Personalized Game Dificulty Prediction Using Factorization Machines
User Interface Software and Technology(2022)
Abstract
The accurate and personalized estimation of task difculty provides many opportunities for optimizing user experience. However, user diversity makes such difculty estimation hard, in that empirical measurements from some user sample do not necessarily generalize to others. In this paper, we contribute a new approach for personalized difculty estimation of game levels, borrowing methods from content recommendation. Using factorization machines (FM) on a large dataset from a commercial puzzle game, we are able to predict diffculty as the number of attempts a player requires to pass future game levels, based on observed attempt counts from earlier levels and levels played by others. In addition to performance and scalability, FMs ofer the beneft that the learned latent variable model can be used to study the characteristics of both players and game levels that contribute to difculty. We compare the approach to a simple non-personalized baseline and a personalized prediction using Random Forests. Our results suggest that FMs are a promising tool enabling game designers to both optimize player experience and learn more about their players and the game.
MoreTranslated text
Key words
Factorization Machines, games, player modelling
AI Read Science
Must-Reading Tree
Example
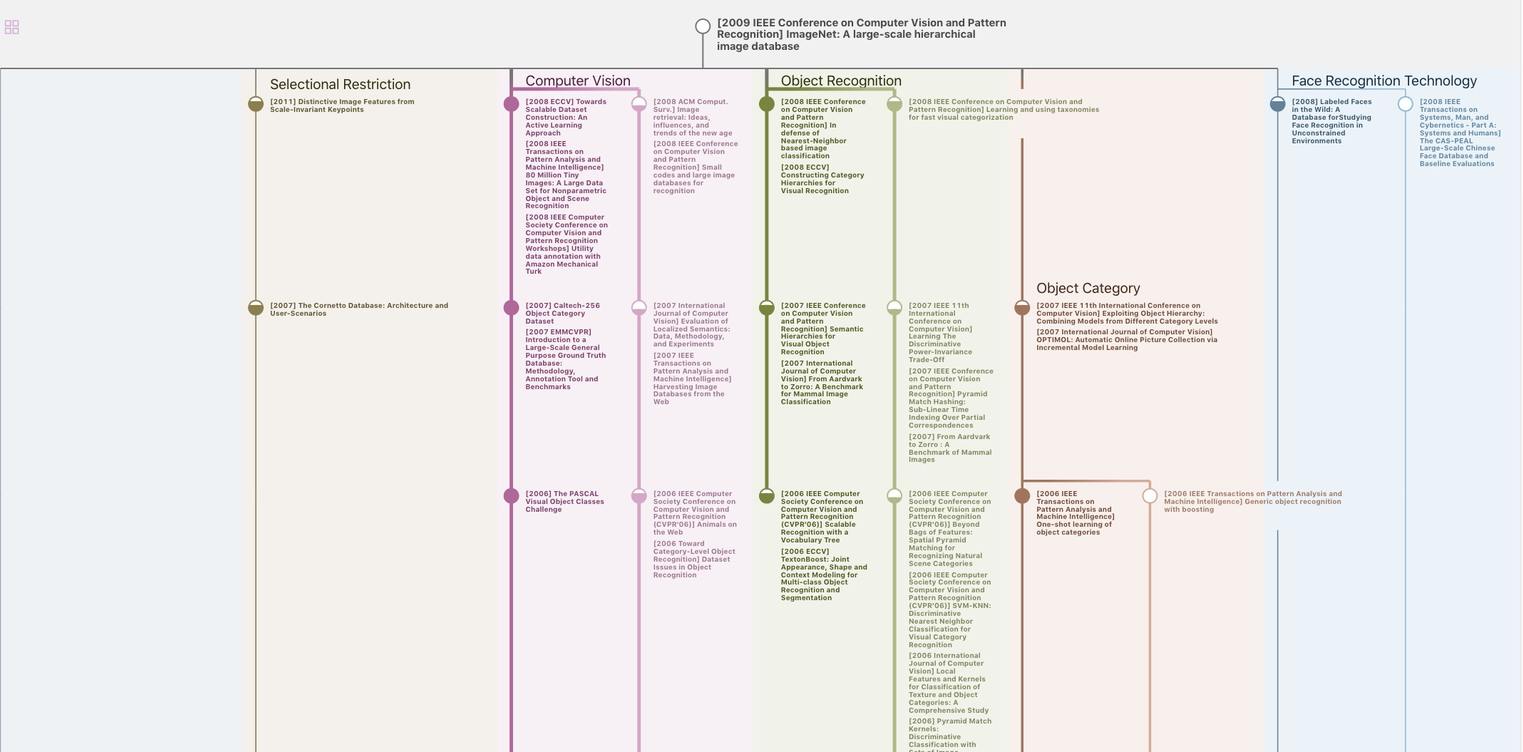
Generate MRT to find the research sequence of this paper
Chat Paper
Summary is being generated by the instructions you defined