Analysis of noisy transient signals based on Gaussian process regression.
Biophysical journal(2023)
摘要
Dynamic systems such as cells or tissues generate, either spontaneously or in response to stimuli, transient signals that carry information about the system. Characterization of recorded transients is often hampered by a low signal-to-noise ratio (SNR). Reduction of the noise by filtering has limited use due to partial signal distortion. Occasionally, transients can be approximated by a mathematical function, but such a function may not hold correctly if recording conditions change. We introduce here the model-independent approximation method for general noisy transient signals based on the Gaussian process regression. The method was implemented in the software TransientAnalyzer, which detects transients in a record, finds their best approximation by the Gaussian process, constructs a surrogate spline function, and estimates specified signal parameters. The method and software were tested on a cellular model of the calcium concentration transient corrupted by various SNR levels and recorded at a low sampling frequency. Statistical analysis of the model data sets provided the error of estimation <7.5% and the coefficient of variation of estimates <17% for peak SNR = 5. The performance of Gaussian process regression on signals of diverse experimental origin was even better than fitting by a function. The software and its description are available on GitHub.
更多查看译文
关键词
Data analysis,Gaussian process,noise,nonlinear regression,transient signal
AI 理解论文
溯源树
样例
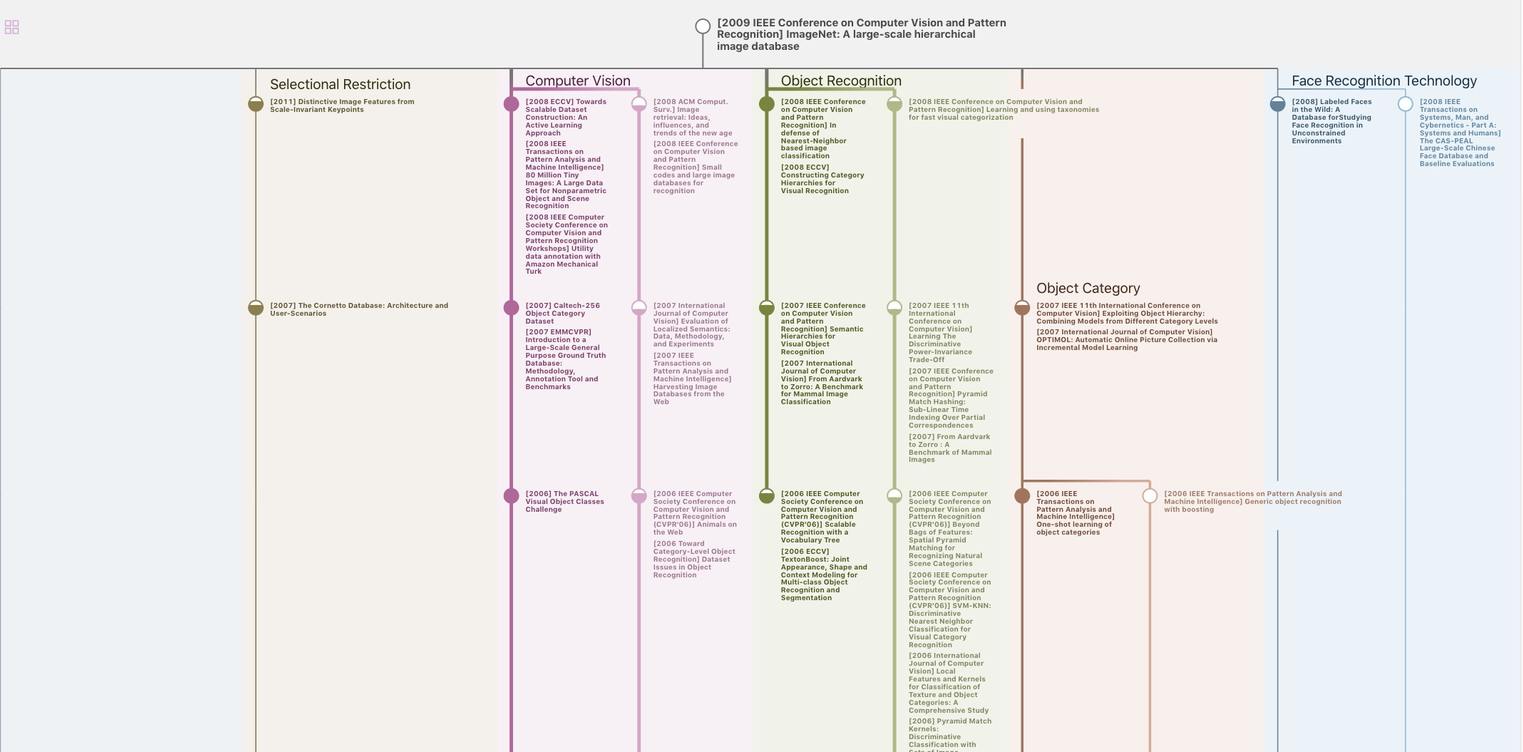
生成溯源树,研究论文发展脉络
Chat Paper
正在生成论文摘要