Perturbations and Subpopulations for Testing Robustness in Token-Based Argument Unit Recognition.
International Conference on Computational Linguistics(2022)
摘要
Argument Unit Recognition and Classification aims at identifying argument units from text and classifying them as pro or against. One of the design choices that need to be made when developing systems for this task is what the unit of classification should be: segments of tokens or full sentences. Previous research suggests that fine-tuning language models on the token-level yields more robust results for classifying sentences compared to training on sentences directly. We reproduce the study that originally made this claim and further investigate what exactly token-based systems learned better compared to sentence-based ones. We develop systematic tests for analysing the behavioural differences between the token-based and the sentence-based system. Our results show that token-based models are generally more robust than sentence-based models both on manually perturbed examples and on specific subpopulations of the data.
更多查看译文
关键词
testing robustness,recognition,token-based
AI 理解论文
溯源树
样例
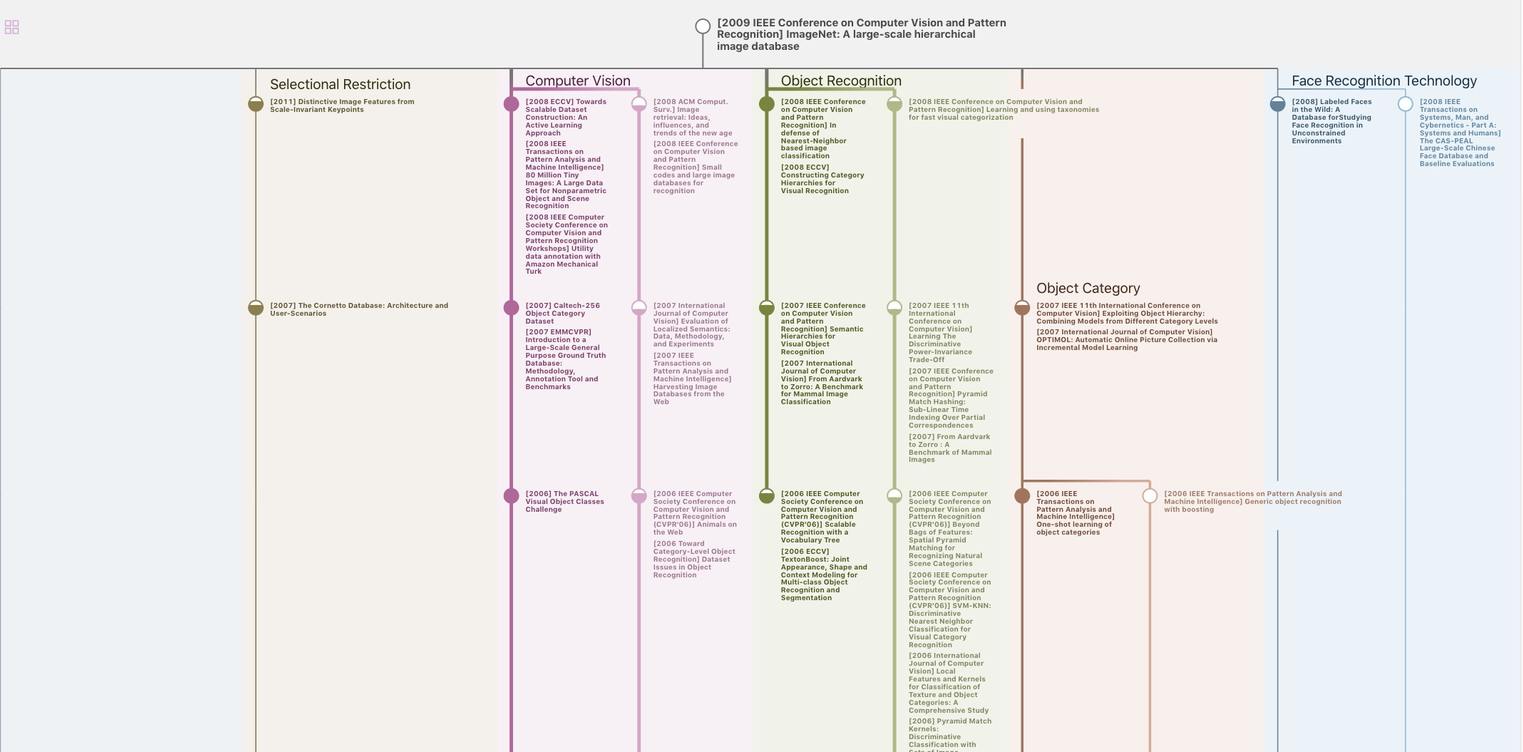
生成溯源树,研究论文发展脉络
Chat Paper
正在生成论文摘要