A Robust De-noising Method via Training Loss for Distantly Supervised Relation Extraction
2022 International Joint Conference on Neural Networks (IJCNN)(2022)
摘要
Distant supervision (DS) is widely used in relation extraction which can automatically generate large-scale training data by aligning a knowledge base with an unlabeled corpus. However, it suffers from the label noise problem. In this paper, we propose a novel explicit training loss based DS relation extraction de-noising method to generate a cleansed dataset. Specifically, we firstly design a noise detector to select noisy bags using average loss during cyclical training, which is based on the idea that the noisy samples will have different loss variation process during training compared to the clean samples. Then we propose a label corrector to generate right labels for the selected samples, which can keep more useful information to a great extent. Finally, the experimental results show that our de-noising method is robust that can detect the label noise quite well on both large scale and small scale dataset and the generated cleansed dataset significantly improves the performance of previous distant supervision models.
更多查看译文
关键词
Relation extraction,Distant supervision,Cyclical training,Training loss
AI 理解论文
溯源树
样例
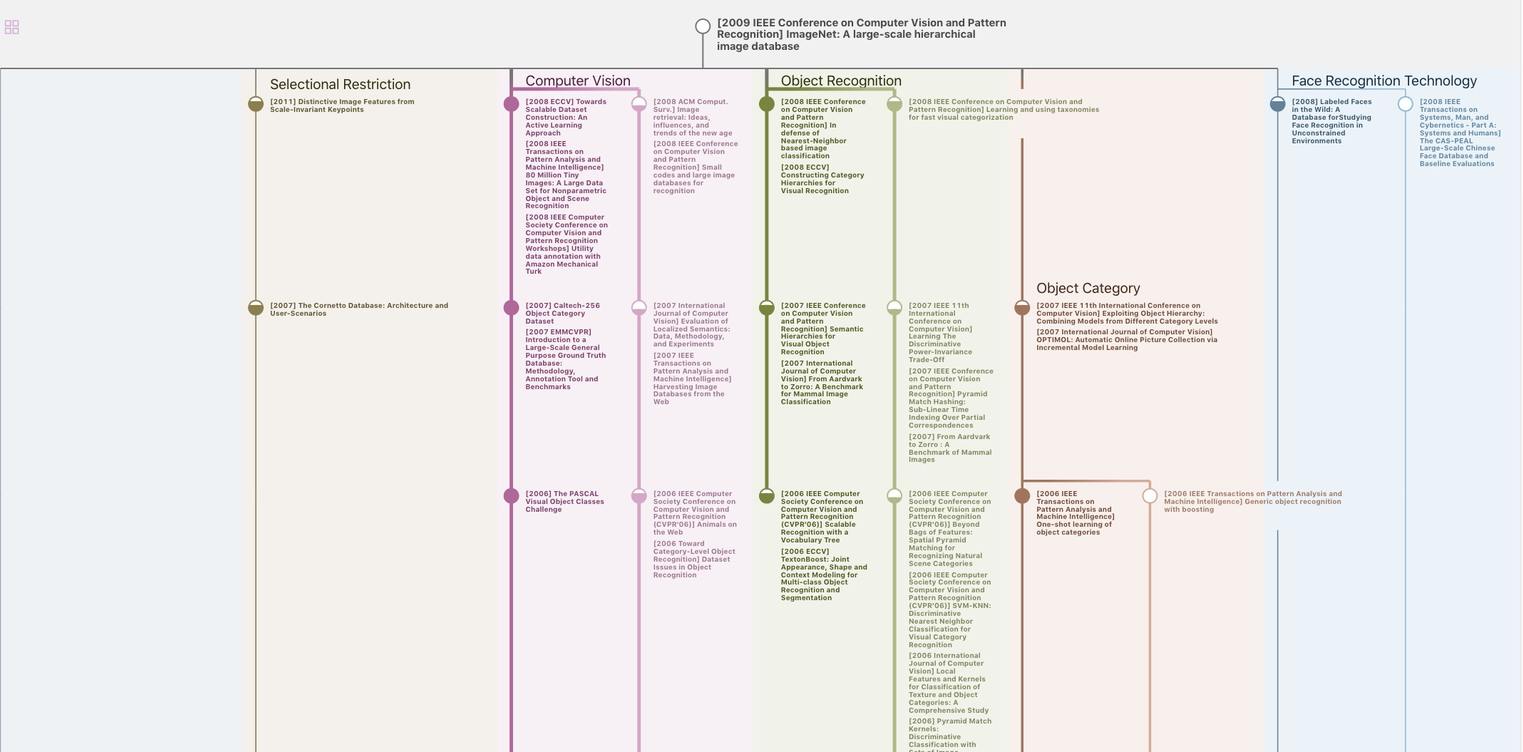
生成溯源树,研究论文发展脉络
Chat Paper
正在生成论文摘要