RepsNet: Combining Vision with Language for Automated Medical Reports
Medical Image Computing and Computer Assisted Intervention – MICCAI 2022(2022)
摘要
Writing reports by analyzing medical images is error-prone for inexperienced practitioners and time consuming for experienced ones. In this work, we present RepsNet that adapts pre-trained vision and language models to interpret medical images and generate automated reports in natural language. RepsNet consists of an encoder-decoder model: the encoder aligns the images with natural language descriptions via contrastive learning, while the decoder predicts answers by conditioning on encoded images and prior context of descriptions retrieved by nearest neighbour search. We formulate the problem in a visual question answering setting to handle both categorical and descriptive natural language answers. We perform experiments on two challenging tasks of medical visual question answering (VQA-Rad) and report generation (IU-Xray) on radiology image datasets. Results show that RepsNet outperforms state-of-the-art methods with
$$81.08 \%$$
classification accuracy on VQA-Rad 2018 and 0.58 BLEU-1 score on IU-Xray. Supplementary details are available at:
https://sites.google.com/view/repsnet
.
更多查看译文
关键词
Vision and language, Visual question answering, Report generation
AI 理解论文
溯源树
样例
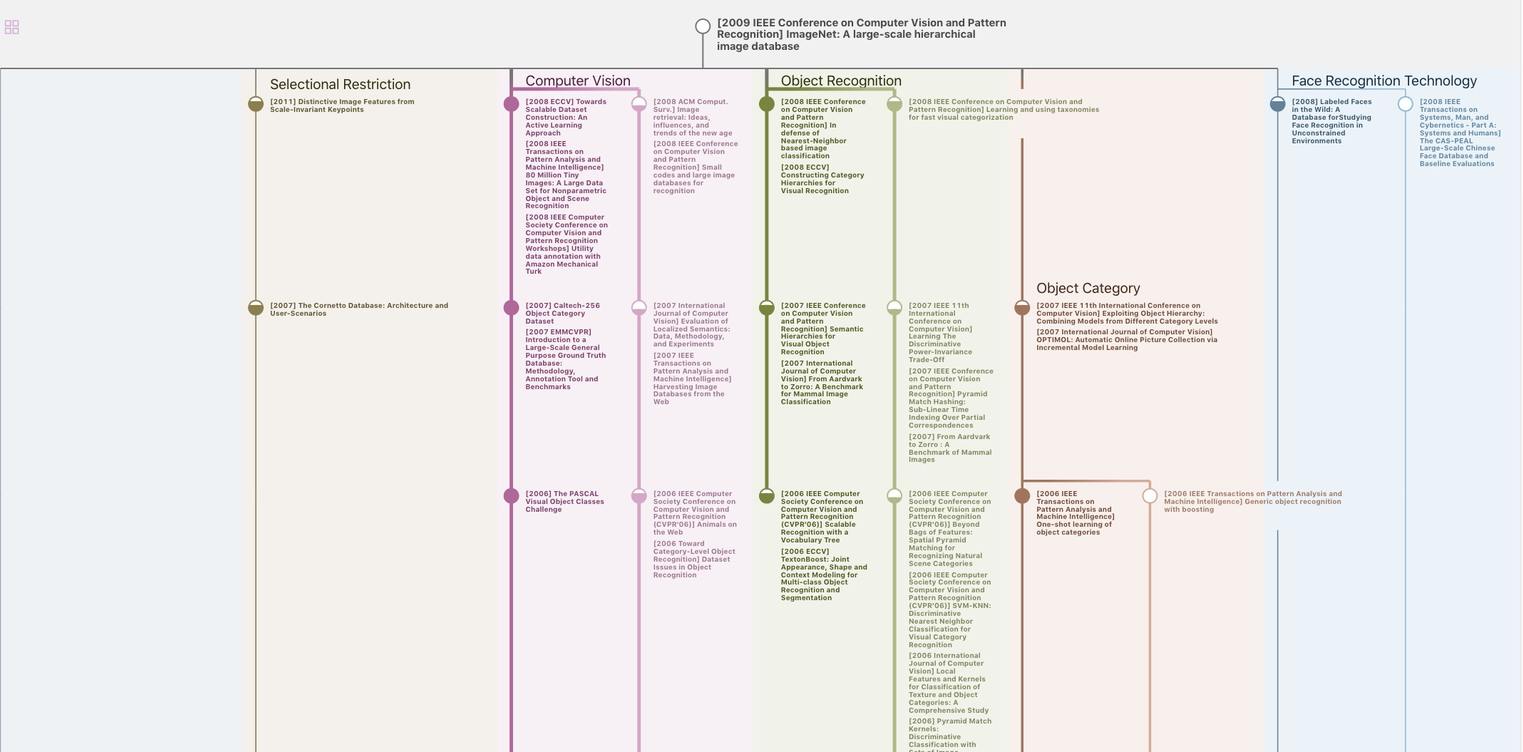
生成溯源树,研究论文发展脉络
Chat Paper
正在生成论文摘要