3D face-model reconstruction from a single image: A feature aggregation approach using hierarchical transformer with weak supervision
Neural Networks(2022)
摘要
Convolutional Neural Networks (CNN) have gained popularity as the de-facto model for any computer vision task. However, CNN have drawbacks, i.e. they fail to extract long-range perceptions in images. Due to their ability to capture long-range dependencies, transformer networks are adopted in computer vision applications, where they show state-of-the-art (SOTA) results in popular tasks like image classification, instance segmentation, and object detection. Although they gained ample attention, transformers have not been applied to 3D face reconstruction tasks. In this work, we propose a novel hierarchical transformer model, added to a feature pyramid aggregation structure, to extract the 3D face parameters from a single 2D image. More specifically, we use pre-trained Swin Transformer backbone networks in a hierarchical manner and add the feature fusion module to aggregate the features in multiple stages. We use a semi-supervised training approach and train our model in a supervised way with the 3DMM parameters from a publicly available dataset and unsupervised training with a differential renderer on other parameters like facial keypoints and facial features. We also train our network on a hybrid unsupervised loss and compare the results with other SOTA approaches. When evaluated across two public datasets on face reconstruction and dense 3D face alignment tasks, our method can achieve comparable results to the current SOTA performance and in some instances do better than the SOTA methods. A detailed subjective evaluation also shows that our method performs better than the previous works in realism and occlusion resistance.
更多查看译文
关键词
Face reconstruction,Hierarchical transformer,Feature fusion,ViT,Swin Transformer
AI 理解论文
溯源树
样例
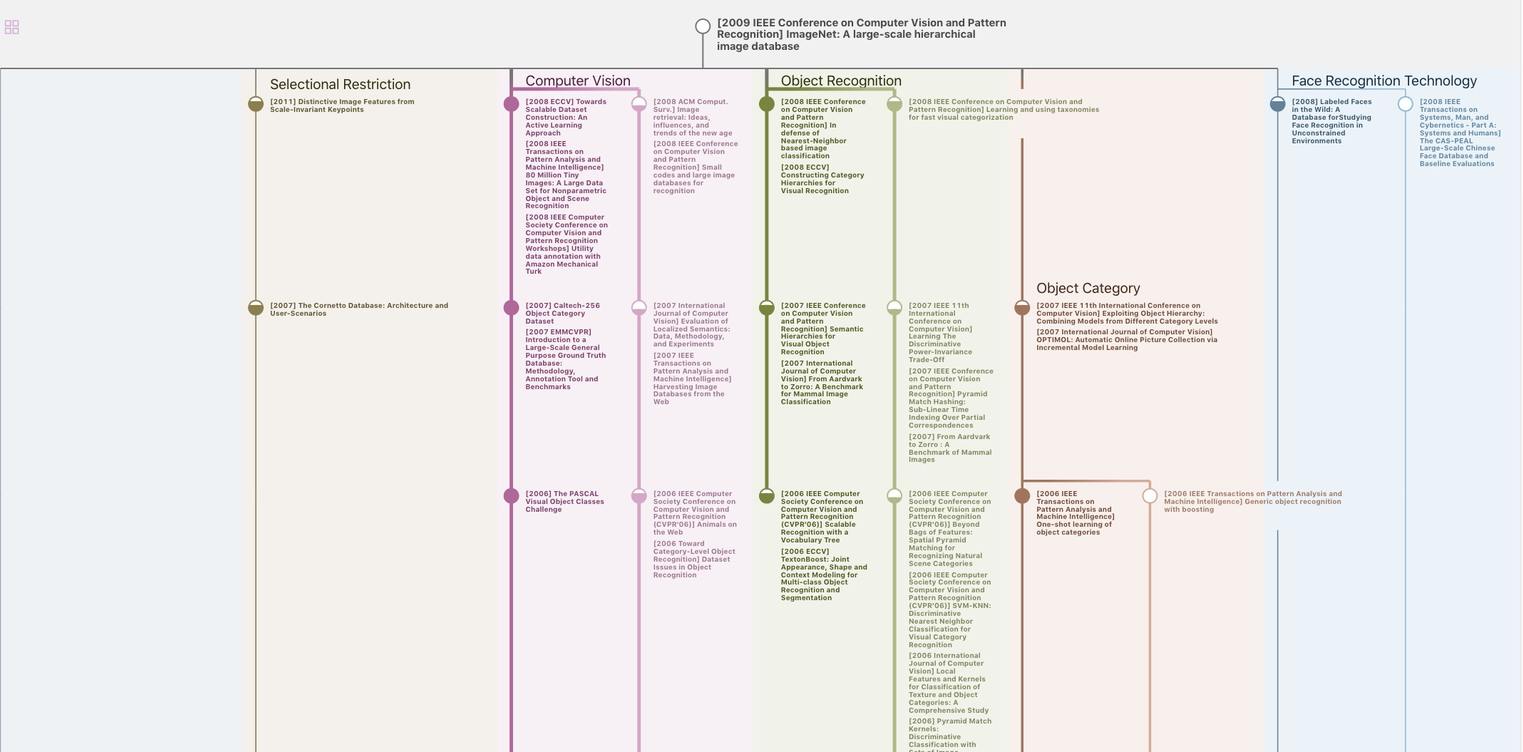
生成溯源树,研究论文发展脉络
Chat Paper
正在生成论文摘要