Generalizability and Bias in a Deep Learning Pediatric Bone Age Prediction Model Using Hand Radiographs
Radiology(2023)
摘要
Background: Although deep learning (DL) models have demonstrated expert-level ability for pediatric bone age prediction, they have shown poor generalizability and bias in other use cases.Purpose: To quantify generalizability and bias in a bone age DL model measured by performance on external versus internal test sets and performance differences between different demographic groups, respectively.Materials and Methods: The winning DL model of the 2017 RSNA Pediatric Bone Age Challenge was retrospectively evaluated and trained on 12 611 pediatric hand radiographs from two U.S. hospitals. The DL model was tested from September 2021 to December 2021 on an internal validation set and an external test set of pediatric hand radiographs with diverse demographic representation. Images reporting ground-truth bone age were included for study. Mean absolute difference (MAD) between ground-truth bone age and the model prediction bone age was calculated for each set. Generalizability was evaluated by comparing MAD between internal and external evaluation sets with use of t tests. Bias was evaluated by comparing MAD and clinically significant error rate (rate of errors changing the clinical diagnosis) between demographic groups with use oft tests or analysis of variance and chi 2 tests, respectively (statistically significant difference defined as P < .05).Results: The internal validation set had images from 1425 individuals (773 boys), and the external test set had images from 1202 individuals (mean age, 133 months +/- 60 [SD]; 614 boys). The bone age model generalized well to the external test set, with no difference in MAD (6.8 months in the validation set vs 6.9 months in the external set; P = .64). Model predictions would have led to clinically significant errors in 194 of 1202 images (16%) in the external test set. The MAD was greater for girls than boys in the internal validation set (P = .01) and in the subcategories of age and Tanner stage in the external test set (P < .001 for both).Conclusion: A deep learning (DL) bone age model generalized well to an external test set, although clinically significant sex-, age-, and sexual maturity-based biases in DL bone age were identified. (c) RSNA, 2022
更多查看译文
关键词
bone,deep learning,pediatric,prediction
AI 理解论文
溯源树
样例
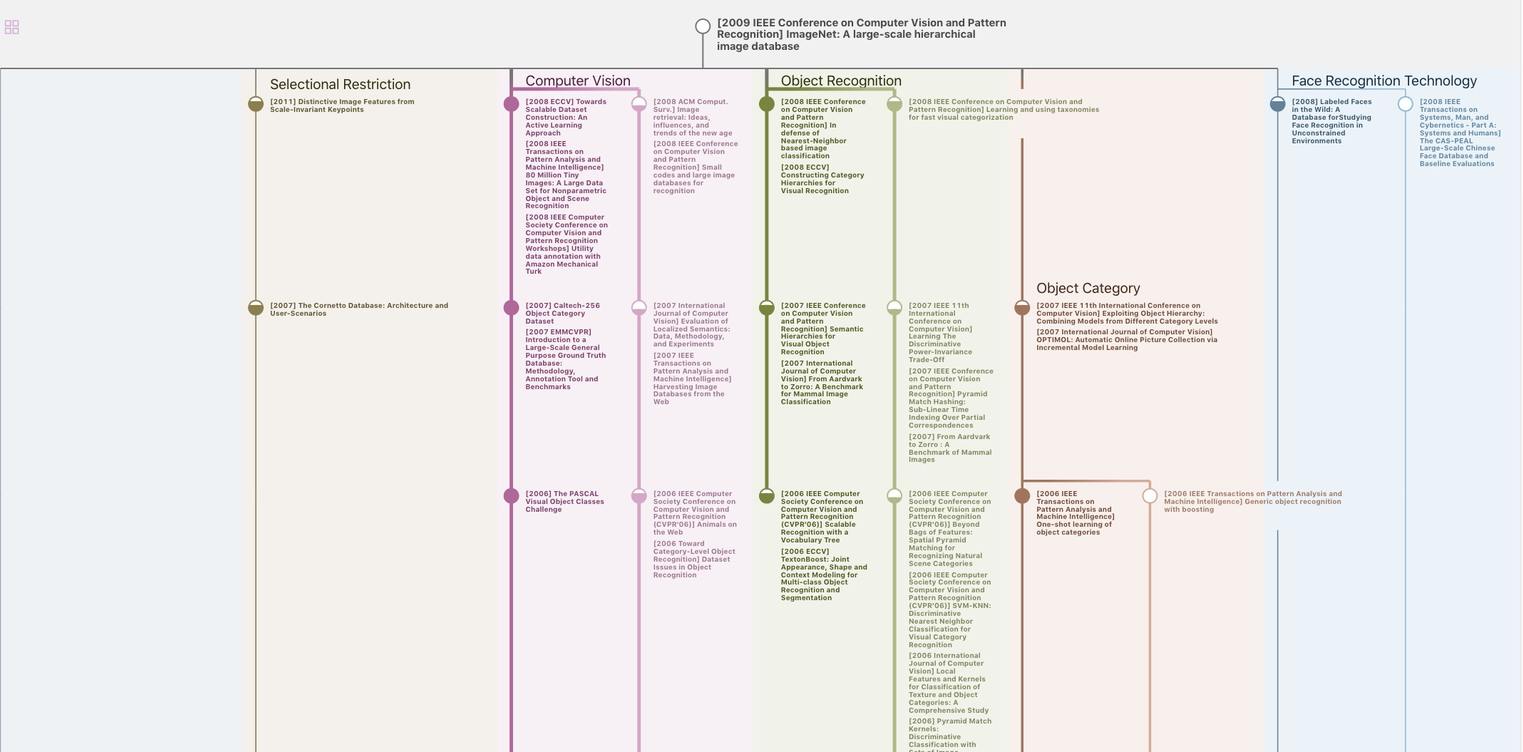
生成溯源树,研究论文发展脉络
Chat Paper
正在生成论文摘要