Unsupervised Domain Adaptation via Deep Conditional Adaptation Network
Pattern Recognition(2023)
摘要
Unsupervised domain adaptation (UDA) aims to generalize the supervised model trained on a source domain to an unlabeled target domain. Previous works mainly rely on the marginal distribution alignment of feature spaces, which ignore the conditional dependence between features and labels, and may suffer from negative transfer. To address this problem, some UDA methods focus on aligning the conditional distributions of feature spaces. However, most of these methods rely on class-specific Maximum Mean Discrepancy or adversarial training, which may suffer from mode collapse and training instability. In this paper, we propose a Deep Conditional Adaptation Network (DCAN) that aligns the conditional distributions by minimizing Conditional Maximum Mean Discrepancy, and extracts discriminant information from the target domain by maximizing the mutual information between samples and the prediction labels. Conditional Maximum Mean Discrepancy measures the difference between conditional distributions directly through their conditional embedding in Reproducing Kernel Hilbert Space, thus DCAN can be trained stably and converge fast. Mutual information can be expressed as the difference between the entropy and conditional entropy of the predicted category variable, thus DCAN can extract the discriminant information of individual and overall distributions in the target domain, simultaneously. In addition, DCAN can be used to address a special scenario, Partial UDA, where the target domain category is a subset of the source domain category. Experiments on both UDA and Partial UDA show that DCAN achieves superior classification performance over state-of-the-art methods.(c) 2022 Elsevier Ltd. All rights reserved.
更多查看译文
关键词
Deep learning,Domain adaptation,Feature extraction,Conditional maximum mean discrepancy,Kernel method
AI 理解论文
溯源树
样例
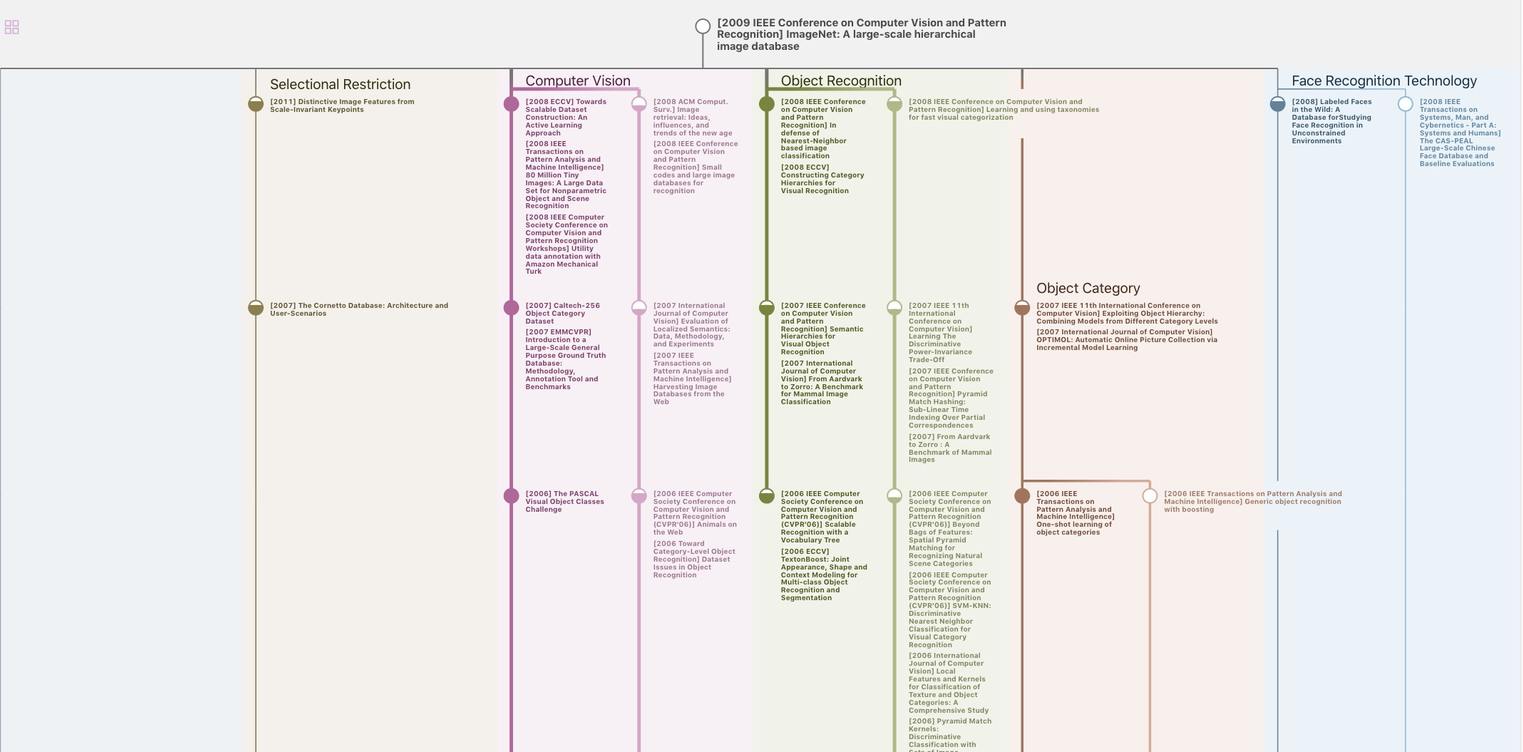
生成溯源树,研究论文发展脉络
Chat Paper
正在生成论文摘要