Meta-learning from Learning Curves: Challenge Design and Baseline Results
IEEE International Joint Conference on Neural Network (IJCNN)(2022)
摘要
Meta-learning has been widely studied and implemented in many Automated Machine Learning systems to improve the process of selecting and training Machine Learning models for new tasks, by leveraging expertise acquired on previously observed tasks. We design a novel meta-learning challenge aiming at learning-to-learn from one of the most essential model evaluation data, the learning curve. It consists of multiple model evaluations collected during the process of training. A metalearner is expected to apply a learned policy to learning curves of partially trained models on the task at hand, to rapidly find the best task solution, without training all potential models to convergence. This implies learning the exploration-exploitation trade-off. Our challenge is split into two phases: a development phase and a final test phase. In each phase, a meta-learner is meta-trained and meta-tested on validation learning curves (development phase) or test learning curves (final test phase). During meta-training, the meta-learner is allowed to learn from the provided learning curves in any possible way. In meta-testing, we borrowed the common Reinforcement Learning setting in which an agent (a meta-learner) learns by interacting with an environment storing pre-computed learning curves. A metalearner must pay a cost (corresponding to the actual training and testing time) to reveal learning curve information progressively. The meta-learner is evaluated and ranked based on the average area under its learning curves. This challenge was accepted as part of the official selection of WCCI 2022 competitions.
更多查看译文
关键词
AutoML,machine learning,meta-learning,learning curves,learning to learn,reinforcement learning.
AI 理解论文
溯源树
样例
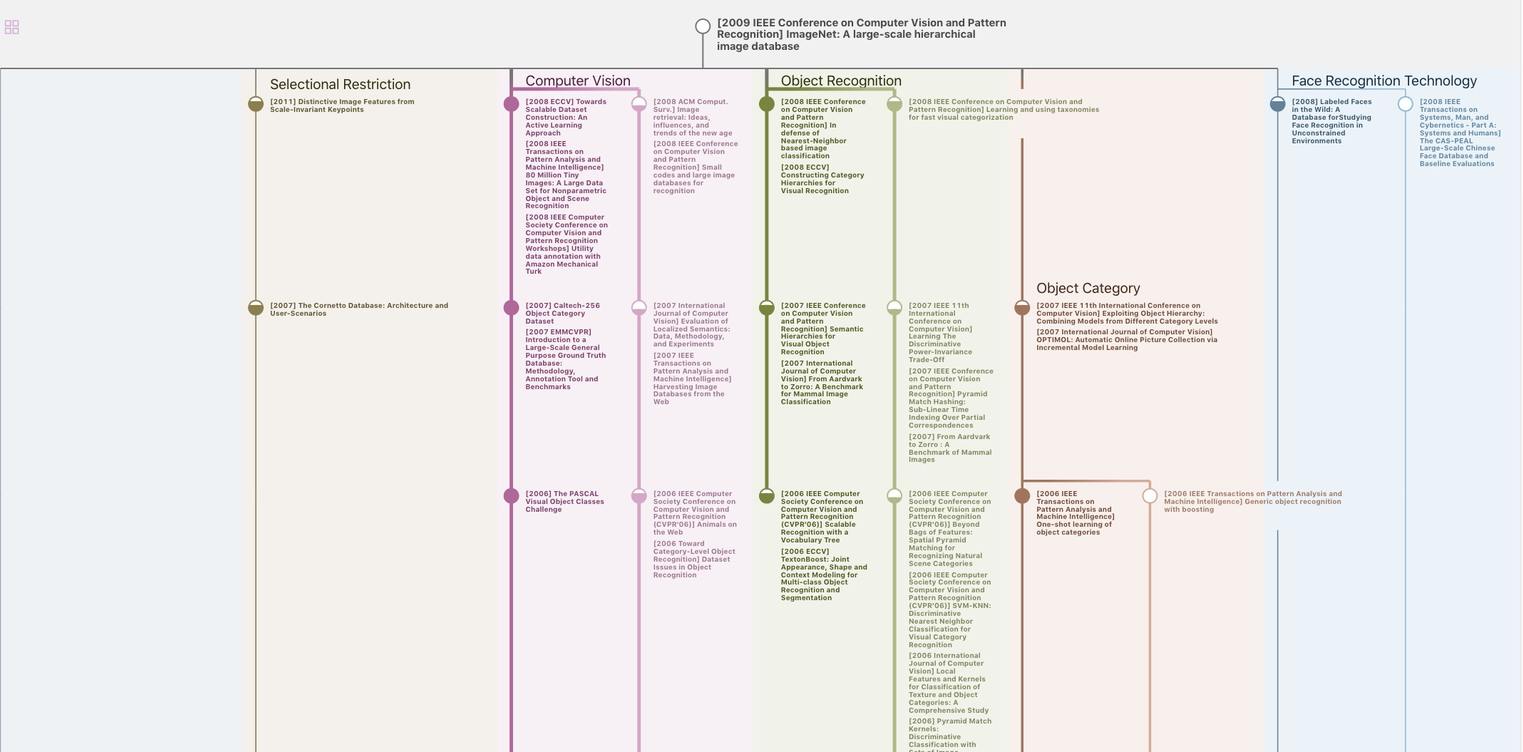
生成溯源树,研究论文发展脉络
Chat Paper
正在生成论文摘要