Active Transfer Prototypical Network: An Efficient Labeling Algorithm for Time-Series Data
arxiv(2022)
摘要
The paucity of labeled data is a typical challenge in the automotive industry. Annotating time-series measurements requires solid domain knowledge and in-depth exploratory data analysis, which implies a high labeling effort. Conventional Active Learning (AL) addresses this issue by actively querying the most informative instances based on the estimated classification probability and retraining the model iteratively. However, the learning efficiency strongly relies on the initial model, resulting in the trade-off between the size of the initial dataset and the query number. This paper proposes a novel Few-Shot Learning (FSL)-based AL framework, which addresses the trade-off problem by incorporating a Prototypical Network (ProtoNet) in the AL iterations. The results show an improvement, on the one hand, in the robustness to the initial model and, on the other hand, in the learning efficiency of the ProtoNet through the active selection of the support set in each iteration. This framework was validated on UCI HAR/HAPT dataset and a real-world braking maneuver dataset. The learning performance significantly surpasses traditional AL algorithms on both datasets, achieving 90% classification accuracy with 10% and 5% labeling effort, respectively.
更多查看译文
关键词
active transfer prototypical network,efficient labeling algorithm,data,time-series
AI 理解论文
溯源树
样例
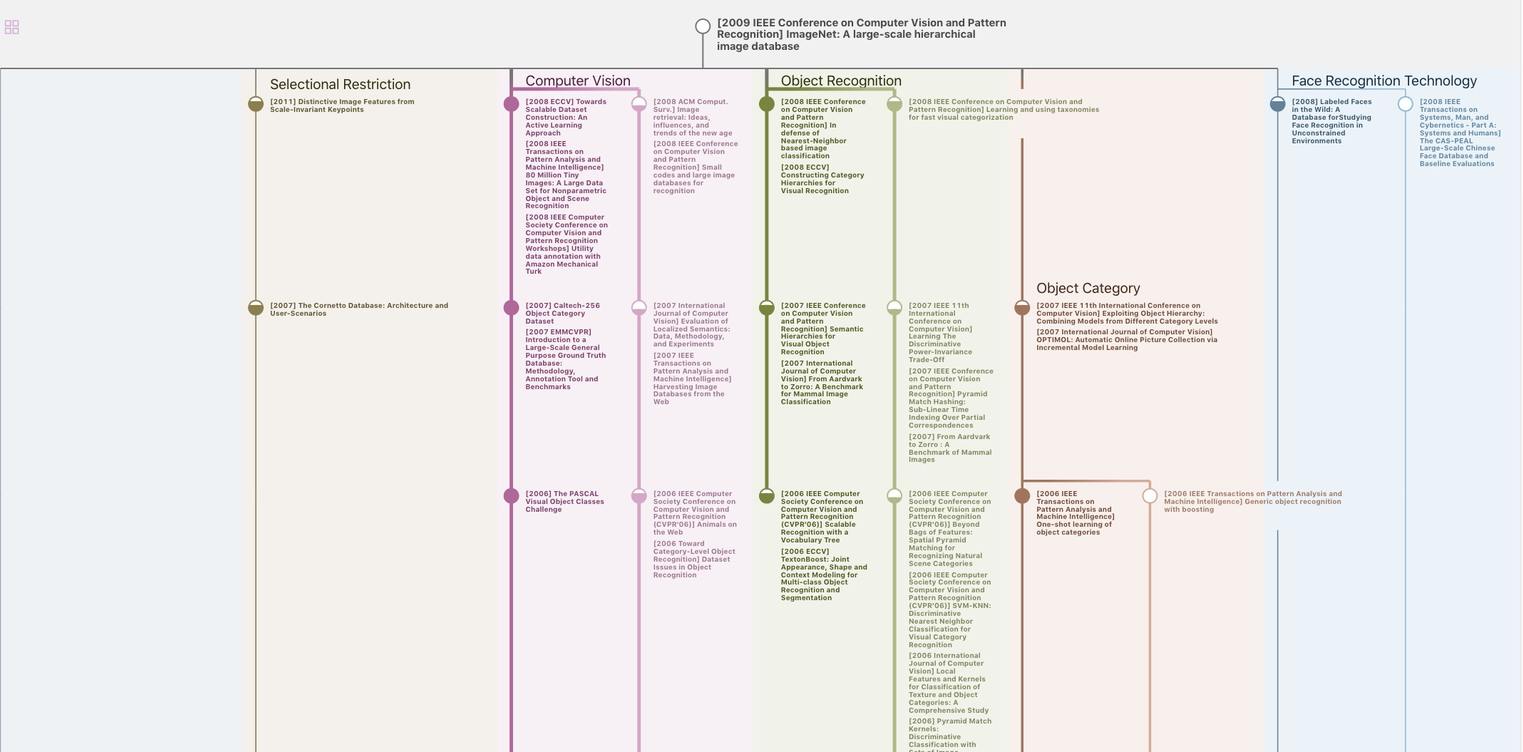
生成溯源树,研究论文发展脉络
Chat Paper
正在生成论文摘要