Domain generalization of deep networks for medical image segmentation via meta learning
Meta-Learning with Medical Imaging and Health Informatics Applications(2023)
摘要
The generalization capacity of deep models is crucial for real-world clinical deployment, where domain shifts usually exist due to changes of image acquisition conditions. This chapter presents domain generalization methods for medical image segmentation with meta learning technique, aiming to generalize the deep models learned from multiple source domains to unseen target domains. We present solutions under two realistic scenarios, i.e., centralized training and decentralized federated training. For the first scenario, we establish meta learning with multidomain data to construct virtual training and testing domains and enhance the model optimization towards simulated domain shifts with two shape-aware metaobjectives. Under the second scenario, a frequency-space interpolation mechanism is devised to enable each client access the multisource distributions under the constraint of data decentralization. The meta learning scheme is then established locally with explicit feature regularization imposed around the ambiguous regions to promote generalizable model parameters for segmentation problems. Experimental results on two medical image segmentation tasks have validated the effectiveness of our methods on the generalization problems under the two realistic training scenarios.
更多查看译文
关键词
medical image segmentation,meta learning,domain generalization,deep networks
AI 理解论文
溯源树
样例
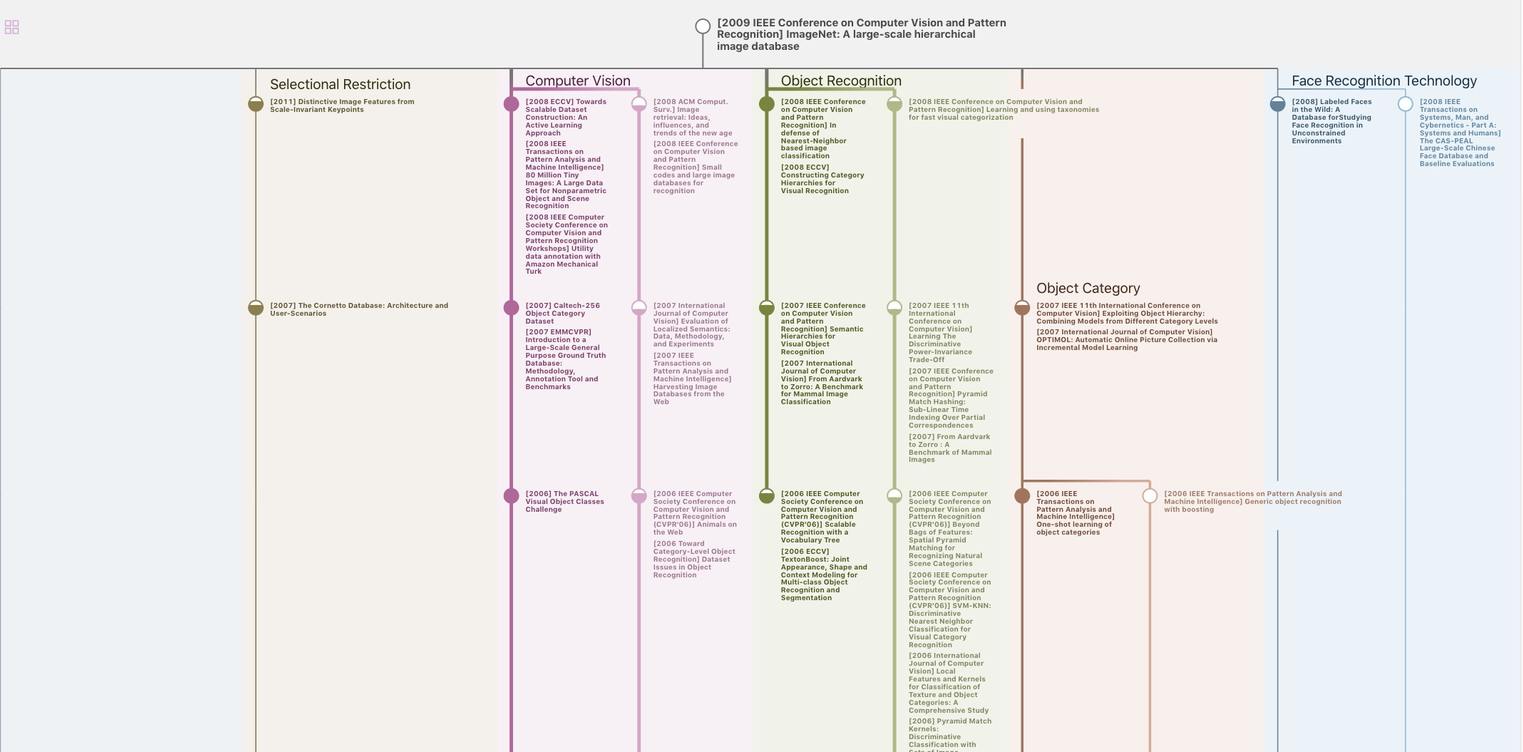
生成溯源树,研究论文发展脉络
Chat Paper
正在生成论文摘要