Multi-Virtual-Agent Reinforcement Learning for a Stochastic Predator-Prey Grid Environment
IEEE International Joint Conference on Neural Network (IJCNN)(2022)
Abstract
Generalization problem of reinforcement learning is crucial especially for dynamic environments. Conventional reinforcement learning methods solve the problems with some ideal assumptions and are difficult to be applied in dynamic environments directly. In this paper, we propose a new multivirtual-agent reinforcement learning (MVARL) approach for a predator-prey grid game. The designed method can find the optimal solution even when the predator moves. Specifically, we design virtual agents to interact with simulated changing environments in parallel instead of using actual agents. Moreover, a global agent learns information from these virtual agents and interacts with the actual environment at the same time. This method can not only effectively improve the generalization performance of reinforcement learning in dynamic environments, but also reduce the overall computational cost. Two simulation studies are considered in this paper to validate the effectiveness of the designed method. We also compare the results with the conventional reinforcement learning methods. The results indicate that our proposed method can improve the robustness of reinforcement learning method and contribute to the generalization to certain extent.
MoreTranslated text
Key words
Reinforcement learning,multiple virtual agents,generalization problem,dynamic environment,and parallel learning
AI Read Science
Must-Reading Tree
Example
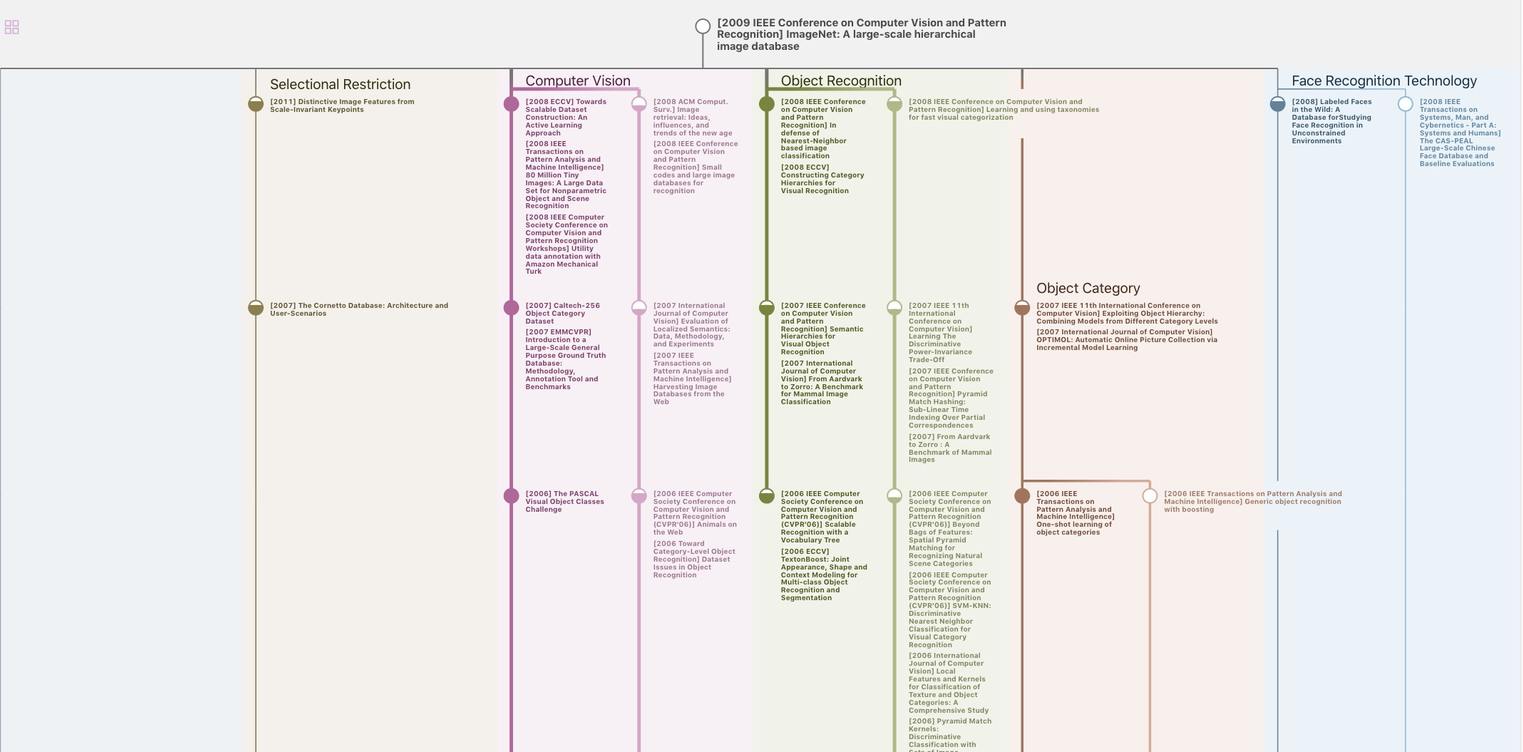
Generate MRT to find the research sequence of this paper
Chat Paper
Summary is being generated by the instructions you defined