A study on transfer learning in enhancing performance of building energy system fault diagnosis with extremely limited labeled data
Building and Environment(2022)
摘要
Transfer learning has been proved to be a feasible way to ensure that the data-driven fault diagnosis model for building energy system still has good diagnostic performance with limited labeled data. The traditional transfer learning method assumes that the actual system can provide limited normal and various fault data. However, faulty conditions in actual system are intrinsically much less frequent than normal conditions. Therefore, it is a more real diagnosis scenario that only limited normal data are available. Faced with the extremely limited labeled data, the traditional transfer learning method is no longer applicable. This study proposes a novel fault diagnosis method based on feature transformation transfer learning. The main idea is to adopt a prior hypothesis to guide the source domain (data sources with sufficient labeled data) to perform feature transformation, and then leverages the fault characteristic knowledge learned from the source domain to facilitate diagnosis tasks in target domain (the actual system with limited normal data). Multiple experiments have been designed to eval-uate and verify, the results show proposed method has obvious advantages over traditional transfer learning method, with the highest accuracy improvement of 0.21, and can achieve satisfactory accuracy, up to 0.9. The insights obtained are valuable for promoting the application of fault diagnosis model in practice.
更多查看译文
关键词
Transfer learning, Fault diagnosis, Feature transformation, Building energy system, Deep learning
AI 理解论文
溯源树
样例
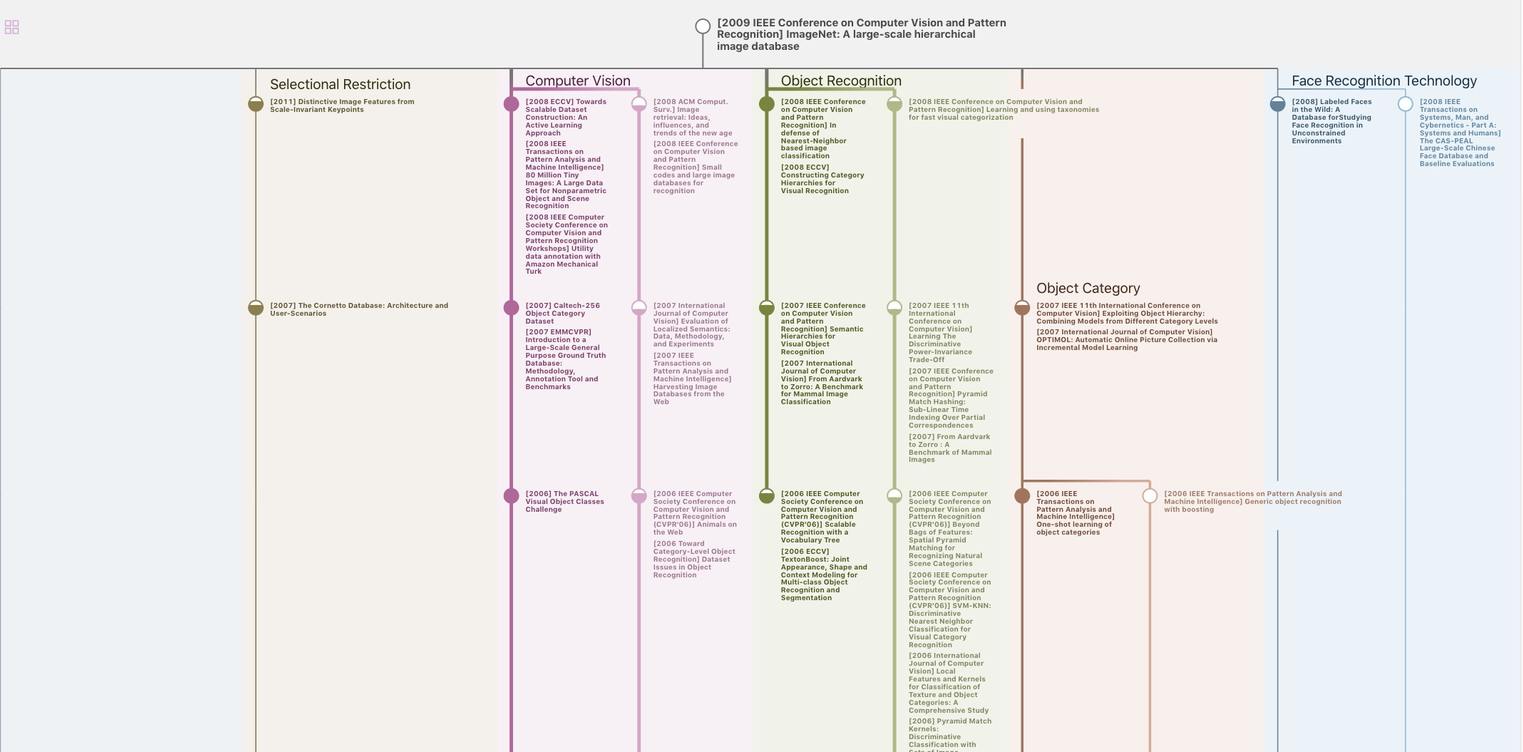
生成溯源树,研究论文发展脉络
Chat Paper
正在生成论文摘要