A novel interpretable machine learning algorithm to identify optimal parameter space for cancer growth
FRONTIERS IN BIG DATA(2022)
摘要
Recent years have seen an increase in the application of machine learning to the analysis of physical and biological systems, including cancer progression. A fundamental downside to these tools is that their complexity and nonlinearity makes it almost impossible to establish a deterministic, a priori relationship between their input and output, and thus their predictions are not wholly accountable. We begin with a series of proofs establishing that this holds even for the simplest possible model of a neural network; the effects of specific loss functions are explored more fully in Appendices. We return to first principles and consider how to construct a physics-inspired model of tumor growth without resorting to stochastic gradient descent or artificial nonlinearities. We derive an algorithm which explores the space of possible parameters in a model of tumor growth and identifies candidate equations much faster than a simulated annealing approach. We test this algorithm on synthetic tumor-growth trajectories and show that it can efficiently and reliably narrow down the area of parameter space where the correct values are located. This approach has the potential to greatly improve the speed and reliability with which patient-specific models of cancer growth can be identified in a clinical setting.
更多查看译文
关键词
cancer, neural networks, white-box machine learning, interpretability, parameter optimization
AI 理解论文
溯源树
样例
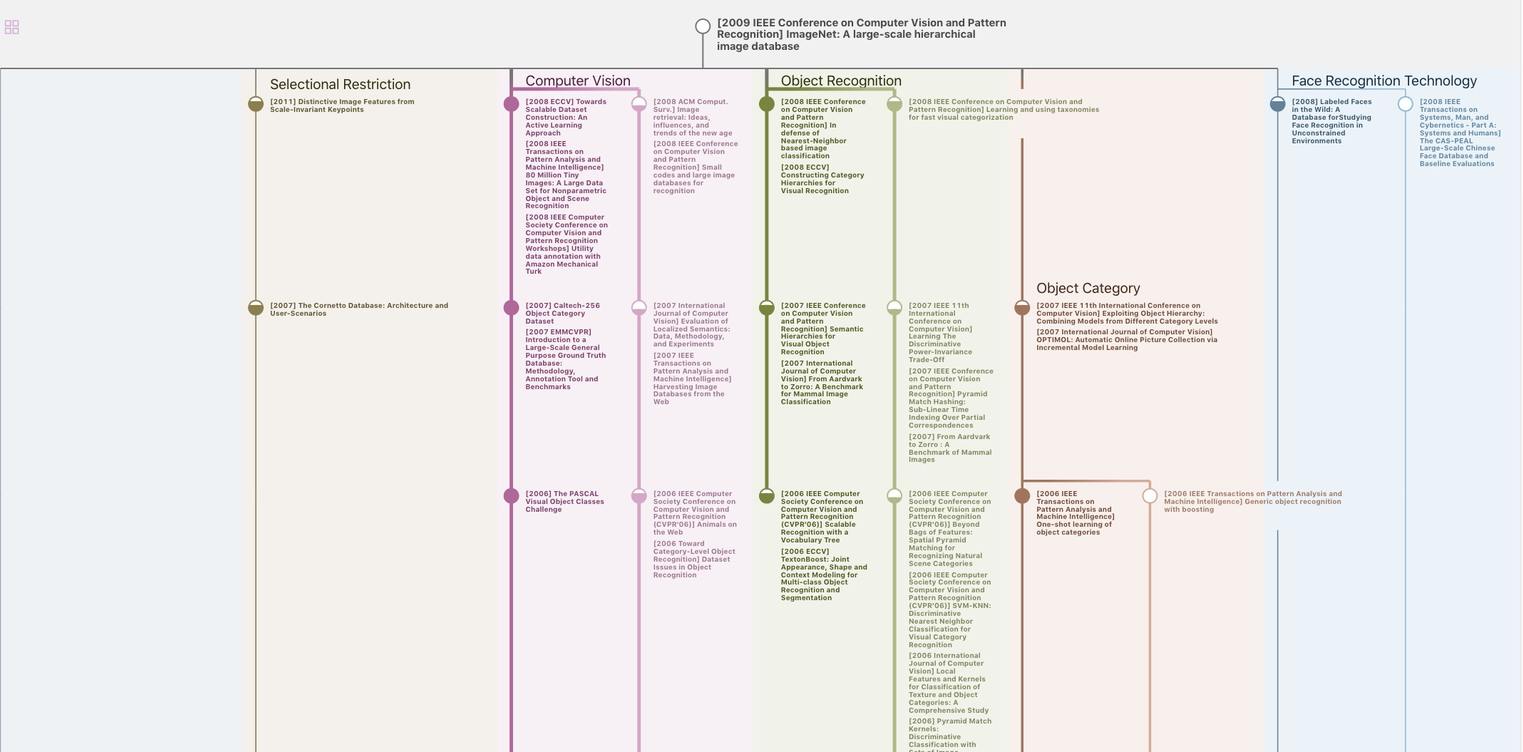
生成溯源树,研究论文发展脉络
Chat Paper
正在生成论文摘要