Flexible Job Classification with Zero-Shot Learning
CoRR(2022)
Abstract
Using a taxonomy to organize information requires classifying objects (documents, images, etc) with appropriate taxonomic classes. The flexible nature of zero-shot learning is appealing for this task because it allows classifiers to naturally adapt to taxonomy modifications. This work studies zero-shot multi-label document classification with fine-tuned language models under realistic taxonomy expansion scenarios in the human resource domain. Experiments show that zero-shot learning can be highly effective in this setting. When controlling for training data budget, zero-shot classifiers achieve a 12% relative increase in macro-AP when compared to a traditional multi-label classifier trained on all classes. Counterintuitively, these results suggest in some settings it would be preferable to adopt zero-shot techniques and spend resources annotating more documents with an incomplete set of classes, rather than spreading the labeling budget uniformly over all classes and using traditional classification techniques. Additional experiments demonstrate that adopting the well-known filter/re-rank decomposition from the recommender systems literature can significantly reduce the computational burden of high-performance zero-shot classifiers, empirically resulting in a 98% reduction in computational overhead for only a 2% relative decrease in performance. The evidence presented here demonstrates that zero-shot learning has the potential to significantly increase the flexibility of taxonomies and highlights directions for future research.
MoreTranslated text
AI Read Science
Must-Reading Tree
Example
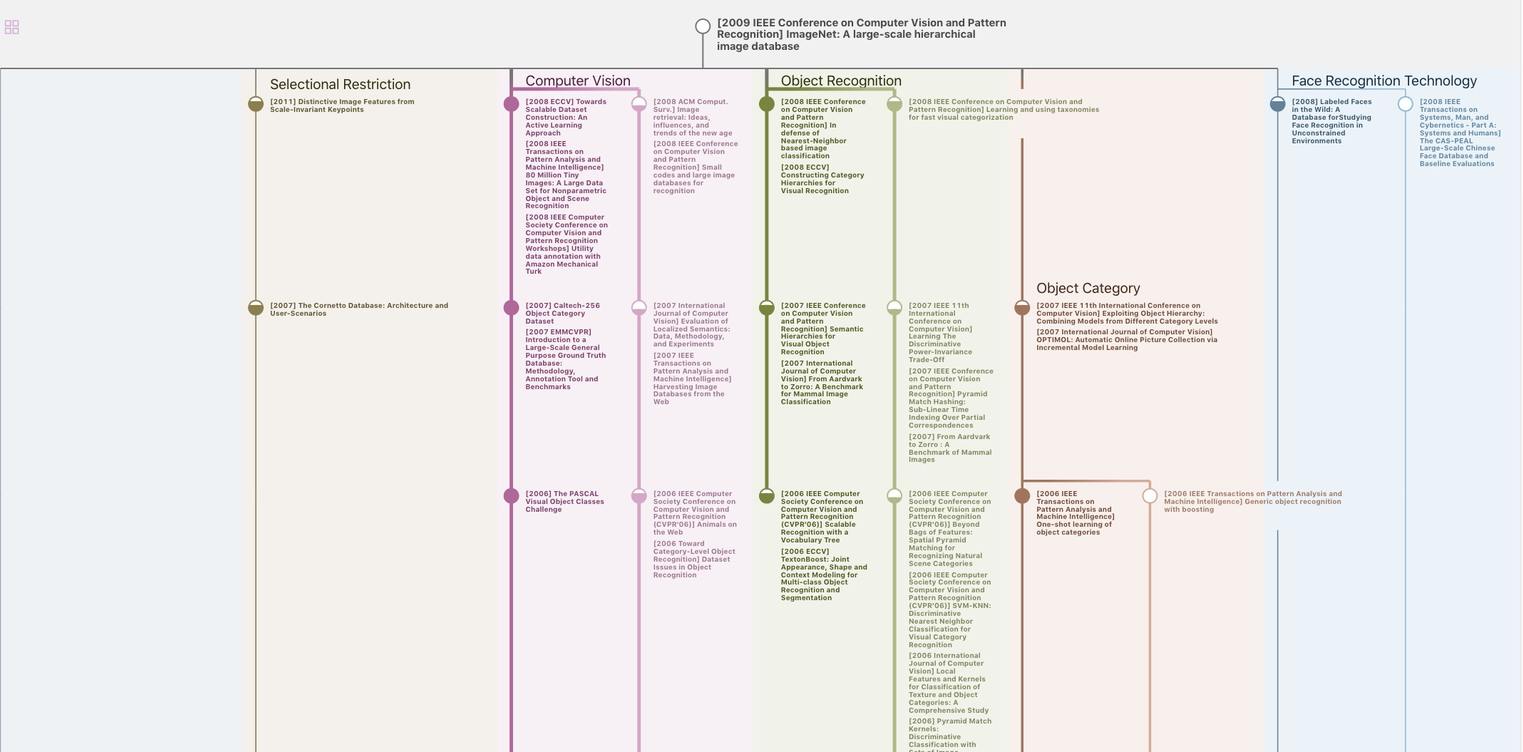
Generate MRT to find the research sequence of this paper
Chat Paper
Summary is being generated by the instructions you defined