MUTE-Reco: MUTual Information Assisted Ensemble Feature RECOmmender System for Healthcare Prognosis
arxiv(2022)
摘要
Purpose: Health recommenders act as important decision support systems,
aiding patients and medical professionals in taking actions that lead to
patients' well-being. These systems extract the information which may be of
particular relevance to the end-user, helping them in making appropriate
decisions. The present study proposes a feature recommender that identifies and
recommends the most important risk factors for healthcare prognosis.
Methods: A novel mutual information and ensemble-based feature ranking
approach (termed as, MUTE-Reco) considering the rank of features obtained from
eight popular feature selection methods, is proposed.
Results: To establish the effectiveness of the proposed method, the
experiment has been conducted on four benchmark datasets of diverse diseases
(clear cell renal cell carcinoma (ccRCC), chronic kidney disease, Indian liver
patient, and cervical cancer risk factors). The performance of the proposed
recommender is compared with four state-of-the-art methods using recommender
systems' performance metrics like average precision@K, precision@K, recall@K,
F1@K, reciprocal rank@K. Experimental results show that the model built with
the recommended features can attain a higher accuracy (96.6
support vector machine and neural network, respectively) for classifying
different stages of ccRCC with a reduced feature set as compared to existing
methods. Moreover, the top two features recommended using the proposed method
with ccRCC, viz. size of tumor and metastasis status, are medically validated
from the existing TNM system. Results are also found to be superior for the
other three datasets.
Conclusion: The proposed recommender, MUTE-Reco, can identify and recommend
risk factors that have the most discriminating power for detecting diseases.
更多查看译文
AI 理解论文
溯源树
样例
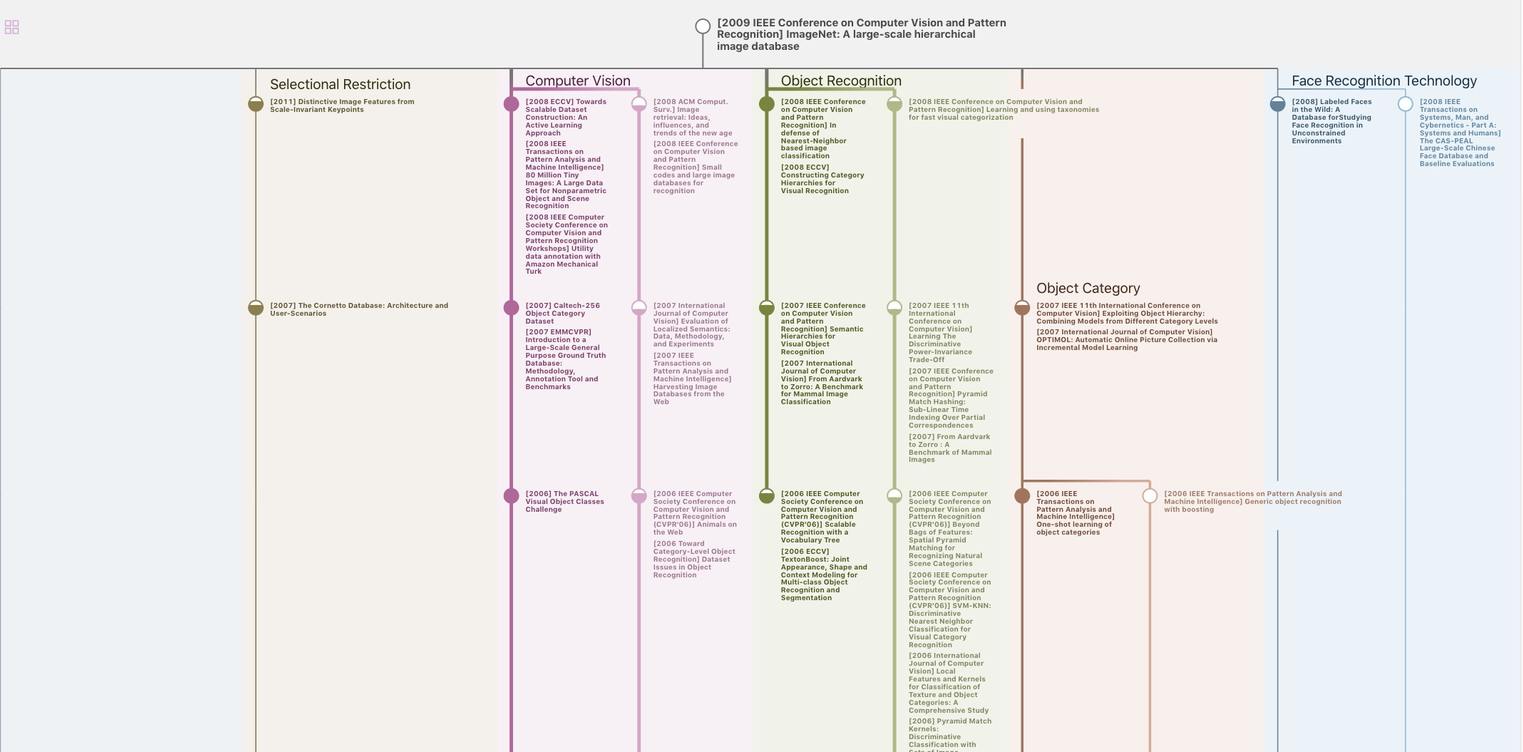
生成溯源树,研究论文发展脉络
Chat Paper
正在生成论文摘要