Fetal Ultrasound Standard Plane Detection With Coarse-to-Fine Multi-Task Learning
IEEE journal of biomedical and health informatics(2023)
摘要
The ultrasound standard plane plays an important role in prenatal fetal growth parameter measurement and disease diagnosis in prenatal screening. However, obtaining standard planes in a fetal ultrasound video is not only laborious and time-consuming but also depends on the clinical experience of sonographers to a certain extent. To improve the acquisition efficiency and accuracy of the ultrasound standard plane, we propose a novel detection framework that utilizes both the coarse-to-fine detection strategy and multi-task learning mechanism for feature-fused images. First, traditional manually-designed features and deep learning-based features are fused to obtain low-level shared features, which can enhance the model's feature expression ability. Inspired by the process of human recognition, ultrasound standard plane detection is divided into a coarse process of plane type classification and a fine process of standard-or-not detection, which is implemented via an end-to-end multi-task learning network. The region-of-interest area is also recognised in our detection framework to suppress the influence of a variable maternal background. Extensive experiments are conducted on three ultrasound planes of the first-class fetal examination, i.e., the femur, thalamus, and abdomen ultrasound images. The experiment results show that our method outperforms competing methods in terms of accuracy, which demonstrates the efficacy of the proposed method and can reduce the workload of sonographers in prenatal screening.
更多查看译文
关键词
ultrasound,learning,detection,coarse-to-fine,multi-task
AI 理解论文
溯源树
样例
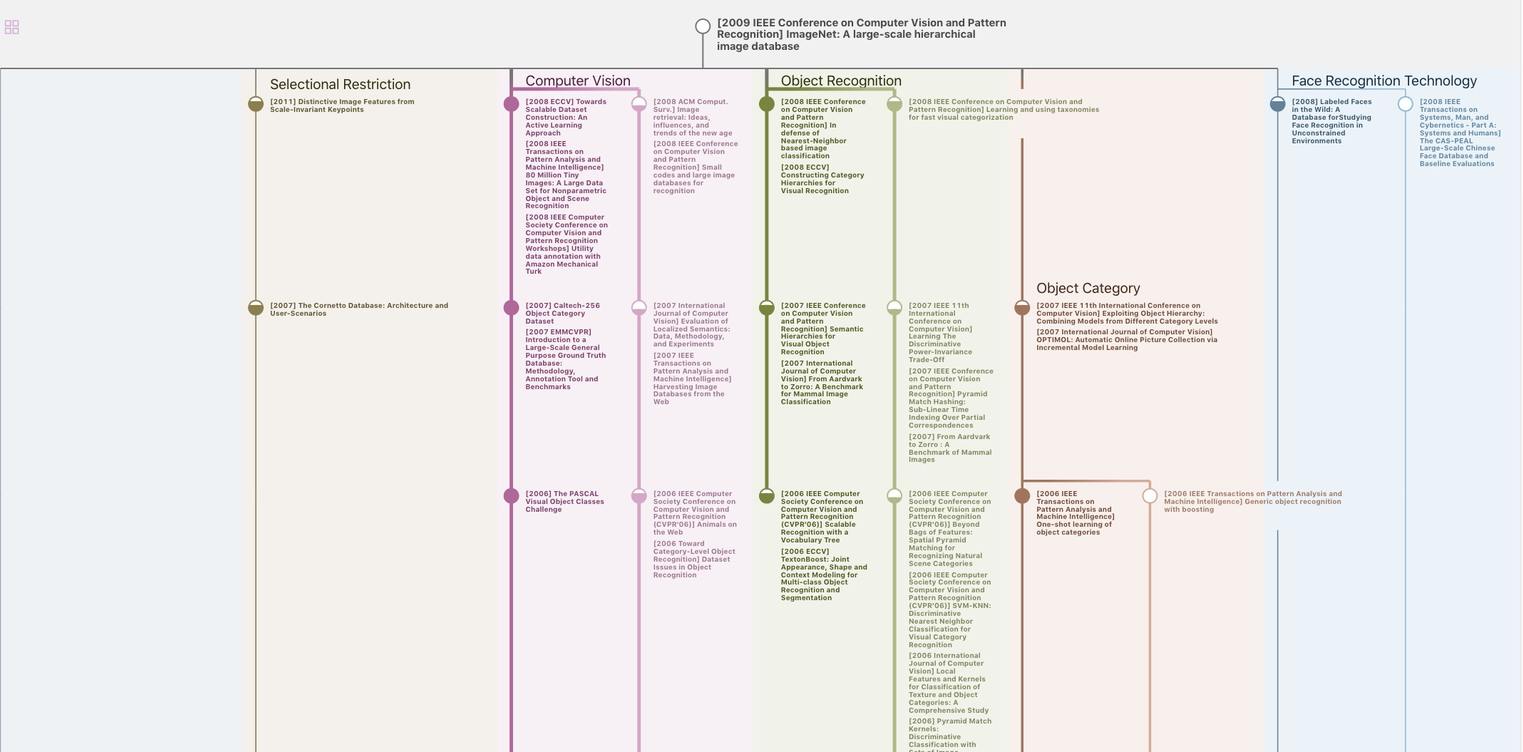
生成溯源树,研究论文发展脉络
Chat Paper
正在生成论文摘要