Semi-Supervised Authentically Distorted Image Quality Assessment With Consistency-Preserving Dual-Branch Convolutional Neural Network
IEEE TRANSACTIONS ON MULTIMEDIA(2023)
摘要
Recently, convolutional neural networks (CNNs) have provided a favoured prospect for authentically distorted image quality assessment (IQA). For good performance, most existing CNN-based methods rely on a large amount of labeled data for training, which is time-consuming and cumbersome to collect. By simultaneously exploiting few labeled data and many unlabeled data, we make a pioneering attempt to propose a semi-supervised framework (termed SSLIQA) with consistency-preserving dual-branch CNN for authentically distorted IQA in this paper. The proposed SSLIQA introduces a consistency-preserving strategy and transfers two kinds of consistency knowledge from the teacher branch to the student branch. Concretely, SSLIQA utilizes the sample prediction consistency to train the student to mimic output activations of individual examples represented by the teacher. Considering that subjects often refer to previous analogous cases to make scoring decisions, SSLIQA computes the semantic relation among different samples in a batch and encourages the consistency of sample semantic relation between two branches to explore extra quality-related information. Benefiting from the consistency-preserving strategy, we can exploit numerous unlabeled data to improve network's effectiveness and generalization. Experimental results on three authentically distorted IQA databases show that the proposed SSLIQA is stably effective under different student-teacher combinations and different labeled-to-unlabeled data ratios. In addition, it points out a new way on how to achieve higher performance with a smaller network.
更多查看译文
关键词
Distortion,Feature extraction,Training,Image quality,Task analysis,Social networking (online),Semantics,Image quality assessment,authentical distortion,consistency-preserving,semi-supervised
AI 理解论文
溯源树
样例
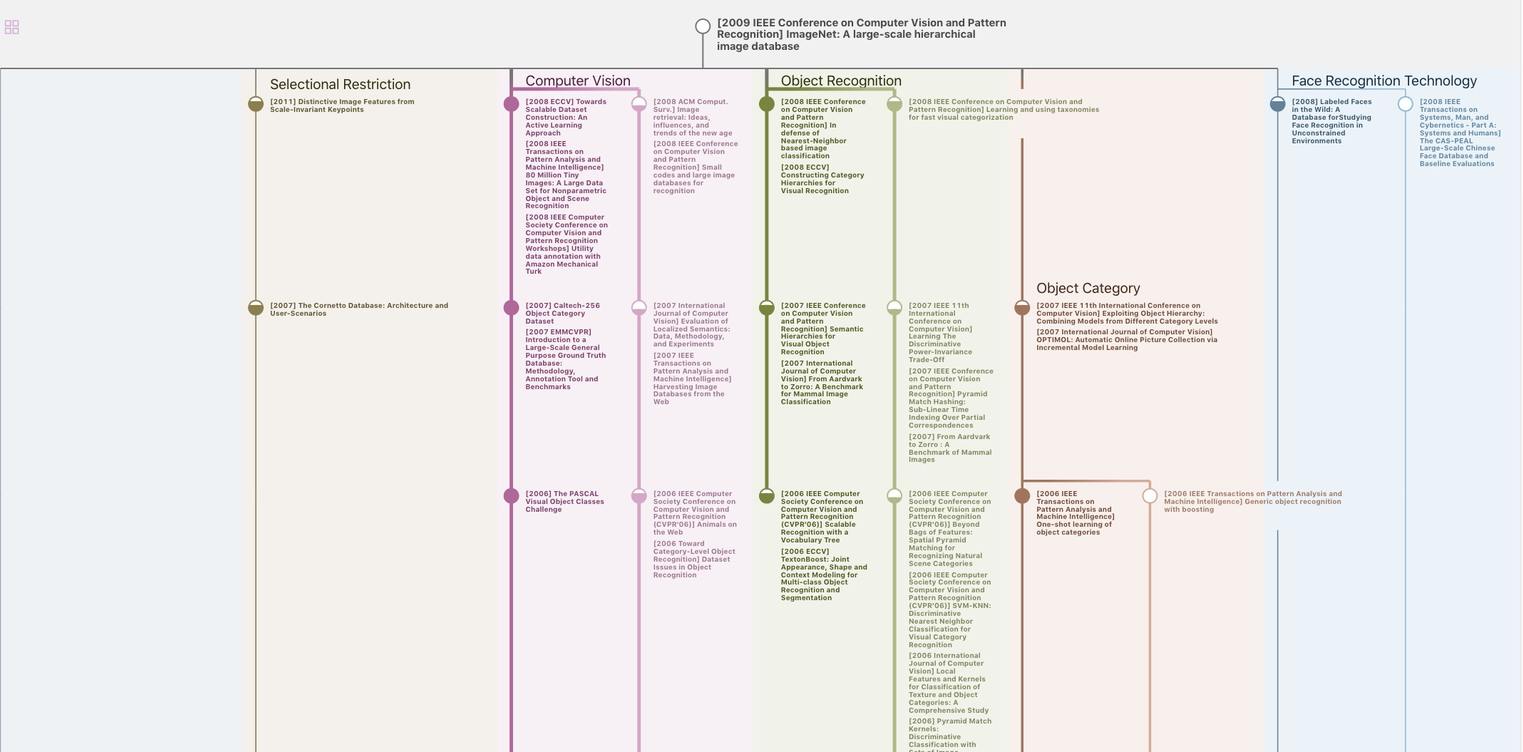
生成溯源树,研究论文发展脉络
Chat Paper
正在生成论文摘要