LISSA: Lazy Initialization with Specialized Solver Aid
ASE 2022(2022)
摘要
Programs that deal with heap-allocated inputs are difficult to analyze with symbolic execution (SE). Lazy Initialization (LI) is an approach to SE that deals with heap-allocated inputs by starting SE over a fully symbolic heap, and initializing the inputs’ fields on demand, as the program under analysis accesses them. However, when the program’s assumed precondition has structural constraints over the inputs, operationally captured via repOK routines, LI may produce spurious symbolic structures, making SE traverse infeasible paths and undermining SE’s performance. repOK can only decide the feasibility of fully concrete structures, and thus previous work relied on manually crafted specifications designed to decide the (in)validity of partially symbolic inputs, to avoid producing spurious symbolic structures. However, these additional specifications require significant further effort from developers. To deal with this issue, we introduce SymSolve, a test generation based approach that, given a partially symbolic structure and a repOK, automatically decides if the structure can be extended to a fully concrete one satisfying repOK. As opposed to previous approaches, SymSolve does not require additional specifications. It works by exploring feasible concretizations of partially symbolic structures in a bounded-exhaustive manner, until it finds a fully concrete structure satisfying repOK, or it exhausts the search space, deeming the corresponding partially symbolic structure spurious. SymSolve exploits sound pruning of the search space, combined with symmetry breaking (to discard structures isomorphic to previously explored ones), to efficiently explore very large search spaces. We incorporate SymSolve into LI in order to decide the feasibility of partially symbolic inputs, obtaining our LISSA technique. We experimentally assess LISSA against related techniques over various case studies, consisting of programs with heap-allocated inputs with complex constraints. The results show that LISSA is faster and scales better than related techniques.
更多查看译文
关键词
Symbolic Execution, Lazy Initialization, Structural Constraint Solving
AI 理解论文
溯源树
样例
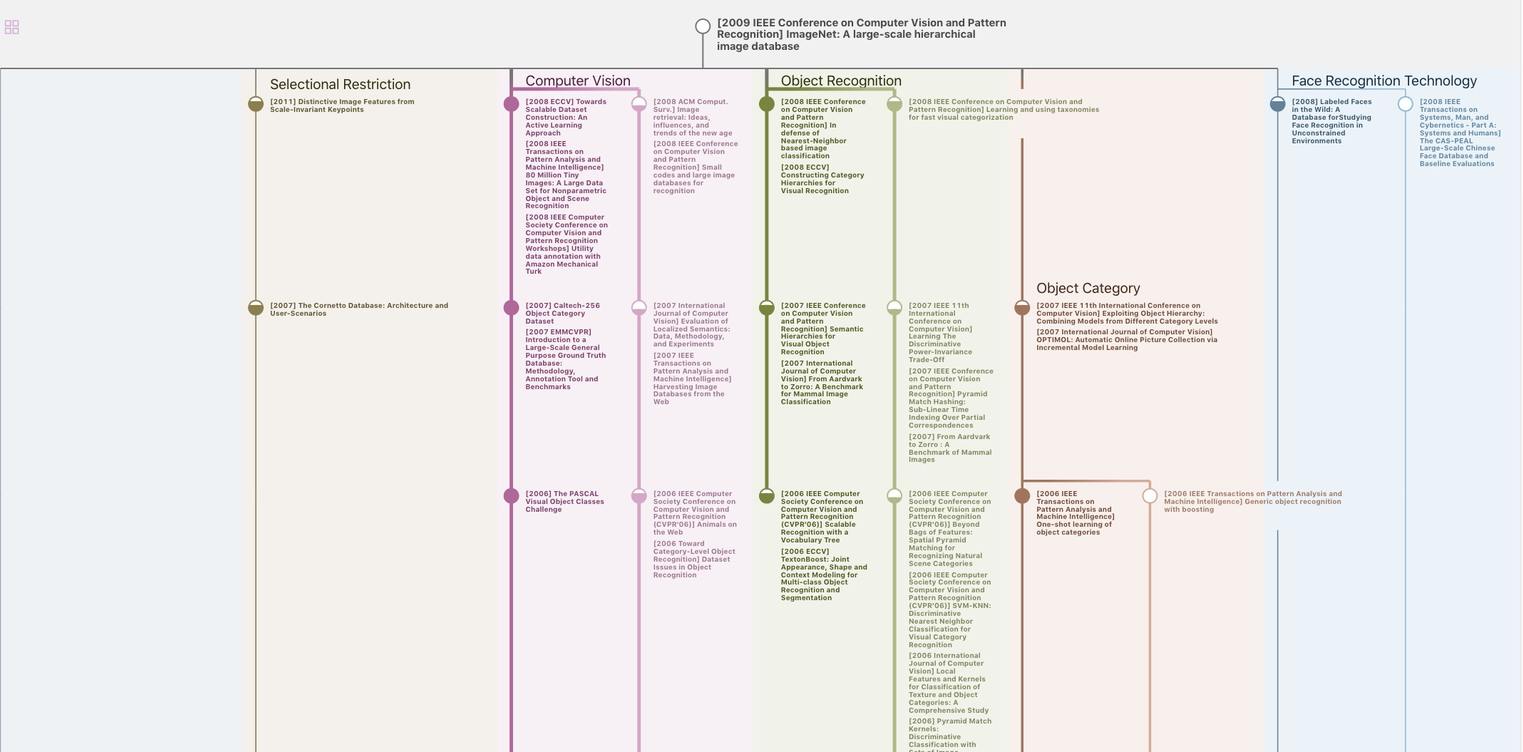
生成溯源树,研究论文发展脉络
Chat Paper
正在生成论文摘要