Ranking-based Federated POI Recommendation with Geographic Effect
IEEE International Joint Conference on Neural Network (IJCNN)(2022)
Abstract
Point of Interest (POI) recommendation system recommends places in which users have never been to but may be interested. Traditionally, it centrally collects contextual information and interaction data to model users' preferences, which raises many privacy concerns. The current studies habitually sacrifice the recommendation performance to cope with privacy concerns. To protect users' privacy while ensuring the performance of the POI recommendation system, we propose a Ranking-based Federated POI Recommendation with Geographic Effect (RFPG). The RFPG allows users to reserve their private data on local devices to secure privacy. It adaptively constructs an active region to model the geographic effect, enhancing users' personalized preference modeling. In addition, we design a probability-based negative sampling method to protect privacy further and improve recommendation performance. This method calculates the probability of a POI being a negative sample through POI geographic distribution, then combines the positive samples to construct a local triplet training dataset. Theoretical analysis and experiments on two real datasets demonstrate that our proposed RFPG improves the performance of the POI recommendation while protecting users' private data.
MoreTranslated text
Key words
Federated Learning,Ranking Learning,POI recommendation
AI Read Science
Must-Reading Tree
Example
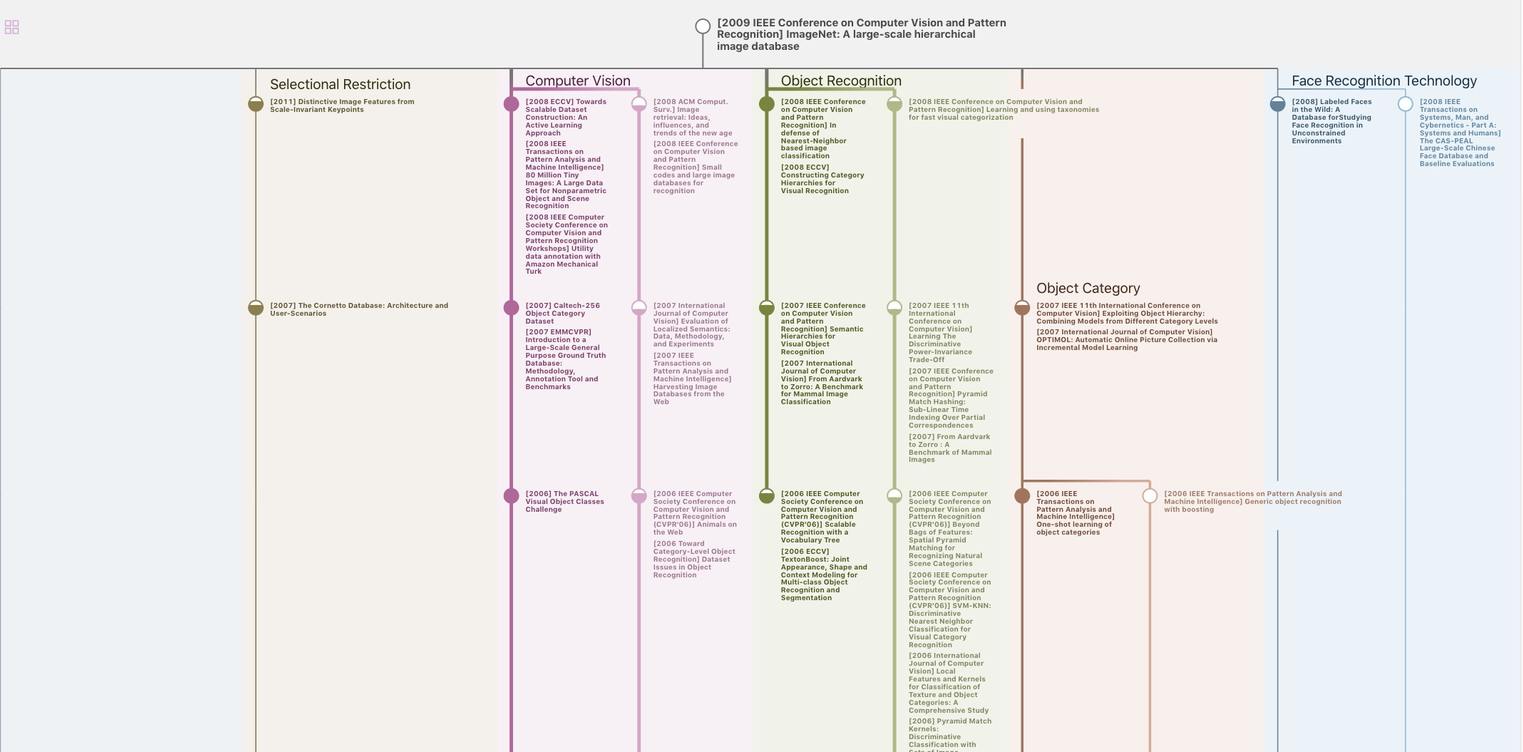
Generate MRT to find the research sequence of this paper
Chat Paper
Summary is being generated by the instructions you defined