Auto-Encoding Independent Attribute Transformation for Naive Bayesian Classifier
IEEE International Joint Conference on Neural Network (IJCNN)(2022)
摘要
To alleviate the attribute independence assumption, this paper proposes an auto-encoding naive Bayesian classifier (AE-NBC) which is trained based on transformed independent condition-attributes using an auto-encoding strategy. First, an auto-encoding neural network (AENN) with single hidden-layer is created to map the original data set to a high-dimensional data space. To guarantee the independence among auto-encoding condition-attributes (AECAs), an efficient and convergent objective function is elaborated to optimize the input-layer and outputlayer weights of the AENN by considering both the auto-encoding error and attribute independence degree. Second, independent component analysis is iteratively carried out to remove the redundant attributes in AECAs so that the optimal transformed condition-attributes (TCAs) are obtained for the training of the NBC. Experiments were conducted on 29 benchmark data sets to validate the rationality, feasibility, and effectiveness of AE-NBC. Experimental results show that the independence among TCAs is stronger than among the original condition-attributes, and that AE-NBC obtain higher training and testing accuracies than six Bayesian classifiers.
更多查看译文
关键词
naive Bayesian classifier,attribute independence assumption,auto-encoding neural network,probability density function,Bayesian network
AI 理解论文
溯源树
样例
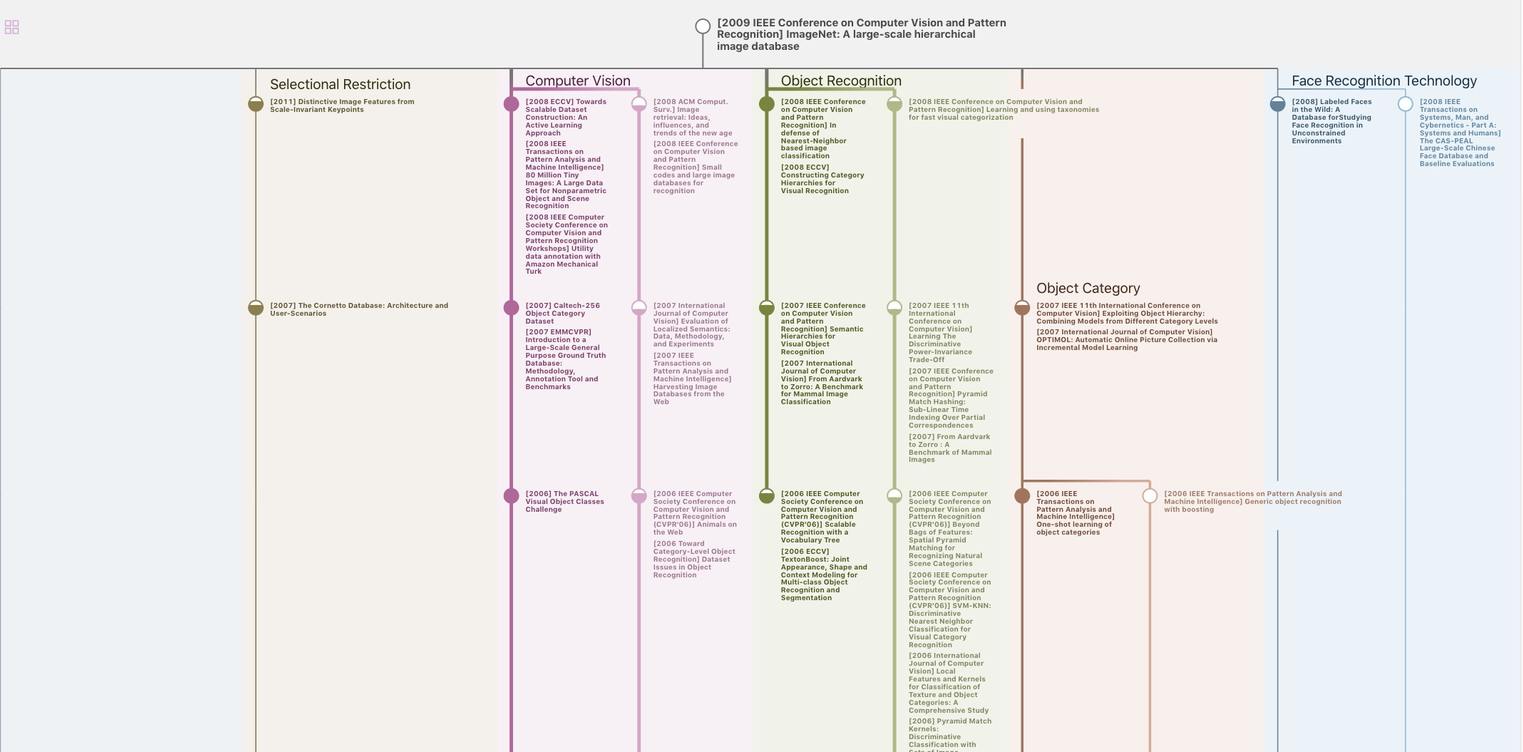
生成溯源树,研究论文发展脉络
Chat Paper
正在生成论文摘要