A Hybrid Feature Reduction Approach for Medical Decision Support System
Mathematical Problems in Engineering(2022)
Abstract
Feature reduction is essential at the preprocessing stage of designing any reliable and fast disease diagnosis model. Addressing the limitations like disease specificity, information loss, and operating NP problem in polynomial time, this paper introduces a two-step hybrid feature selection approach to identify a subset of most relevant and contributing features of each medical dataset for constructing diagnostic model. The concept of information gain is used in Step I to select the informative features, whereas a correlation coefficient-based approach is employed in Step II to retain the informative features possessing much dependency with class attribute but less dependency among the non-class attributes. In particular, both the approaches are sequentially fused to select approximately optimal features in order to construct better classification model in terms of performance and time. The optimal threshold criteria are decided to choose the most appropriate features from the datasets. The effectiveness of the proposed approach is assessed using six individual competent learners and one ensemble learner over seventeen disease datasets of smaller to larger dimensions. The empirical results indicate that the proposed approach improves the performance over the datasets after feature selection, reducing considerable amount of irrelevant and redundant data.
MoreTranslated text
Key words
hybrid feature reduction approach,decision,support
AI Read Science
Must-Reading Tree
Example
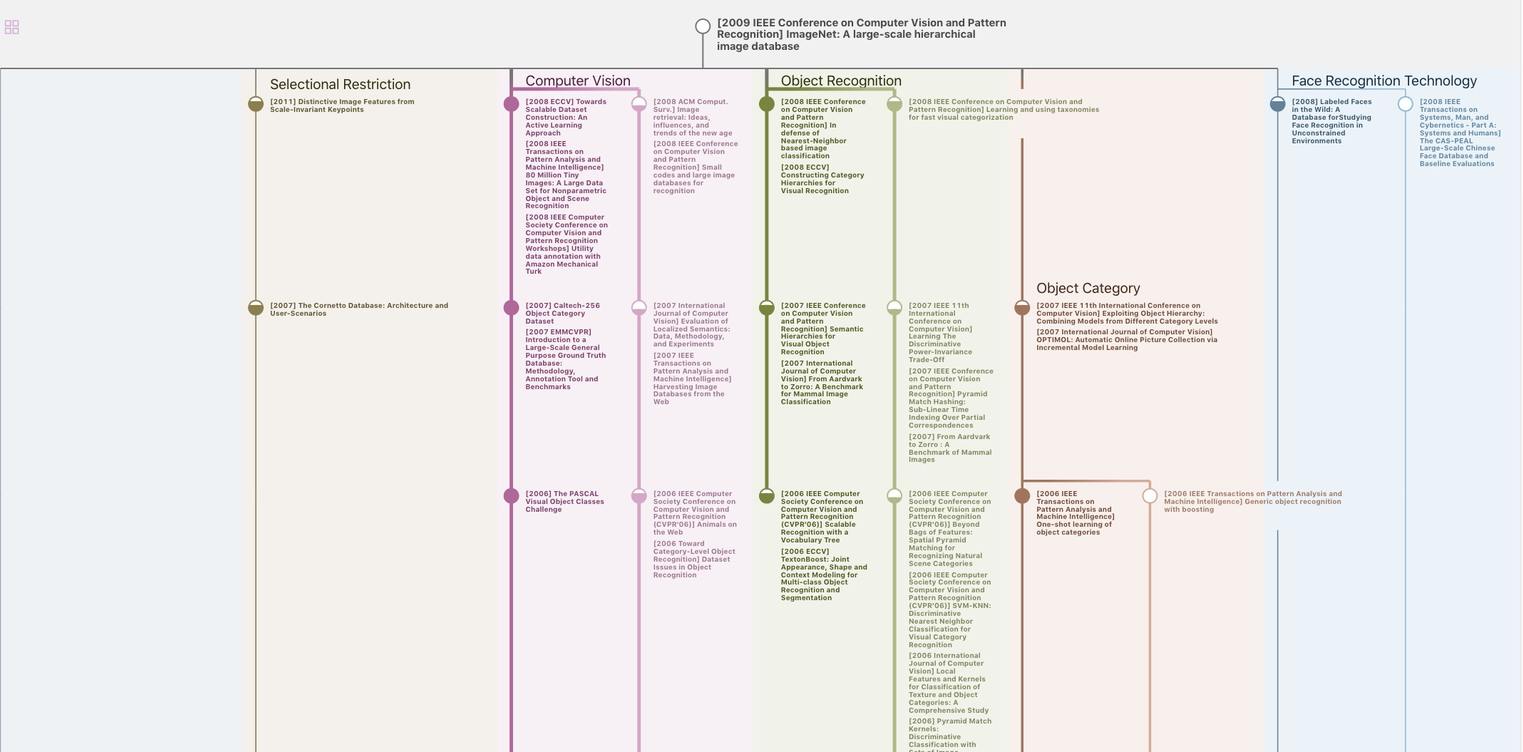
Generate MRT to find the research sequence of this paper
Chat Paper
Summary is being generated by the instructions you defined