Local-Diversity Evaluation Assignment Strategy for Decomposition-Based Multiobjective Evolutionary Algorithm
IEEE Transactions on Systems, Man, and Cybernetics: Systems(2023)
摘要
Decomposition-based multiobjective evolutionary algorithms (MOEAs) transform a multiobjective optimization problem (MOP) into a set of subproblems and then optimize them collaboratively. When two adjacent objective vectors are similar but the difference in the corresponding decision vectors is large, it is difficult to transfer from a solution of one subproblem to solutions of its neighboring subproblems. In this article, we argue that the key to overcoming this difficulty is the evaluation assignment for different subproblems based on local density and global utility. We propose a local-density measurement model to estimate the solution density around each subproblem. Based on the model, a local-diversity evaluation assignment strategy for the decomposition-based MOEA is designed to assign fitness evaluations among different subproblems. Two discrete MOPs are selected as test instances for experiments because they satisfy the premise that the difference in the corresponding decision vectors of adjacent objective vectors is large. Experimental results indicate that the proposed strategy enhances the population diversity. Besides, the combination of the proposed strategy and the evaluation assignment for high global utility subproblems can overcome the difficulty in transferring from a solution of one subproblem to solutions of its neighboring subproblems. The results also verify that the proposed algorithm significantly outperforms the state-of-the-art evaluation assignment MOEAs regarding the inverted generational distance and the hypervolume metrics.
更多查看译文
关键词
Decomposition-based multiobjective evolutionary algorithms (MOEAs),discrete multiobjective optimization problems (MOPs),local-density measurement,local-diversity evaluation assignment (LdEA)
AI 理解论文
溯源树
样例
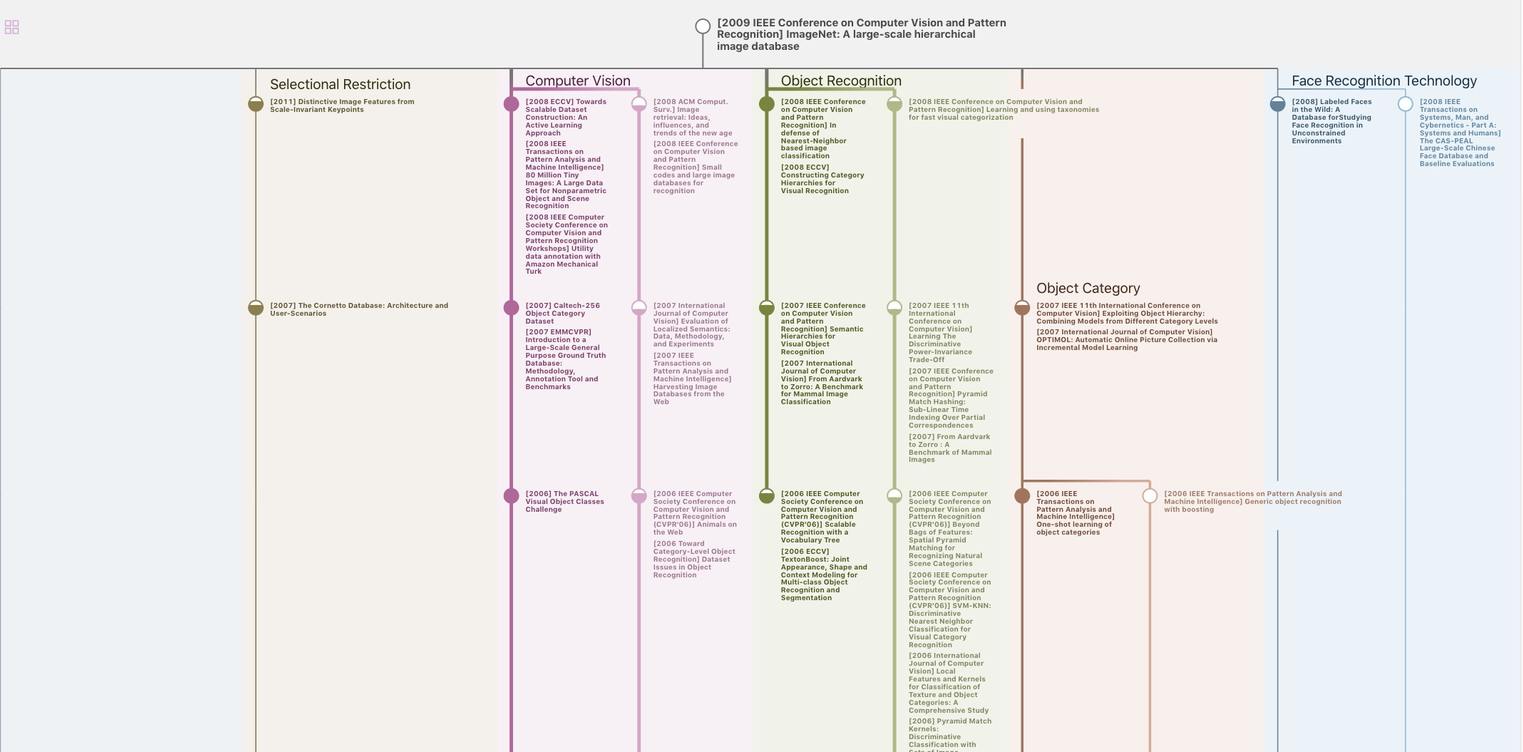
生成溯源树,研究论文发展脉络
Chat Paper
正在生成论文摘要