FedNCF: Federated Neural Collaborative Filtering for Privacy-preserving Recommender System
IEEE International Joint Conference on Neural Network (IJCNN)(2022)
摘要
Recommender systems are collecting user data to provide better personalized services. However, centralized collection and analysis of users' private data will raise privacy concerns and legal risks. The emergence of federated learning enables training a machine learning model from highly decentralized data. This paper extends the Neural Collaborative Filtering (NCF) method using a federated setting and proposes a privacy-preserving federated recommender system named FedNCF, which can train NCF models without needing to know user's private data. We apply differential privacy to the computed gradients to prevent inference attacks. To improve the utility of differential privacy, we propose an adaptive differential privacy approach that meticulously adjusts the noise scale in each iteration controlled by a decay rate. Our proposed approach provides an explicit mathematical expression to estimate the user's privacy loss by truncated Concentrated Differential Privacy (tCDP). Extensive experiments and analysis demonstrate that FedNCF can achieve competitive performance with the centralized NCF meanwhile effectively protect users' privacy.
更多查看译文
关键词
neural collaborative filtering,federated learning,differential privacy,privacy-preserving
AI 理解论文
溯源树
样例
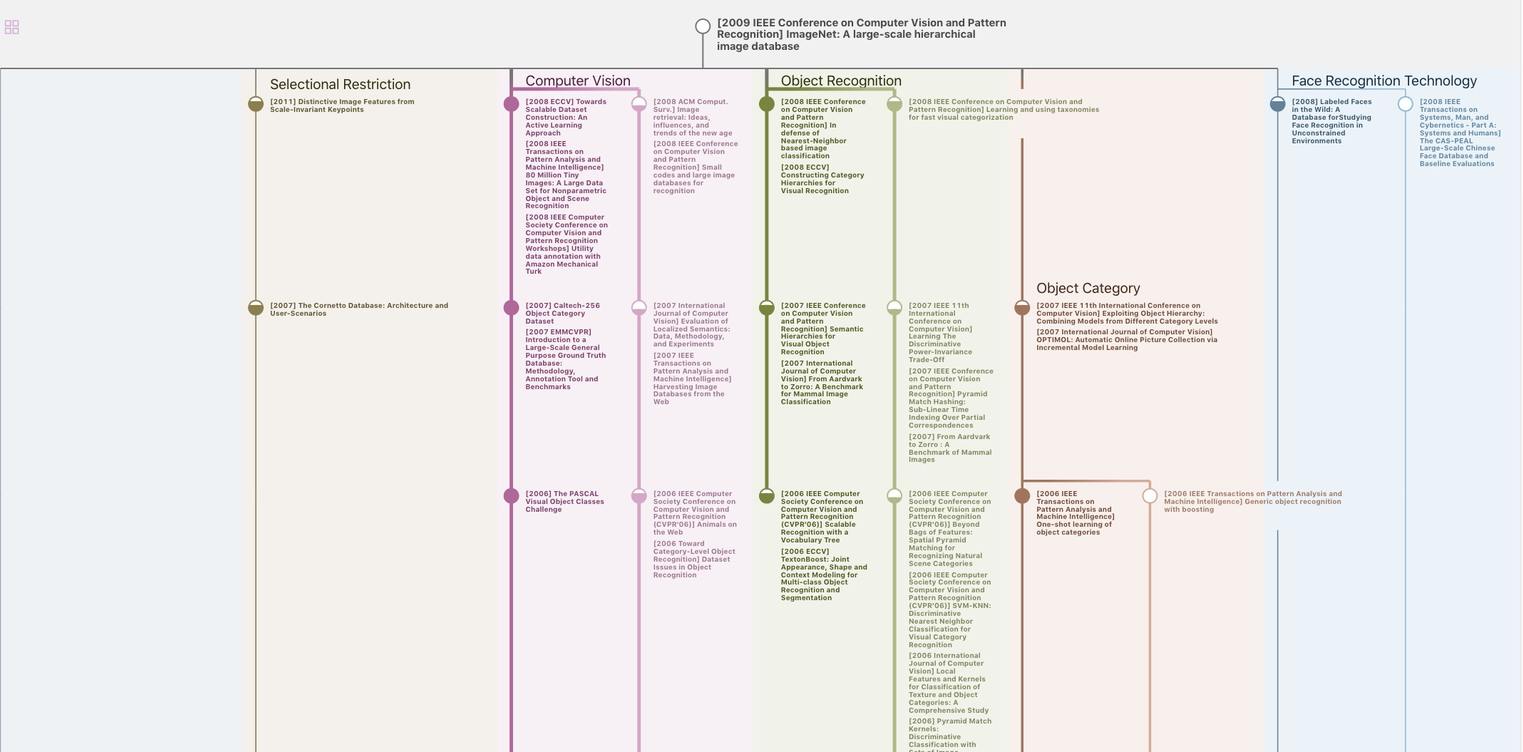
生成溯源树,研究论文发展脉络
Chat Paper
正在生成论文摘要