C-BDCLSTM: A false emotion recognition model in micro blogs combined Char-CNN with bidirectional dilated convolutional LSTM.
Appl. Soft Comput.(2022)
Abstract
In recent years, researchers are enthusiastic about the field of sentiment computing. They pay attention to normal emotion recognition, but little to abnormal emotion recognition. In social network, users are more and more abnormal emotions, which is easy to generate in the network public opinion. However, in order to achieve different personal purposes, some users with ulterior motives often express some abnormal emotions (such as false emotion) in social networks. Recognizing false emotion correctly and effectively has great significance in analyzing users' original views on goods, movies or hot events on various websites as well as the guidance of public opinion. In this paper, we define the false emotion by extending the definition of normal emotion recognition and propose the task of false emotion recognition based on the six-element basic emotion combined with the idea of abnormal emotion detection. We construct a Char-CNN and bidirectional dilated convolutional LSTM combined model (denoted to C-BDCLSTM) for the task of false emotion recognition in micro blogs. This model is divided into RNN and CNN units, in which RNN unit includes embedding layer, BiLSTM layer, dilation layer, attention mechanism layer, and CNN unit includes convolution pooling layer and multiple filters of different sizes. By combined RNN and CNN units, C-BDCLSTM model will learn the six emotions of micro blog comments and the polarity of micro blog content, and then carry out decision-level fusion to finally complete the task of false emotion recognition. Due to the lack of false emotion recognition data set, we specially annotate and provide a data set about the false emotion recognition of micro blog contents and micro blog comments, which includes a total of ten thousand pieces of record. The experiment results demonstrate that C-BDCLSTM outperform other baselines (BiLSTM, Char-CNN, TextRCNN, LSTM-Attention, Transformer). The F1 score of our proposed C-BDCLSTM model is 0.7259 for the six emotions classification on the data set, and the F1 score of the twelve classification including false emotion is 0.6989. In addition, we also propose an evaluation index FFS for false emotion recognition, and the FFS score of our proposed C-BDCLSTM model is 0.7013. (c) 2022 Elsevier B.V. All rights reserved.
MoreTranslated text
Key words
False emotion recognition,Char-CNN,Bidirectional dilated convolutional LSTM,Sentiment computing,Network fusion
AI Read Science
Must-Reading Tree
Example
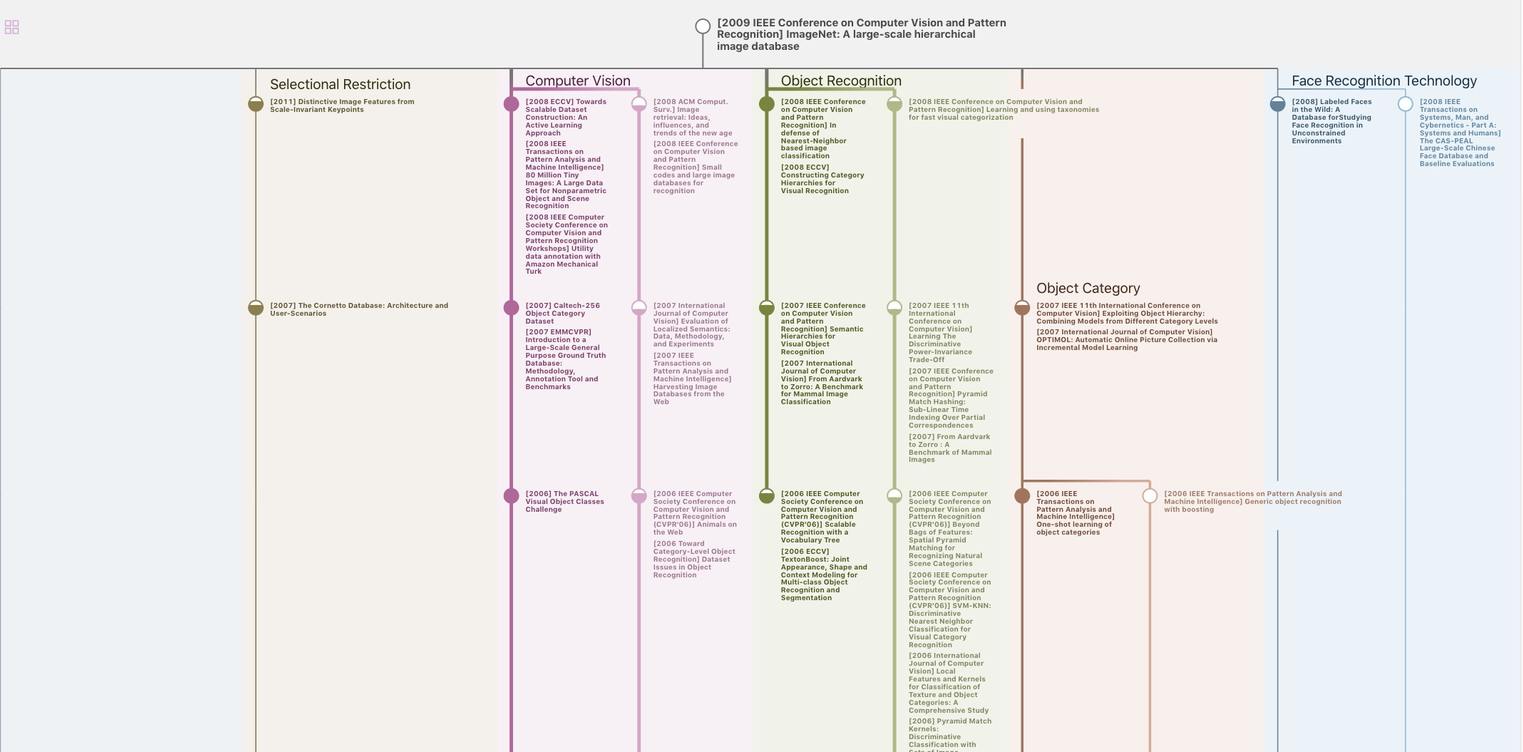
Generate MRT to find the research sequence of this paper
Chat Paper
Summary is being generated by the instructions you defined