Grouped Spherical Data Modeling Through Hierarchical Nonparametric Bayesian Models and Its Application to fMRI Data Analysis.
IEEE transactions on neural networks and learning systems(2024)
摘要
Recently, spherical data (i.e., L2 normalized vectors) modeling has become a promising research topic in various real-world applications (such as gene expression data analysis, document categorization, and gesture recognition). In this work, we propose a hierarchical nonparametric Bayesian model based on von Mises-Fisher (VMF) distributions for modeling spherical data that involve multiple groups, where each observation within a group is sampled from a VMF mixture model with an infinite number of components allowing them to be shared across groups. Our model is formulated by employing a hierarchical nonparametric Bayesian framework known as the hierarchical Pitman-Yor (HPY) process mixture model, which possesses a power-law nature over the distribution of the components and is particularly useful for data distributions with heavy tails and skewness. To learn the proposed HPY process mixture model with VMF distributions, we systematically develop a closed-form optimization algorithm based on variational Bayes (VB). The merits of the proposed hierarchical Bayesian nonparametric model for modeling grouped spherical data are demonstrated through experiments on both synthetic data and a real-world application about resting-state functional magnetic resonance imaging (fMRI) data analysis.
更多查看译文
AI 理解论文
溯源树
样例
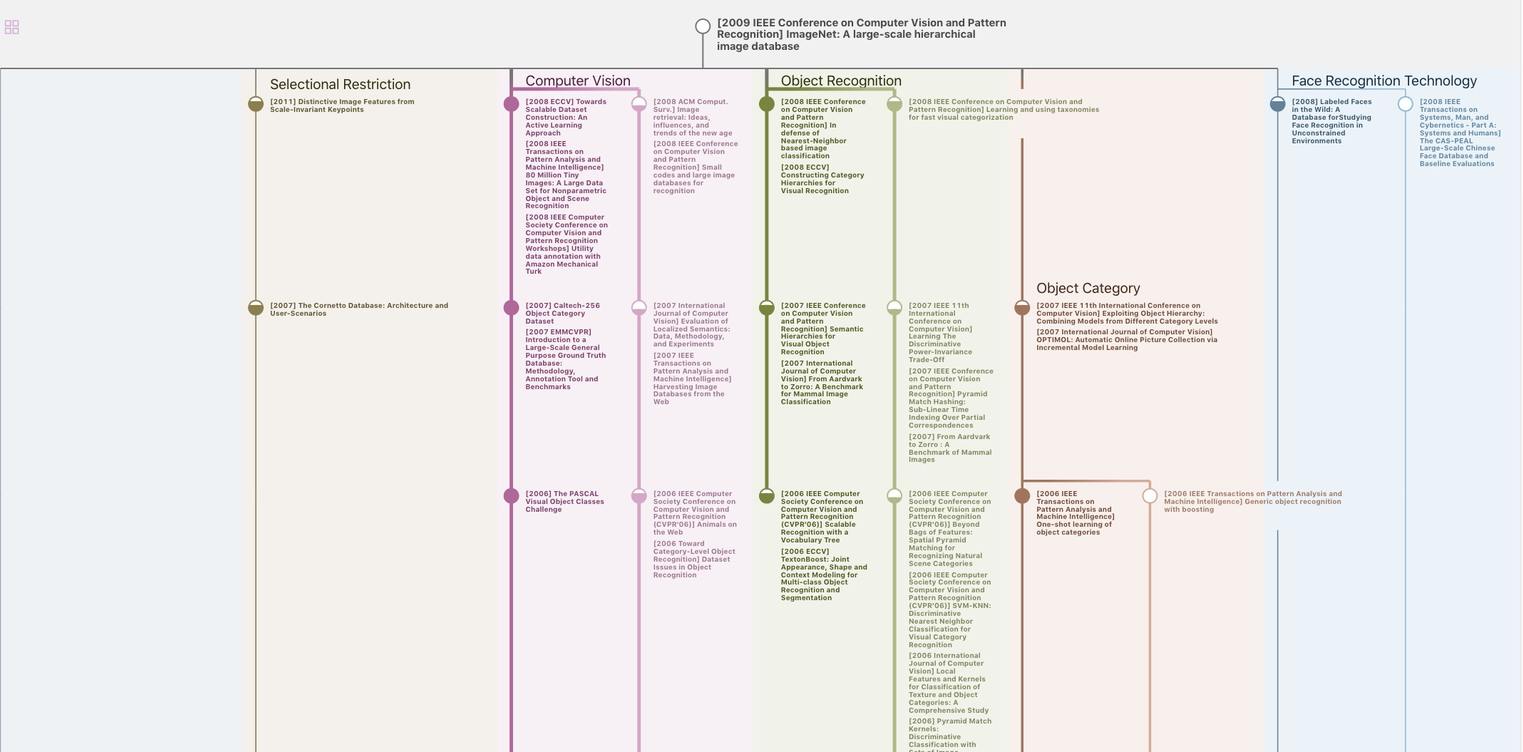
生成溯源树,研究论文发展脉络
Chat Paper
正在生成论文摘要