A benchmark for multi-class object counting and size estimation using deep convolutional neural networks.
Eng. Appl. Artif. Intell.(2022)
摘要
Automatic object counting and object size estimation in digital images can be very useful in many real -world applications such as surveillance, smart farming, intelligent traffic systems, etc. However, most existing research mainly focus on scenarios where only one type of object is considered due to the lack of proper datasets. Furthermore, they use the traditional detection algorithms for size estimation and can only do segmenting tasks but cannot identify different types of objects and return corresponding individual size information. To fill these gaps, we create a synthetic dataset and propose a benchmark for multi-class object counting and size estimation (MOCSE) within a unified framework. We create the dataset MOCSE13 by using Unity to generate synthetic images for 13 different objects (fruits and vegetables). Besides, we propose a deep architecture approach for multi-class object counting and object size estimation. Our proposed models with different backbones are evaluated on the synthetic dataset. The experimental results provide a benchmark for multi-class object counting and size estimation and the synthetic dataset can be served as a proper testbed for future studies.
更多查看译文
关键词
Multi-class object counting,Crowd counting,Object size estimation,Convolutional neural networks,Synthetic dataset
AI 理解论文
溯源树
样例
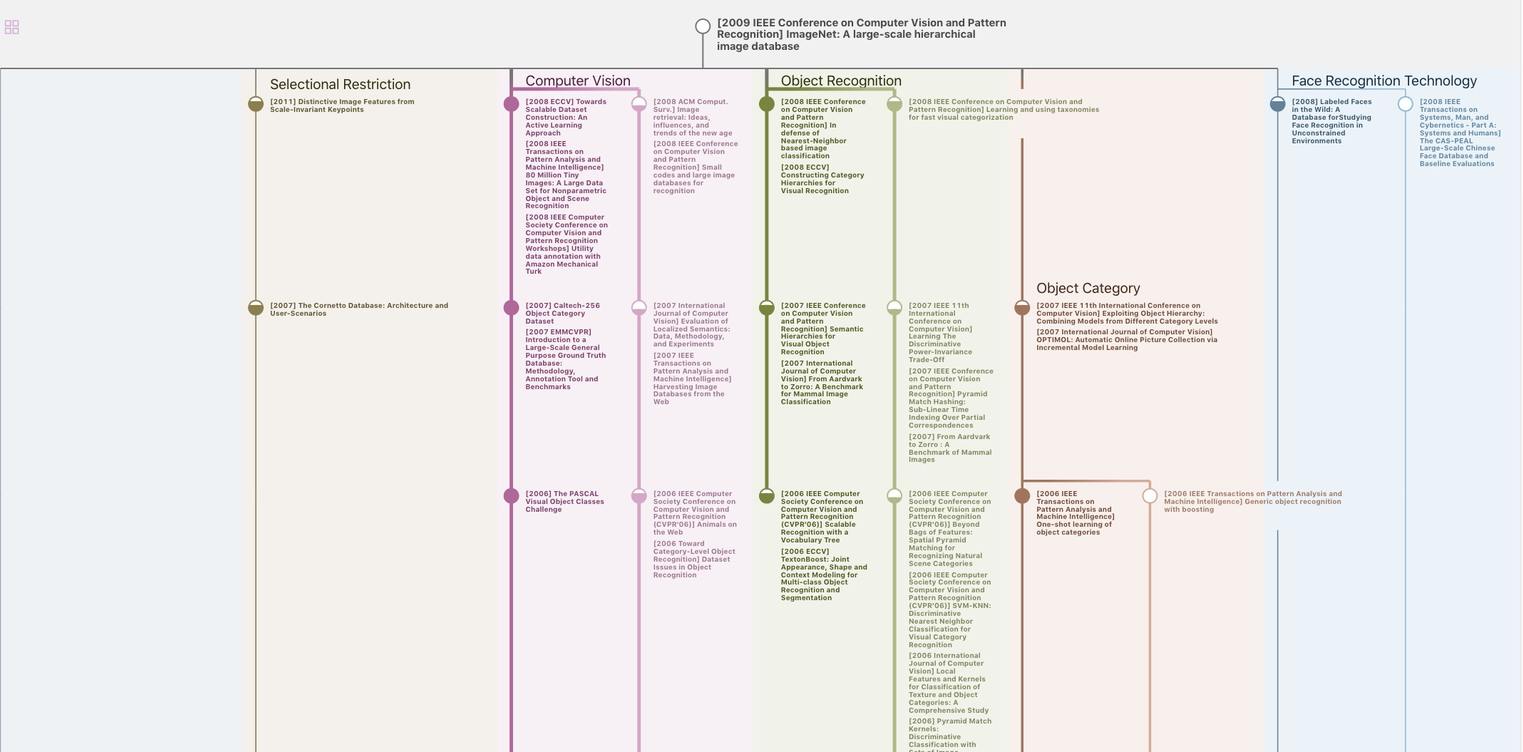
生成溯源树,研究论文发展脉络
Chat Paper
正在生成论文摘要