Automatic lung tumor segmentation from CT images using improved 3D densely connected UNet
Medical & Biological Engineering & Computing(2022)
摘要
Accurate lung tumor segmentation has great significance in the treatment planning of lung cancer. However, robust lung tumor segmentation becomes challenging due to the heterogeneity of tumors and the similar visual characteristics between tumors and surrounding tissues. Hence, we developed an improved 3D dense connected UNet (I-3D DenseUNet) to segment various lung tumors from CT images. The nested dense skip connection adopted in the I-3D DenseUNet aims to contribute similar feature maps between encoder and decoder sub-networks. The dense connection used in encoder-decoder blocks also encourages feature propagation and reuse. A robust data augmentation strategy was employed to alleviate over-fitting based on a 3D thin plate spline (TPS) algorithm. We evaluated our method on 938 lung tumors from three datasets consisting of 421 tumors from the Cancer Imaging Archive (TCIA), 450 malignant tumors from the Lung Image Database Consortium (LIDC), and 67 tumors from the private dataset. Experiment results showed an excellent Dice similarity coefficients (DSC) of 0.8316 for the TCIA and LIDC and 0.8167 for the private dataset. The proposed method presents a strong ability in lung tumor segmentation, and it has the potential to help radiologists in lung cancer treatment planning. Graphical abstract Framework of the proposed lung tumor segmentation method.
更多查看译文
关键词
Lung tumor segmentation, Nested dense skip connection, Deep learning, Lung cancer
AI 理解论文
溯源树
样例
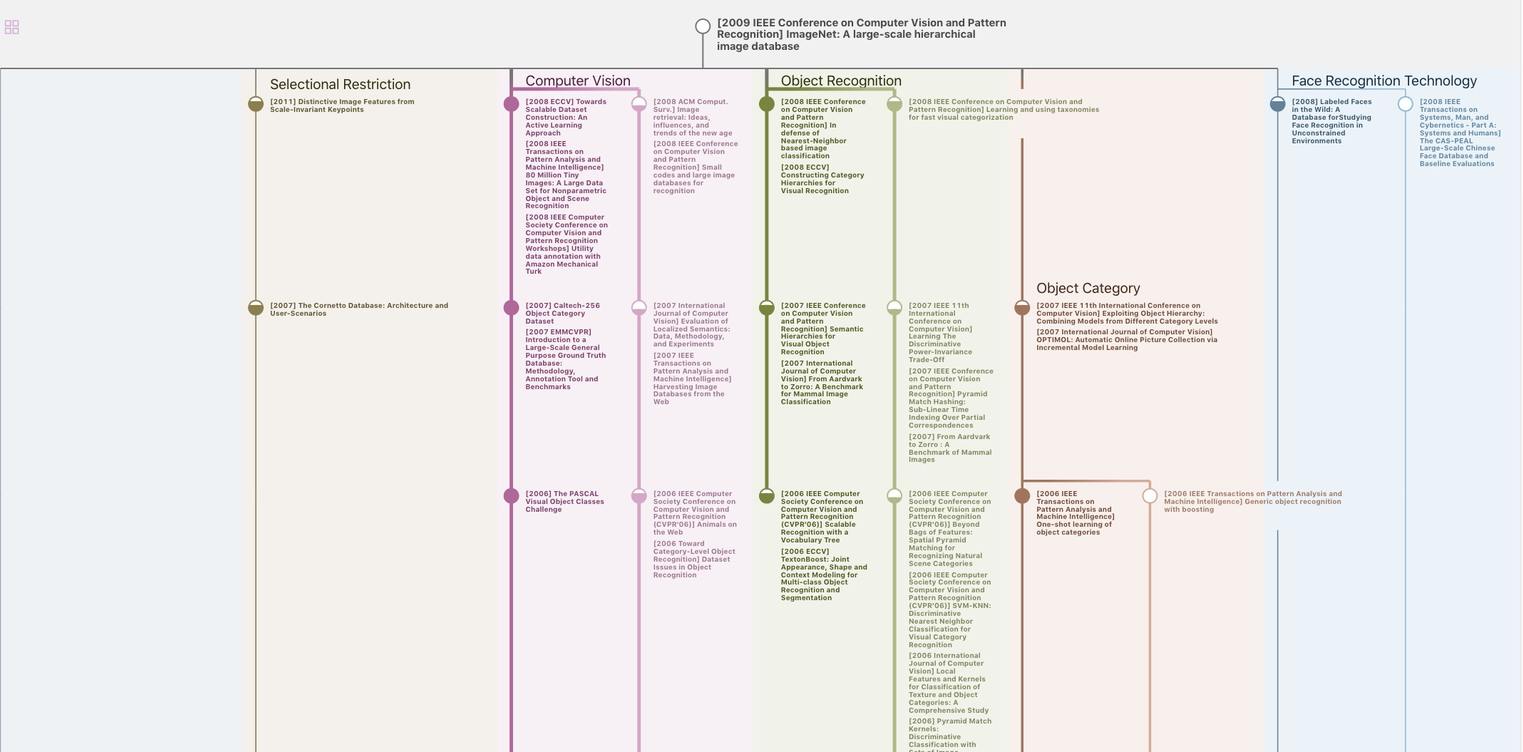
生成溯源树,研究论文发展脉络
Chat Paper
正在生成论文摘要