Segmentation and Tracking of Mammary Epithelial Organoids in Brightfield Microscopy
IEEE Transactions on Medical Imaging(2023)
摘要
We present an automated and deep-learning-based workflow to quantitatively analyze the spatiotemporal development of mammary epithelial organoids in two-dimensional time-lapse (2D+t) sequences acquired using a brightfield microscope at high resolution. It involves a convolutional neural network (U-Net), purposely trained using computer-generated bioimage data created by a conditional generative adversarial network (pix2pixHD), to infer semantic segmentation, adaptive morphological filtering to identify organoid instances, and a shape-similarity-constrained, instance-segmentation-correcting tracking procedure to reliably cherry-pick the organoid instances of interest in time. By validating it using real 2D+t sequences of mouse mammary epithelial organoids of morphologically different phenotypes, we clearly demonstrate that the workflow achieves reliable segmentation and tracking performance, providing a reproducible and laborless alternative to manual analyses of the acquired bioimage data.
更多查看译文
关键词
Organoid segmentation,organoid tracking,brightfield microscopy,deep learning,image synthesis
AI 理解论文
溯源树
样例
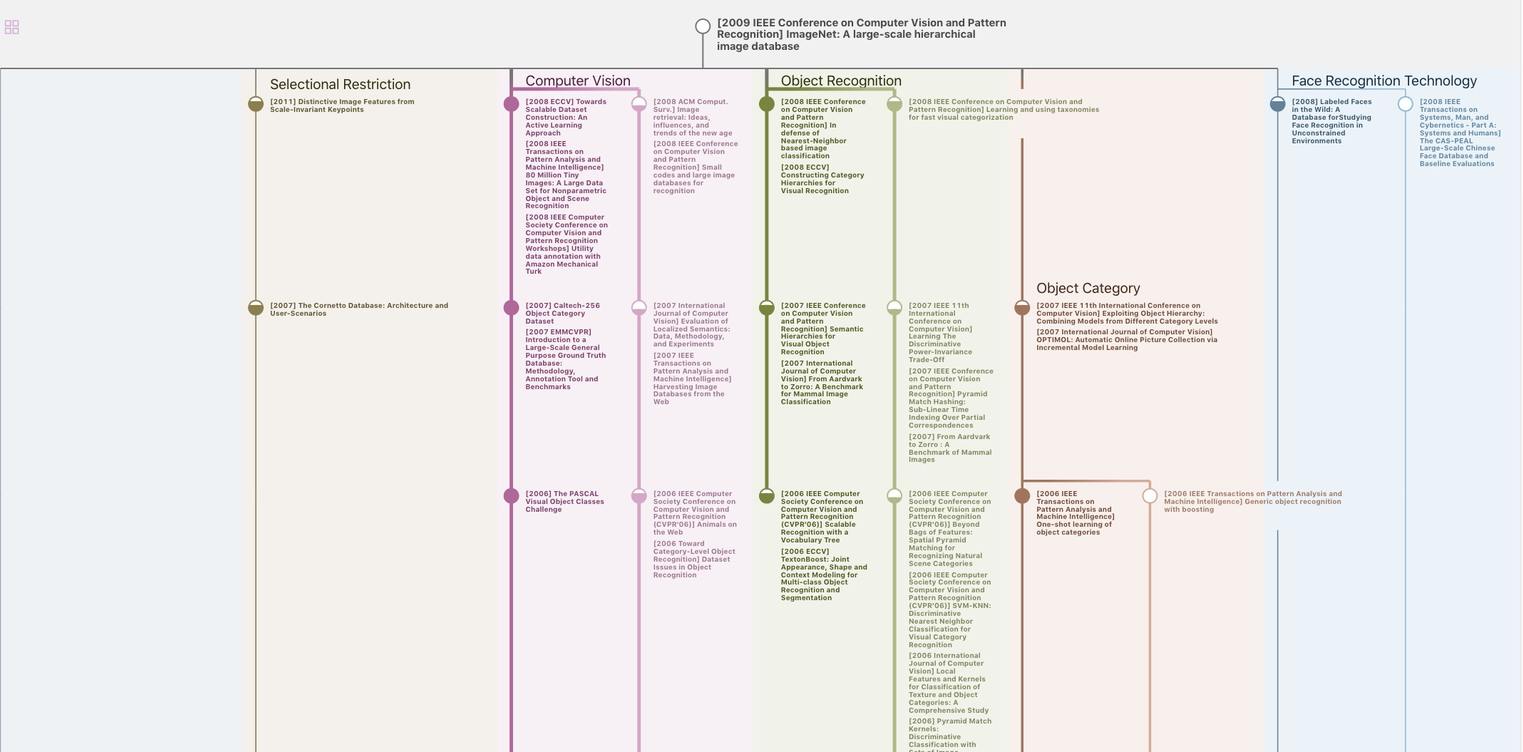
生成溯源树,研究论文发展脉络
Chat Paper
正在生成论文摘要