A Neural Network Approach for Stochastic Optimal Control
arxiv(2022)
摘要
We present a neural network approach for approximating the value function of high-dimensional stochastic control problems. Our training process simultaneously updates our value function estimate and identifies the part of the state space likely to be visited by optimal trajectories. Our approach leverages insights from optimal control theory and the fundamental relation between semi-linear parabolic partial differential equations and forward-backward stochastic differential equations. To focus the sampling on relevant states during neural network training, we use the stochastic Pontryagin maximum principle (PMP) to obtain the optimal controls for the current value function estimate. By design, our approach coincides with the method of characteristics for the non-viscous Hamilton-Jacobi-Bellman equation as the dynamics become deterministic. Our training loss consists of a weighted sum of the objective functional of the control problem and penalty terms that enforce the HJB equations along the sampled trajectories. Importantly, training is unsupervised in that it does not require solutions of the control problem. In our numerical experiments, we compare our method to existing solvers for a more general class of semi-linear PDEs. Using a two-dimensional model problem, we demonstrate the importance of the stochastic PMP to inform the sampling. For a 100-dimensional benchmark problem, we demonstrate that our approach improves accuracy and time-to-solution.
更多查看译文
关键词
optimal control,neural network approach,neural network
AI 理解论文
溯源树
样例
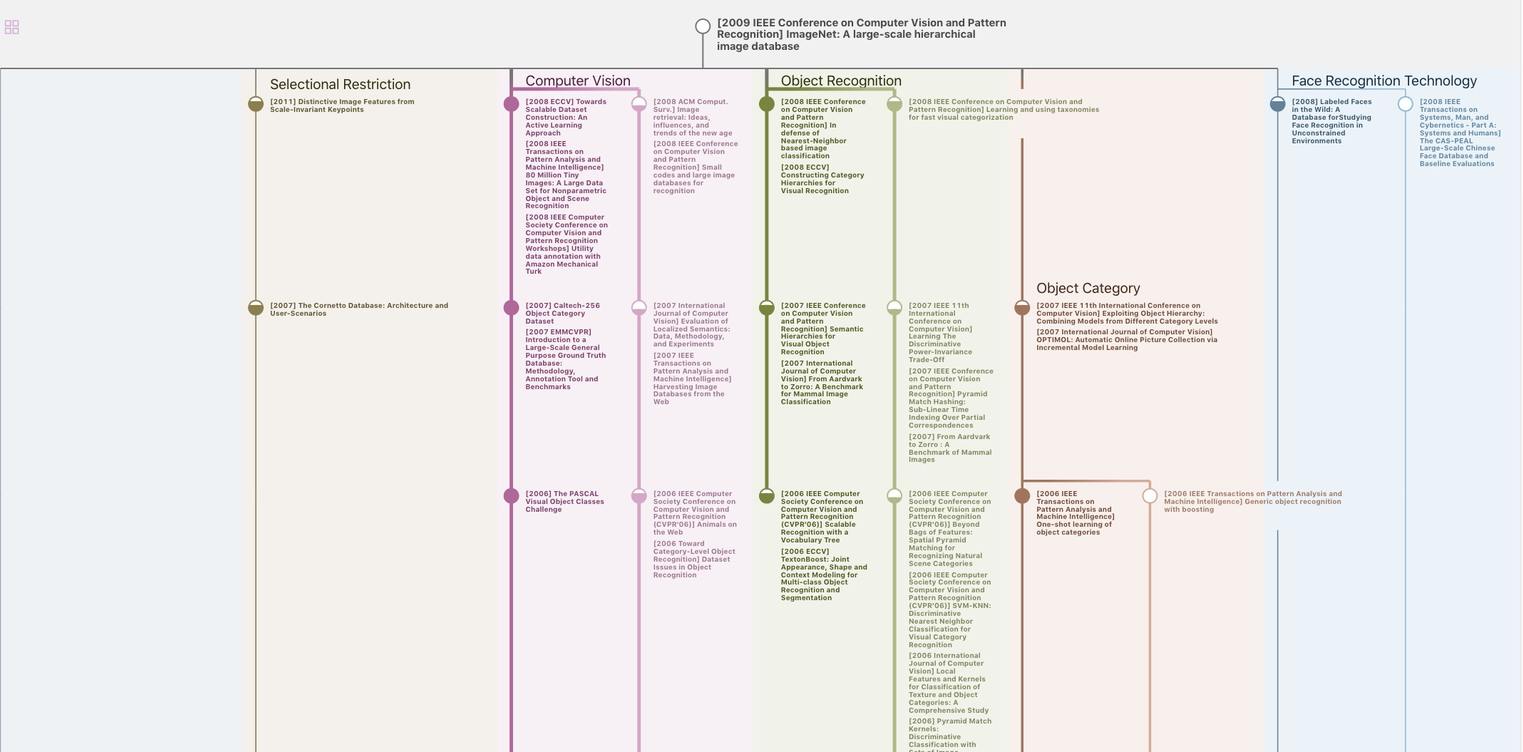
生成溯源树,研究论文发展脉络
Chat Paper
正在生成论文摘要