Quantifying Mutational Response to Track the Evolution of SARS-CoV-2 Spike Variants: Introducing a Statistical-Mechanics-Guided Machine Learning Method
JOURNAL OF PHYSICAL CHEMISTRY B(2022)
摘要
The emergence of SARS-CoV-2 and its variants that critically affect global public health requires characterization of mutations and their evolutionary pattern from specific Variants of Interest (VOIs) to Variants of Concern (VOCs). Leveraging the concept of equilibrium statistical mechanics, we introduce a new responsive quantity defined as "Mutational Response Function (MRF)" aptly quantifying domain-wise average entropy-fluctuation in the spike glycoprotein sequence of SARS-CoV-2 based on its evolutionary database. As the evolution transits from a specific variant to VOC, we find that the evolutionary crossover is accompanied by a dramatic change in MRF, upholding the characteristic of a dynamic phase transition. With this entropic information, we have developed an ancestral-based machine learning method that helps predict future domain-specific mutations. The feedforward binary classification model pinpoints possible residues prone to future mutations that have implications for enhanced fusogenicity and pathogenicity of the virus. We believe such MRF analyses followed by a statistical mechanics augmented ML approach could help track different evolutionary stages of such species and identify a critical evolutionary transition that is alarming.
更多查看译文
关键词
spike,mutational response,machine learning,sars-cov,statistical-mechanics-guided
AI 理解论文
溯源树
样例
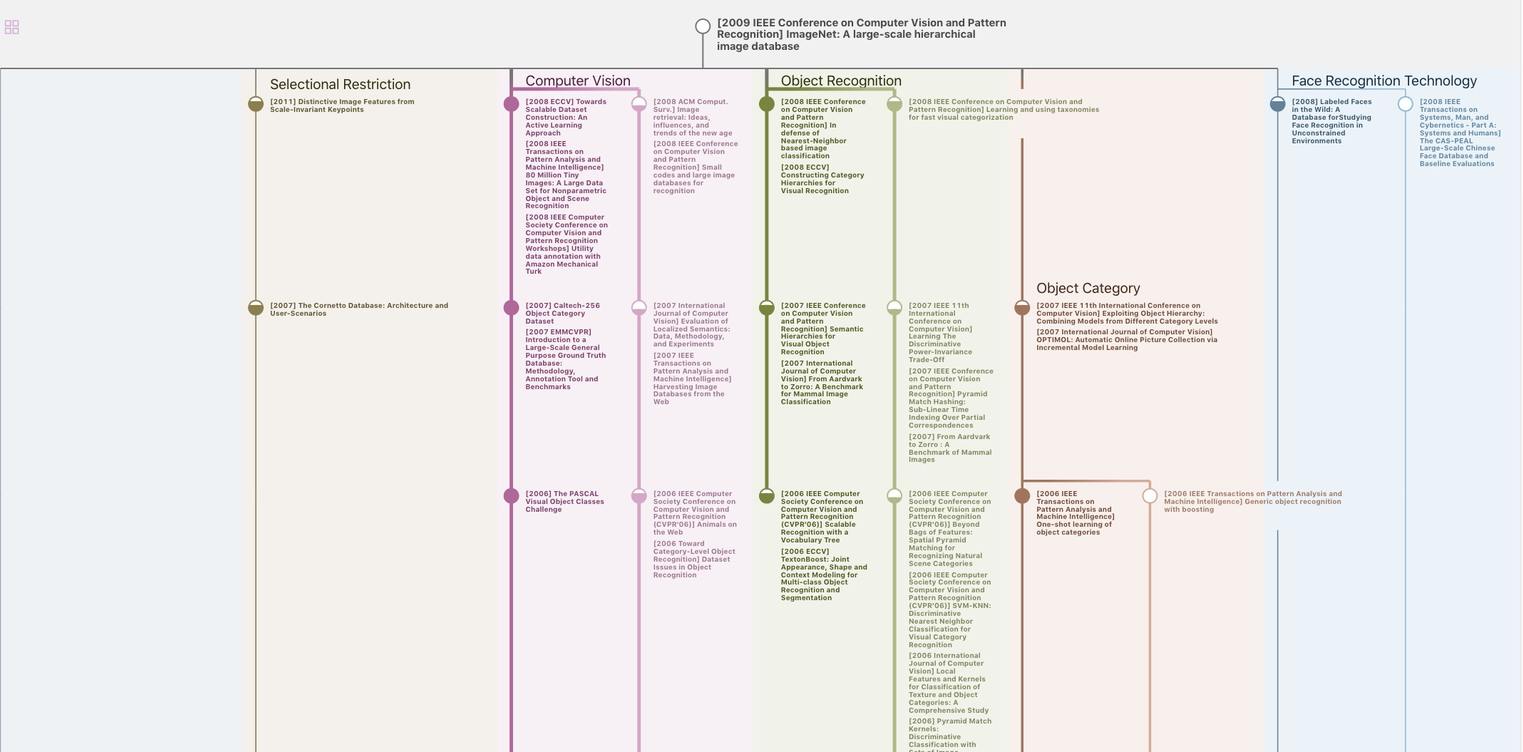
生成溯源树,研究论文发展脉络
Chat Paper
正在生成论文摘要