Dynamics-Aware Spatiotemporal Occupancy Prediction in Urban Environments.
IEEE/RJS International Conference on Intelligent RObots and Systems (IROS)(2022)
摘要
Detection and segmentation of moving obstacles, along with prediction of the future occupancy states of the local environment, are essential for autonomous vehicles to proactively make safe and informed decisions. In this paper, we propose a framework that integrates the two capabilities together using deep neural network architectures. Our method first detects and segments moving objects in the scene, and uses this information to predict the spatiotemporal evolution of the environment around autonomous vehicles. To address the problem of direct integration of both static-dynamic object segmentation and environment prediction models, we propose using occupancy-based environment representations across the whole framework. Our method is validated on the real-world Waymo Open Dataset and demonstrates higher prediction accuracy than baseline methods.
更多查看译文
关键词
occupancy,urban environments,dynamics-aware
AI 理解论文
溯源树
样例
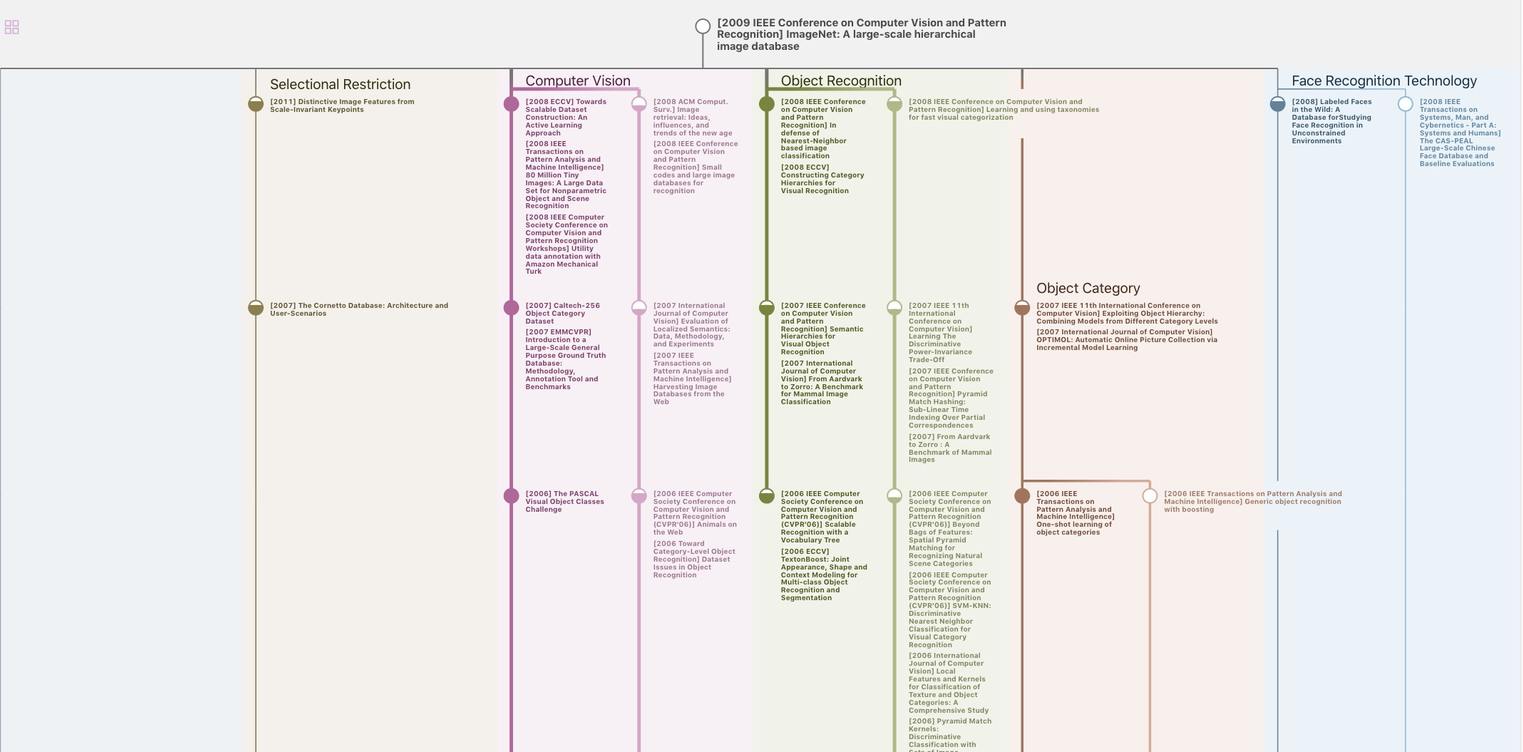
生成溯源树,研究论文发展脉络
Chat Paper
正在生成论文摘要