Wavefront sensor fusion via shallow decoder neural networks for aero-optical predictive control
Interferometry XXI(2022)
摘要
Sensor limitations often result in devices with particularly high spatial-imaging resolution or high sampling rates but not both concurrently. Adaptive optics control mechanisms, for example, rely on high-fidelity sensing technology to predictively correct wavefront phase aberrations. We propose fusing these two categories of sensors: those with high spatial resolution and those with high temporal resolution. As a prototype, we first sub-sample simulations of the Kuramoto-Sivashinsky equation, known for its chaotic flow from diffusive instability, and build a map between such simulated sensors using a Shallow Decoder Neural Network. We then examine how to fuse the merits of a common sensor in aero-optical sensing, the Shack-Hartmann wavefront sensor, with the increased spatial information of a Digital Holography wavefront sensor, training on supersonic wind-tunnel wavefront data provided by the Aero-Effects Laboratory at the Air Force Research Laboratory Directed Energy Directorate. These maps merge the high-temporal and high-spatial resolutions from each respective sensor, demonstrating a proof-of-concept for wavefront sensor fusion for adaptive optical applications.
更多查看译文
关键词
aero-optics, optics, photonics, neural networks, Kuramoto-Sivashinsky
AI 理解论文
溯源树
样例
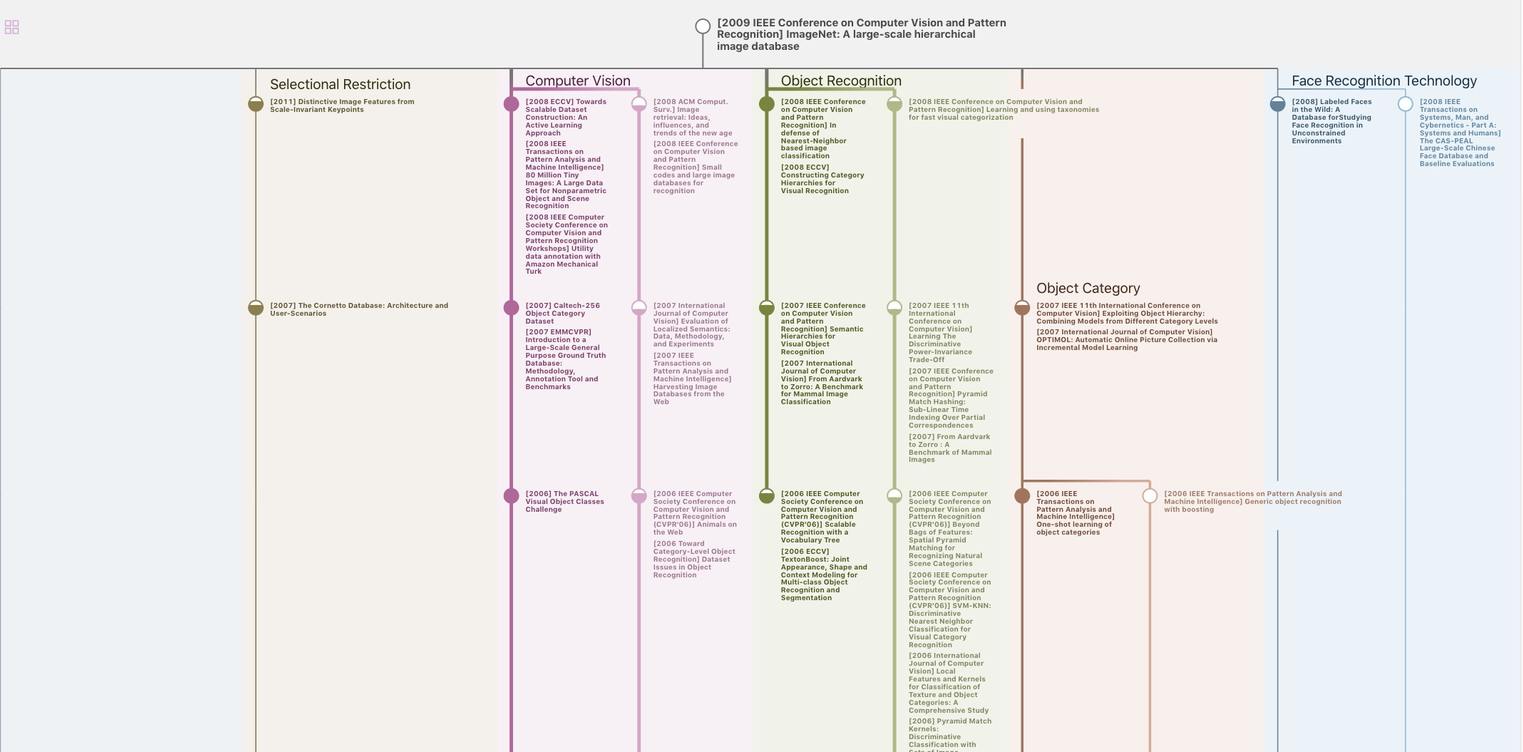
生成溯源树,研究论文发展脉络
Chat Paper
正在生成论文摘要