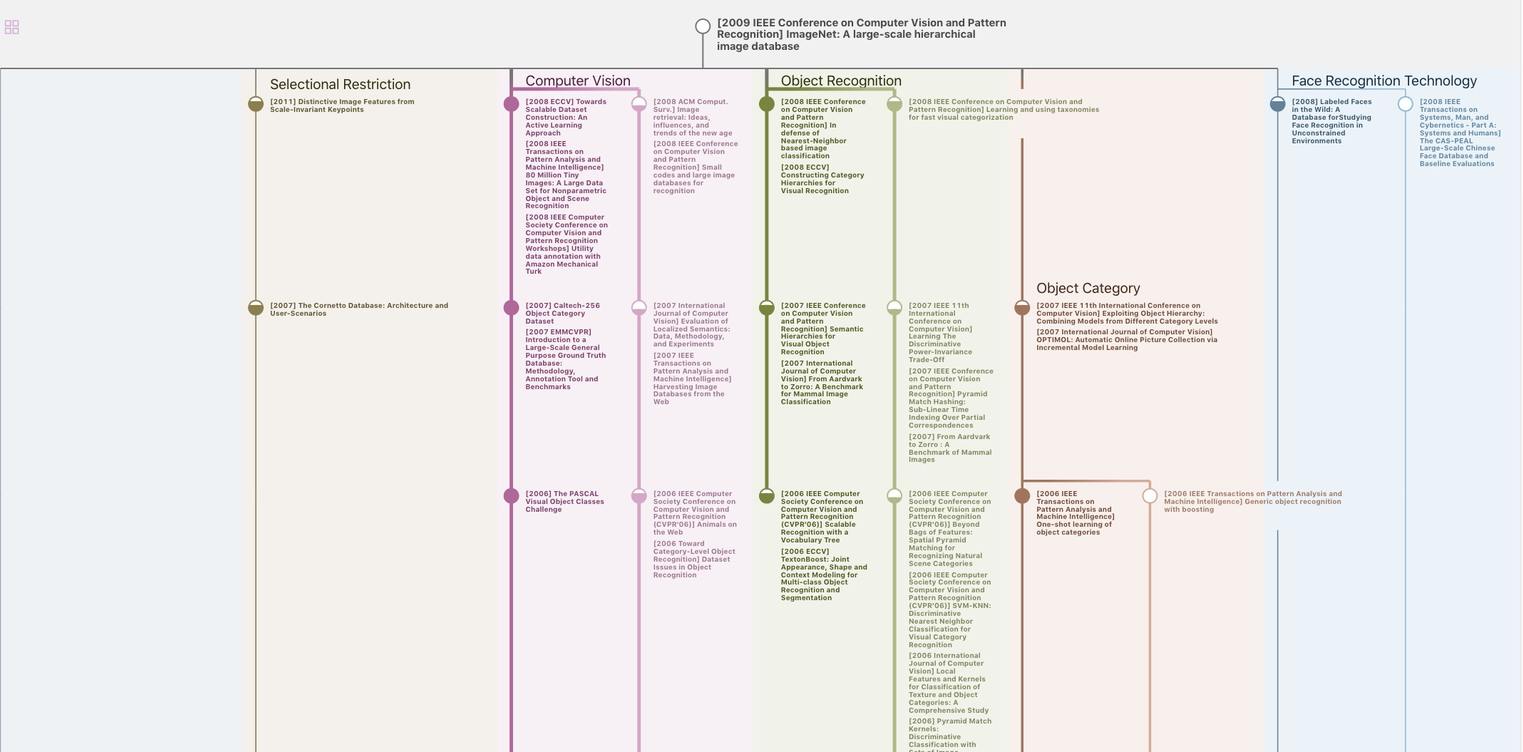
生成溯源树,研究论文发展脉络
Chat Paper
正在生成论文摘要