An office building energy consumption forecasting model with dynamically combined residual error correction based on the optimal model
Energy Reports(2022)
Abstract
Accurate forecasting of energy consumption in office buildings is of great importance for optimal management of energy consumption and reduction of building energy consumption. A variety of combination forecasting models (FMs) have become the current research hotspots in the field of building energy consumption forecasting. For the problems of large systematic errors and poor generalization ability of existing combination FMs, this paper proposes a dynamic combination residual forecasting model (FM) with the optimal combination approach. Firstly, support vector regression (SVR) is selected as the basic FM, and the SVR residual errors are forecasted by the dynamic combination FM based on the weights, and the SVR forecast value is finally corrected. Further, the basis for the selection of the single FM in the combination model and the optimal number of combination terms are given by mathematical proof in this paper. A case study in Xi'an shows that the dynamic combined residual errors correction FM with the optimal number of terms proposed in this paper can reduce the mean absolute error (MAE) of the basic model from 1918.59 kW to 349.37 kW, the mean absolute percentage error (MAPE) from 15.80% to 2.96%, and the root mean square error (RMSE) from 2278.74 to 471.44. (c) 2022 The Authors. Published by Elsevier Ltd. This is an open access article under the CC BY-NC-ND license (http://creativecommons.org/licenses/by-nc-nd/4.0/).
MoreTranslated text
Key words
Building energy forecasting, Combination forecasting, Residual error correction, Support vector regression, Optimal combination model
AI Read Science
Must-Reading Tree
Example
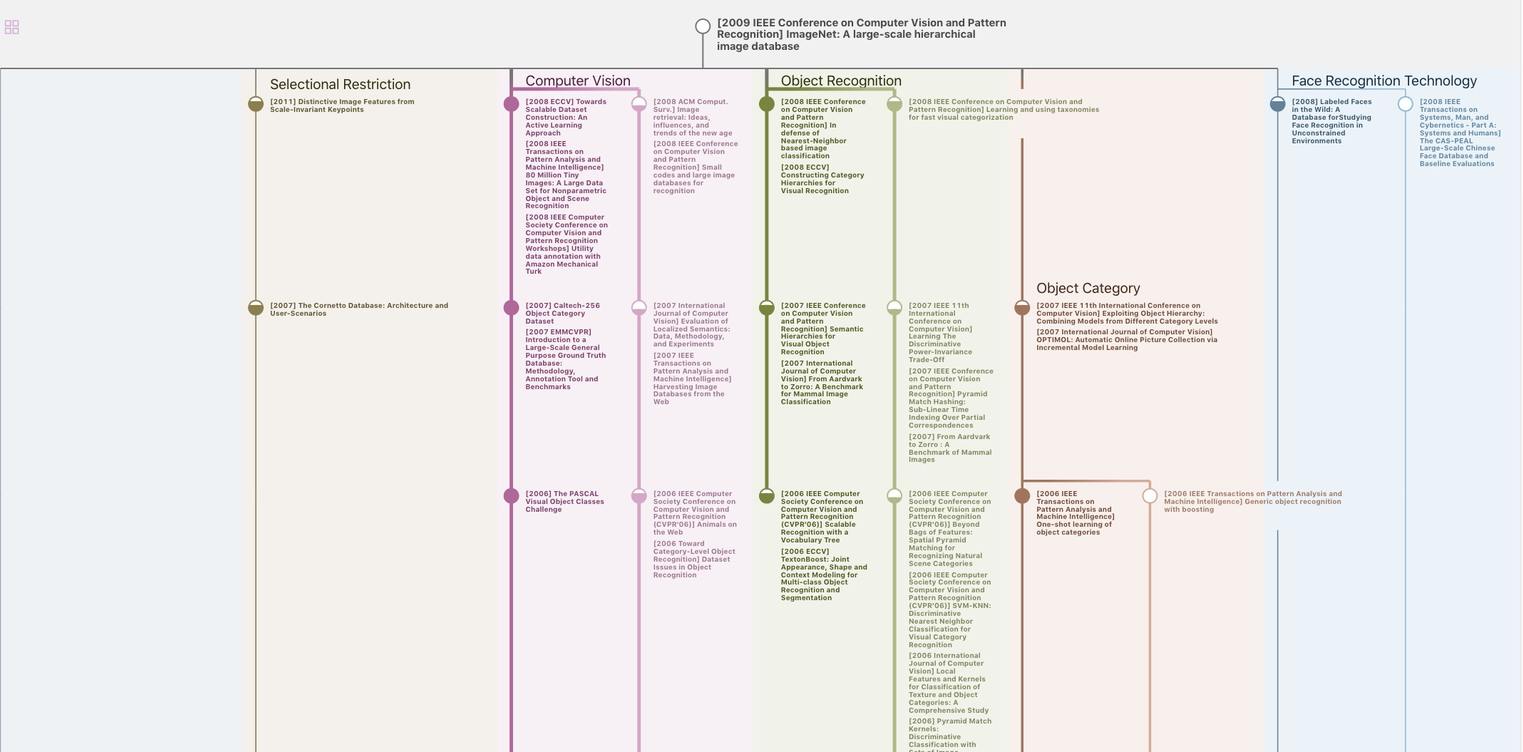
Generate MRT to find the research sequence of this paper
Chat Paper
Summary is being generated by the instructions you defined