Dual Cost-sensitive Graph Convolutional Network
IEEE International Joint Conference on Neural Network (IJCNN)(2022)
摘要
In graph node classification tasks, traditional graph neural network (GNN) models assume that different types of misclassification have equal loss and thus seek to maximize the posterior probability of sample nodes under labeled classes. However, the graph data in realistic scenarios tend to follow unbalanced long-tail class distributions, making it difficult for GNN to accurately represent the minority class samples because of the overfitting tendency to the majority class features. To address this problem, in this paper we propose a novel GNN model, named Dual Cost-sensitive Graph Convolutional Network (DCSGCN). The DCSGCN is a two-tower model containing two sub-networks that compute the posterior probability and the mis-classification cost separately. It uses the cost as complementary information in classification to correct the posterior probability under the minimal risk perspective. Furthermore, we propose a series of new methods to compute node cost labels based on graph topological information and node class distribution. Extensive experiments lead to the observation that DCSGCN outperforms the state-of-the-art model on diverse real-world imbalanced graphs.
更多查看译文
关键词
Graph neural networks,cost-sensitive learning,imbalanced classification
AI 理解论文
溯源树
样例
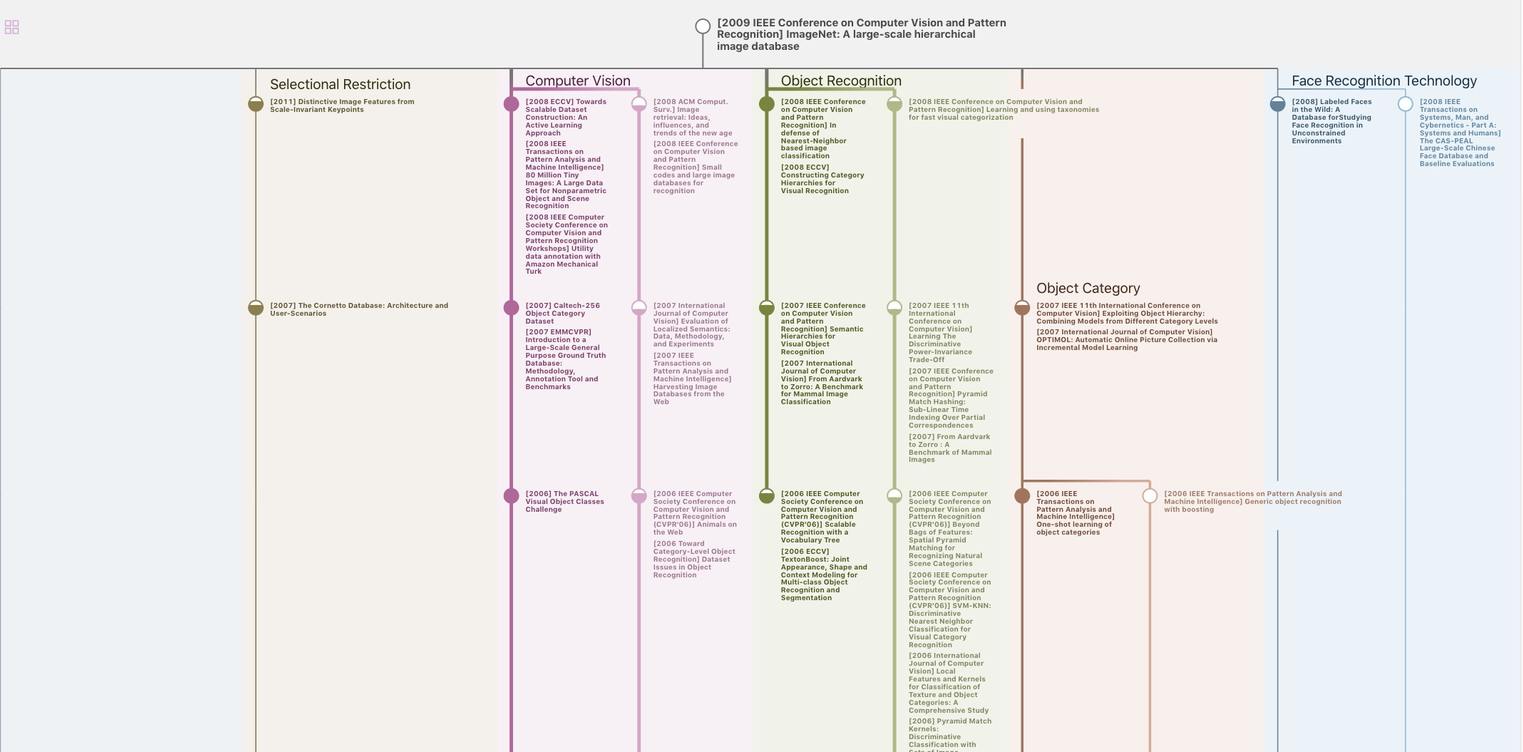
生成溯源树,研究论文发展脉络
Chat Paper
正在生成论文摘要