FSRDD: An Efficient Few-Shot Detector for Rare City Road Damage Detection.
IEEE Trans. Intell. Transp. Syst.(2022)
摘要
Road damage detection (RDD) is indispensable for safe autonomous driving. Existing RDD models focus on designing feature representations following expert knowledge. However, collecting and labeling all types of samples is time-consuming and leads to insufficient training data. To alleviate the adverse effect of few training samples, a novel few-shot road damage detector (FSRDD) is proposed in this paper to detect rare road damages. The proposed FSRDD includes three stages. First, fully annotated abundant base classes are leveraged to train a base detector, where ghost attention (GA) and proposal feature metric (PFM) modules are developed to eliminate the redundant information and measure the proposal features, respectively. Second, the recognition branch of the detector is fine-tuned using a few samples of all classes. Finally, the test set is inferred with the help of an offline scale-aware prototypical calibration block (SPCB). Extensive experiments show that our FSRDD achieves 10-shot rare road damage detection with 33.4% and 12.9% mAP50 on RDD and CNRDD datasets, respectively, significantly outperforming state-of-the-art methods.
更多查看译文
关键词
Roads,Detectors,Feature extraction,Prototypes,Proposals,Training,Measurement,Road damage,deep learning,few-shot detection,fine-tuning
AI 理解论文
溯源树
样例
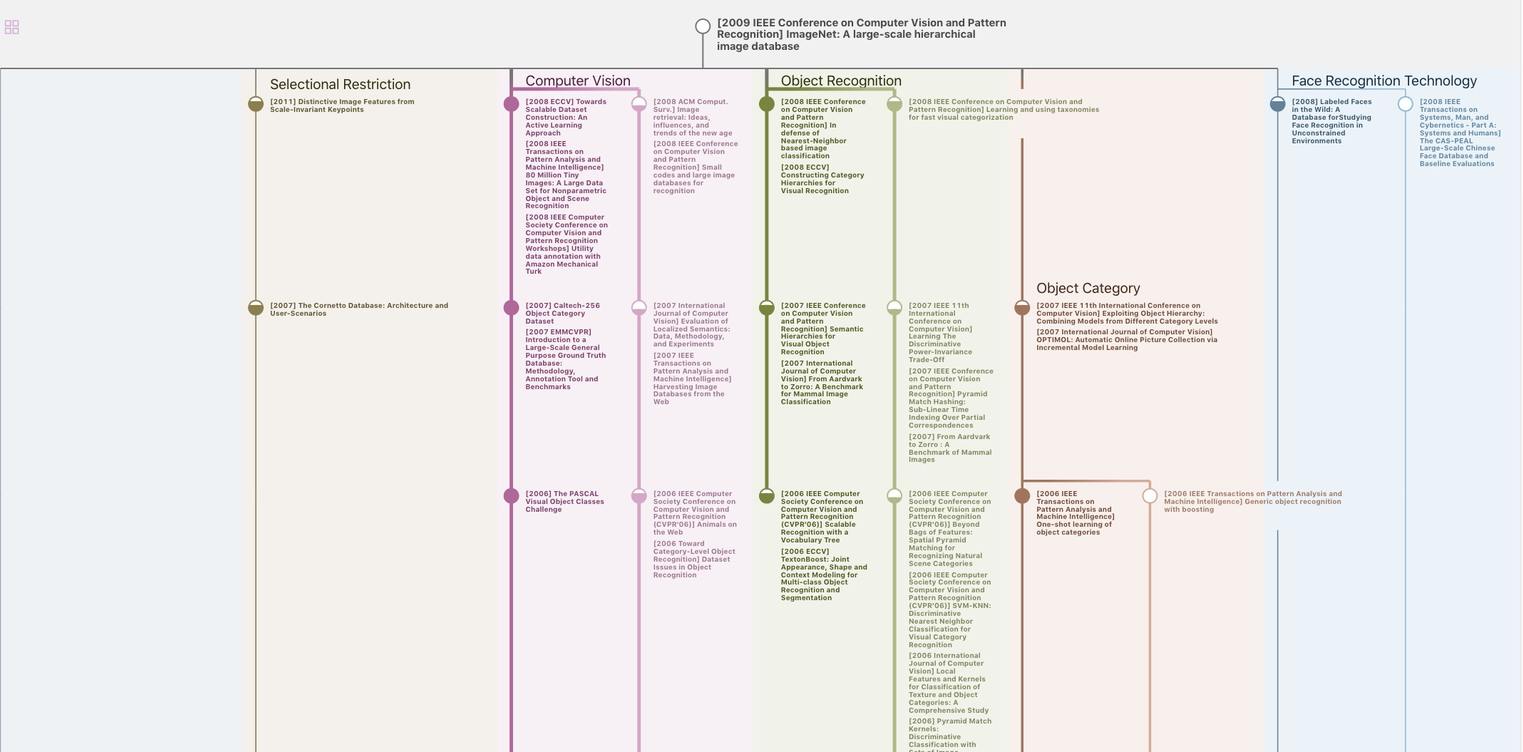
生成溯源树,研究论文发展脉络
Chat Paper
正在生成论文摘要