VDDB: a comprehensive resource and machine learning platform for antiviral drug discovery
arXiv (Cornell University)(2022)
Abstract
Virus infection is one of the major diseases that seriously threaten human health. To meet the growing demand for mining and sharing data resources related to antiviral drugs and to accelerate the design and discovery of new antiviral drugs, we presented an open-access antiviral drug resource and machine learning platform (VDDB), which, to the best of our knowledge, is the first comprehensive dedicated resource for experimentally verified potential drugs/molecules based on manually curated data. Currently, VDDB highlights 848 clinical vaccines, 199 clinical antibodies, as well as over 710,000 small molecules targeting 39 medically important viruses including SARS-CoV-2. Furthermore, VDDB stores approximately 3 million records of pharmacological data for these collected potential antiviral drugs/molecules, involving 314 cell infection-based phenotypic and 234 target-based genotypic assays. Based on these annotated pharmacological data, VDDB allows users to browse, search and download reliable information about these collects for various viruses of interest. In particular, VDDB also integrates 57 cell infection- and 117 target-based associated high-accuracy machine learning models to support various antivirals identification-related tasks, such as compound activity prediction, virtual screening, drug repositioning and target fishing. VDDB is freely accessible at http://vddb.idruglab.cn.
MoreTranslated text
Key words
compound activity prediction,drug repositioning,target fishing,virtual screening
AI Read Science
Must-Reading Tree
Example
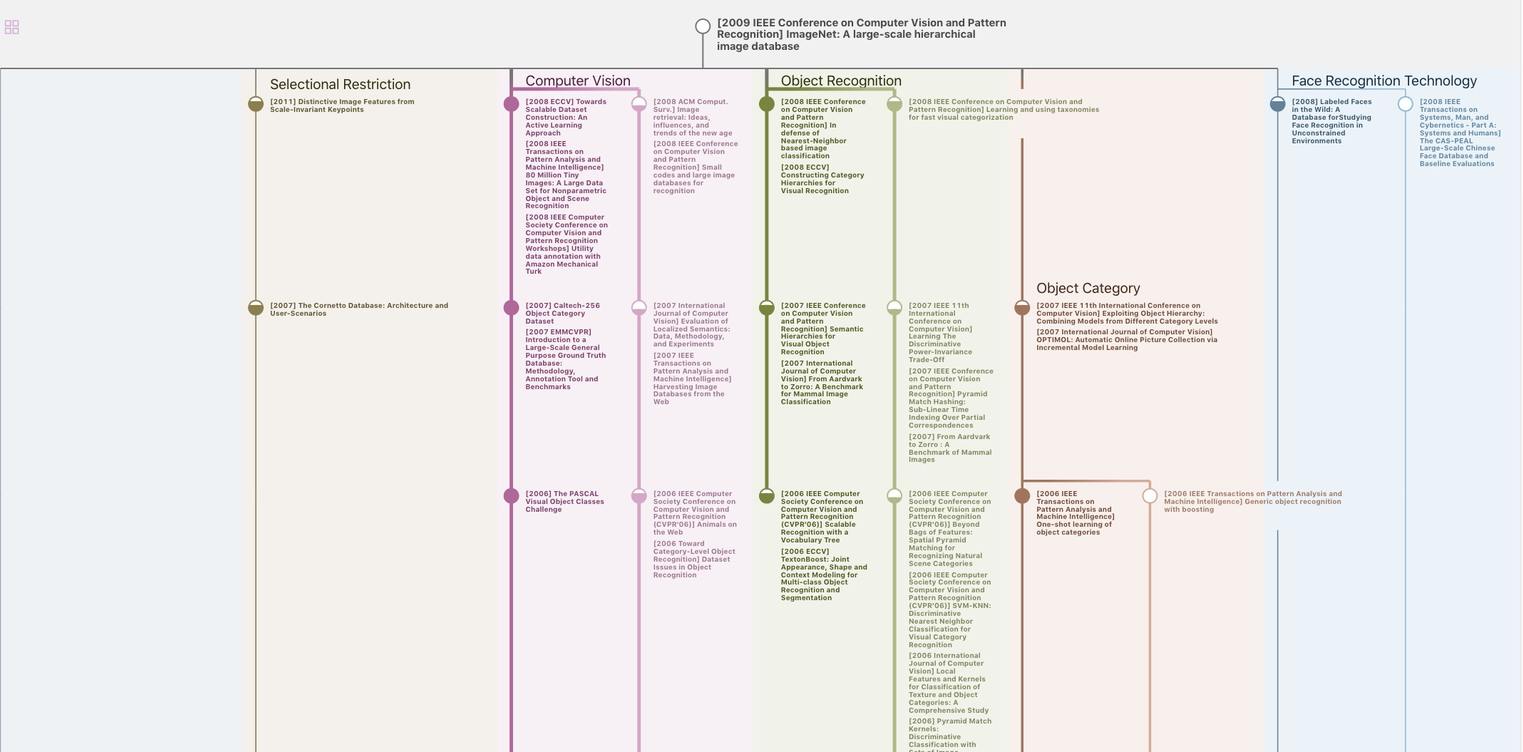
Generate MRT to find the research sequence of this paper
Chat Paper
Summary is being generated by the instructions you defined