Adaptive Robust Least-Squares Smoothing Algorithm
IEEE TRANSACTIONS ON INSTRUMENTATION AND MEASUREMENT(2022)
摘要
The Kalman smoothing algorithm is widely used in offline data processing in global navigation satellites system (GNSS) to improve filter calculation accuracy. When there are measurement outliers or biased random models, the adaptive Kalman smoothing algorithm can reduce their impact on the smoothing results to a certain extent. Nevertheless, the adaptive Kalman smoothing algorithm usually has difficulty obtaining optimal estimation in a complex observation environment due to the inherent defects of the algorithm itself. Therefore, we propose an adaptive robust least-squares smoothing algorithm. The row and column transformation of the least-square smooth noise covariance matrix is realized by constructing an orthogonal space transformation matrix, and the noise covariance estimation problem of the Kalman filter is transformed into the problem of solving the variance-covariance component elements under a specific matrix structure. In addition, by selecting the observation value with the largest standardized residual to determine whether it is a gross error, we avoid the gross error misjudgment problem caused by the two-factor robust estimation method under least-square smoothing and further improve the smoothing accuracy. Finally, two experiments are studied to compare the performance of the new algorithm and the state-of-the-art algorithms. The proposed algorithm has demonstrated better performance in terms of robustness and estimation accuracy.
更多查看译文
关键词
Smoothing methods,Estimation,Kalman filters,Mathematical models,Noise measurement,State estimation,Resists,Least squares,noise covariance estimation,robust estimation,smoothing
AI 理解论文
溯源树
样例
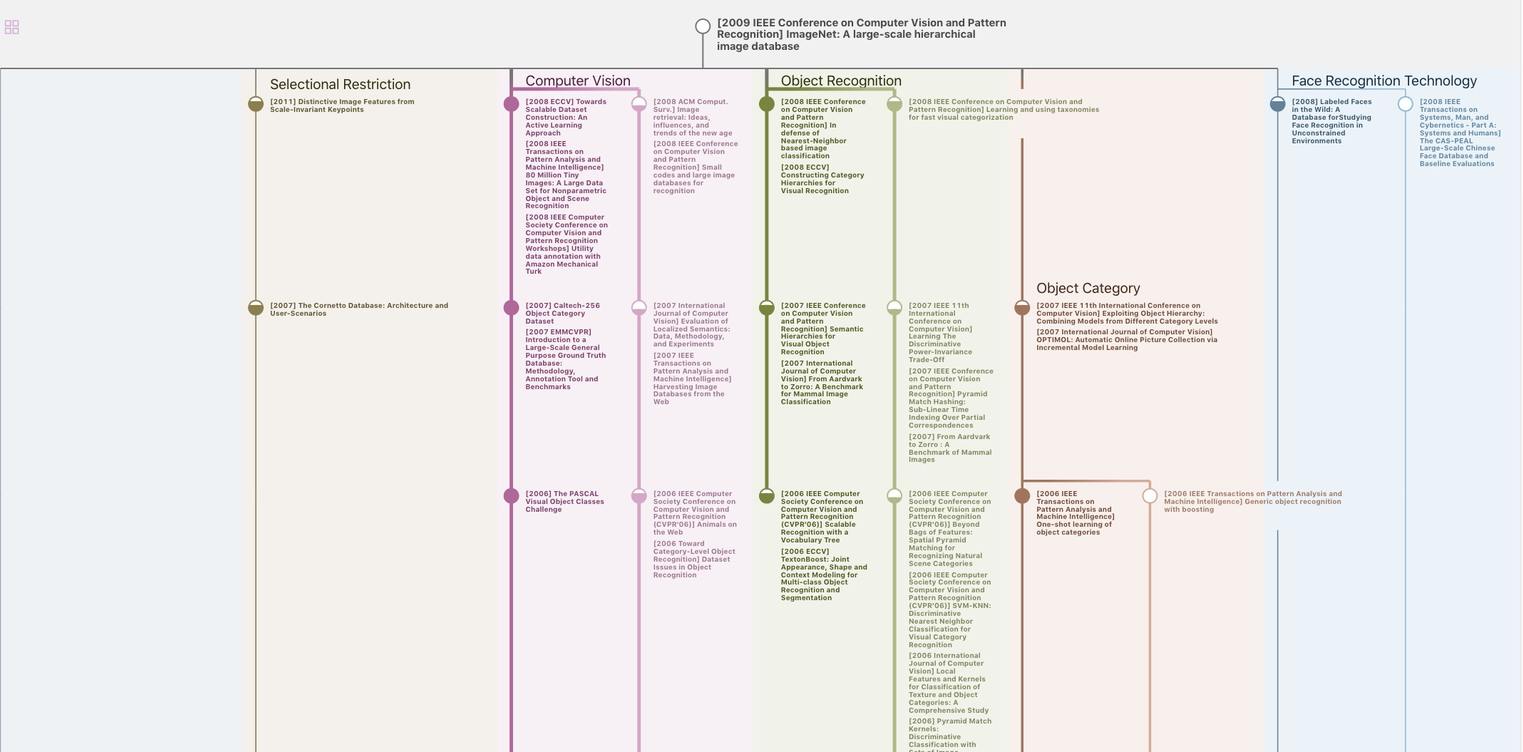
生成溯源树,研究论文发展脉络
Chat Paper
正在生成论文摘要