ConvRNN-T: Convolutional Augmented Recurrent Neural Network Transducers for Streaming Speech Recognition
Conference of the International Speech Communication Association (INTERSPEECH)(2022)
摘要
The recurrent neural network transducer (RNN-T) is a prominent streaming end-to-end (E2E) ASR technology. In RNN-T, the acoustic encoder commonly consists of stacks of LSTMs. Very recently, as an alternative to LSTM layers, the Conformer architecture was introduced where the encoder of RNN-T is replaced with a modified Transformer encoder composed of convolutional layers at the frontend and between attention layers. In this paper, we introduce a new streaming ASR model, Convolutional Augmented Recurrent Neural Network Transducers (ConvRNN-T) in which we augment the LSTM-based RNN-T with a novel convolutional frontend consisting of local and global context CNN encoders. ConvRNN-T takes advantage of causal 1-D convolutional layers, squeeze-and-excitation, dilation, and residual blocks to provide both global and local audio context representation to LSTM layers. We show ConvRNN-T outperforms RNN-T, Conformer, and ContextNet on Librispeech and in-house data. In addition, ConvRNN-T offers less computational complexity compared to Conformer. ConvRNN-T's superior accuracy along with its low footprint make it a promising candidate for on-device streaming ASR technologies.
更多查看译文
关键词
speech recognition,streaming,neural network
AI 理解论文
溯源树
样例
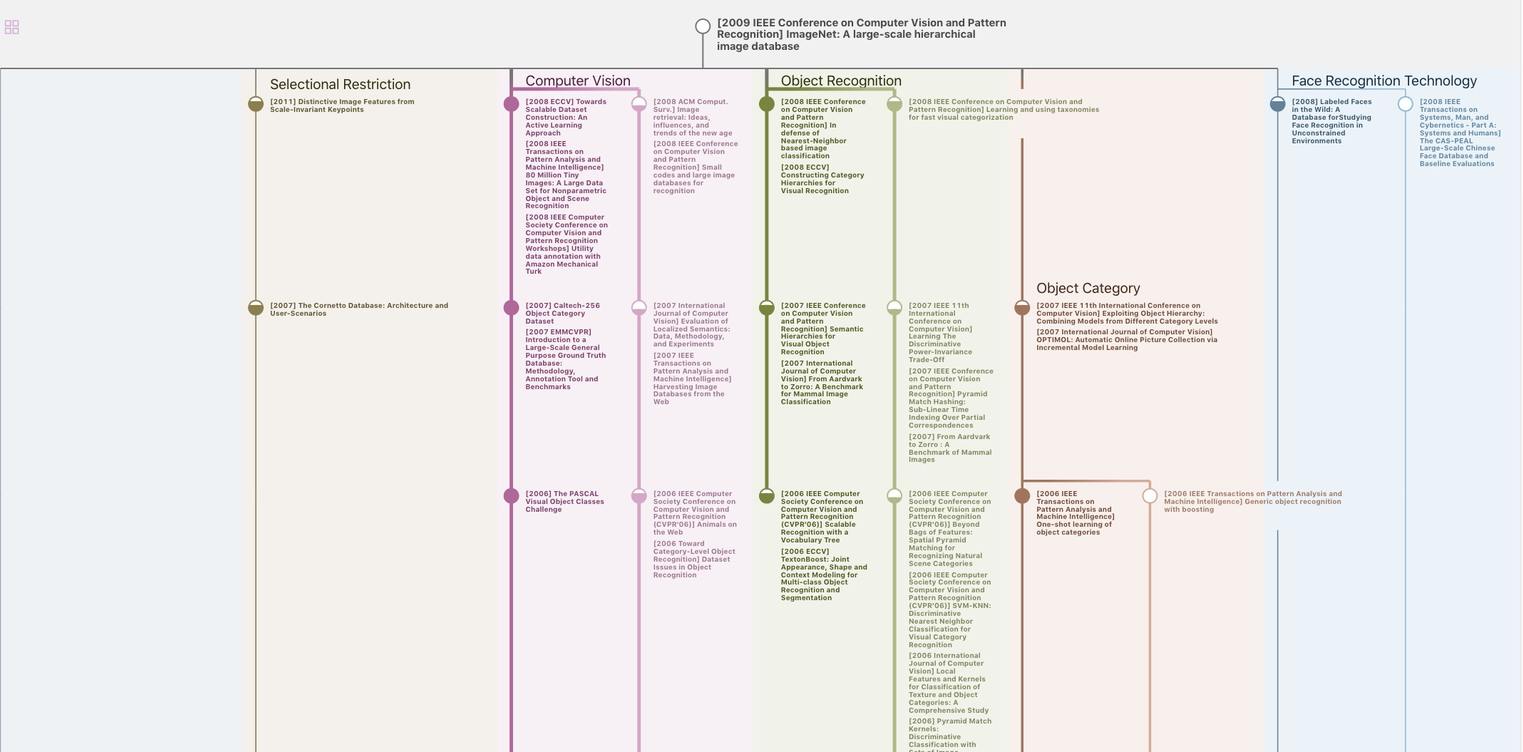
生成溯源树,研究论文发展脉络
Chat Paper
正在生成论文摘要