D-AnoGAN: Anomaly Detection in Disconnected Data Manifolds with Generative Adversarial Networks
2022 International Joint Conference on Neural Networks (IJCNN)(2022)
摘要
We present a novel GAN-based method, D-AnoGAN, for unsupervised anomaly detection in multi-class, disconnected data manifolds. Current state of the art methods learn the non-anomalous data as a single, continuous manifold, limiting their use in multi-class problems. The key contribution of D-AnoGAN is specifically accounting for potential discontinuities between manifolds through clustering. To achieve this, we implement a multi-generator network, where each generator is responsible for learning a unique manifold of data. We also implement a bandit to find the optimal set of generators required to cover all data manifolds through unsupervised prior-learning. Finally, we use this multi-generator and bandit within a GAN architecture to reconstruct queried images using distinct manifolds, ensuring that queries falling outside these manifolds are labeled as anomalous. We demonstrate our method's effectiveness on three publicly available datasets. We also introduce a software package for producing fully parameterized, disconnected manifold datasets of simulated automobile wheel images. We use this software to further show D-AnoGAN's anomaly detection capabilities in a real-world application.
更多查看译文
关键词
disconnected data manifolds,generative adversarial networks,novel GAN-based method,unsupervised anomaly detection,nonanomalous data,single manifold,continuous manifold,multiclass problems,multigenerator network,unsupervised prior-learning,distinct manifolds,disconnected manifold datasets,D-AnoGAN's anomaly detection capabilities
AI 理解论文
溯源树
样例
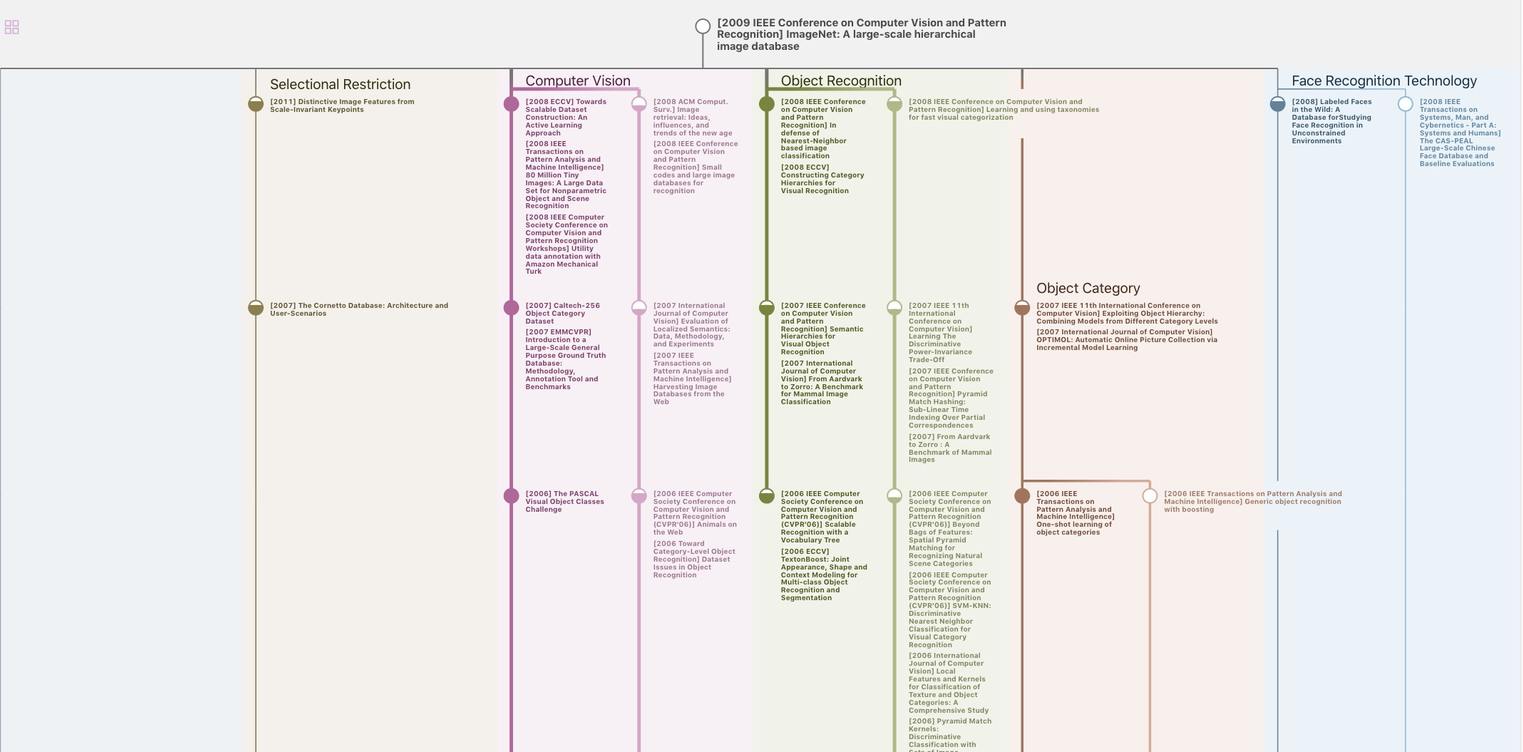
生成溯源树,研究论文发展脉络
Chat Paper
正在生成论文摘要