SPSRec: An Efficient Signal Phase Recommendation for Signalized Intersections With GAN and Decision-Tree Model
IEEE Transactions on Intelligent Transportation Systems(2023)
摘要
Signal phase optimization at signalized intersection is of great importance to urban traffic control and management yet is very challenging. The traditional approaches for signal phase optimization heavily rely on the traffic engineering practitioners’ experience. To tackle these challenges, a novel data-driven method is proposed to realize signal phase optimization and recommendation solely using limited amount of real signalized intersection samples. Firstly, all of discrete features related to signal phase design, encoded by one-hot representation, are sampled by the Gumbel-SoftMax distribution, which is a continuous approximation to a multinomial distribution. With this approximation distribution, the generative adversarial network (GAN) is applied to produce the most acceptable signal phase samples among all acceptable choices, dealing with the problem of insufficient samples and uneven sample distribution in real word. Thirdly, a decision-tree based classifier is established to realize signal phase recommendation automatically. We conducted extensive experiments to evaluate our proposed method on three cities in China, including Beijing, Tongxiang and Chaozhou. The experimental results showed that the proposed method could effectively improve the signalized intersection operation efficiency. Moreover, the proposed method has already been deployed in several cities, and it successfully keeps serving hundreds of signalized intersections. This confirms that SPSRec is a practical and robust solution for large-scale real-world signal control services.
更多查看译文
关键词
Optimization,Urban areas,Generative adversarial networks,Vehicle dynamics,Geometry,Feature extraction,Delays,Signal phase design representation,generative adversarial network (GAN),decision-tree model,signalized intersection control
AI 理解论文
溯源树
样例
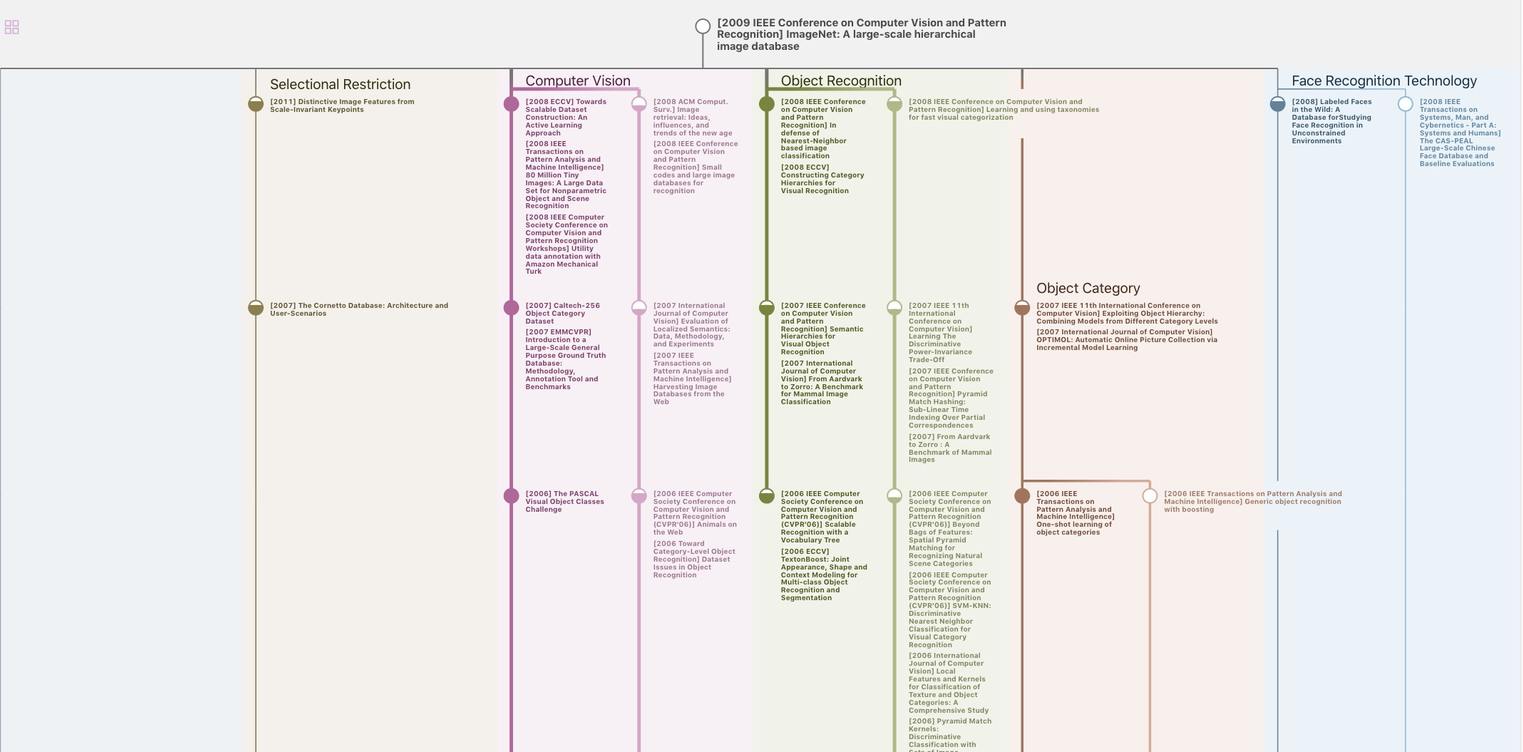
生成溯源树,研究论文发展脉络
Chat Paper
正在生成论文摘要