Learning variational models with unrolling and bilevel optimization
ANALYSIS AND APPLICATIONS(2024)
摘要
In this paper, we consider the problem of learning variational models in the context of supervised learning via risk minimization. Our goal is to provide a deeper understanding of the two approaches of learning of variational models via bilevel optimization and via algorithm unrolling. The former considers the variational model as a lower level optimization problem below the risk minimization problem, while the latter replaces the lower level optimization problem by an algorithm that solves said problem approximately. Both approaches are used in practice, but unrolling is much simpler from a computational point of view. To analyze and compare the two approaches, we consider a simple toy model, and compute all risks and the respective estimators explicitly. We show that unrolling can be better than the bilevel optimization approach, but also that the performance of unrolling can depend significantly on further parameters, sometimes in unexpected ways: While the stepsize of the unrolled algorithm matters a lot (and learning the stepsize gives a significant improvement), the number of unrolled iterations plays a minor role.
更多查看译文
关键词
Algorithm unrolling,bilevel optimization,supervised learning,risk minimization
AI 理解论文
溯源树
样例
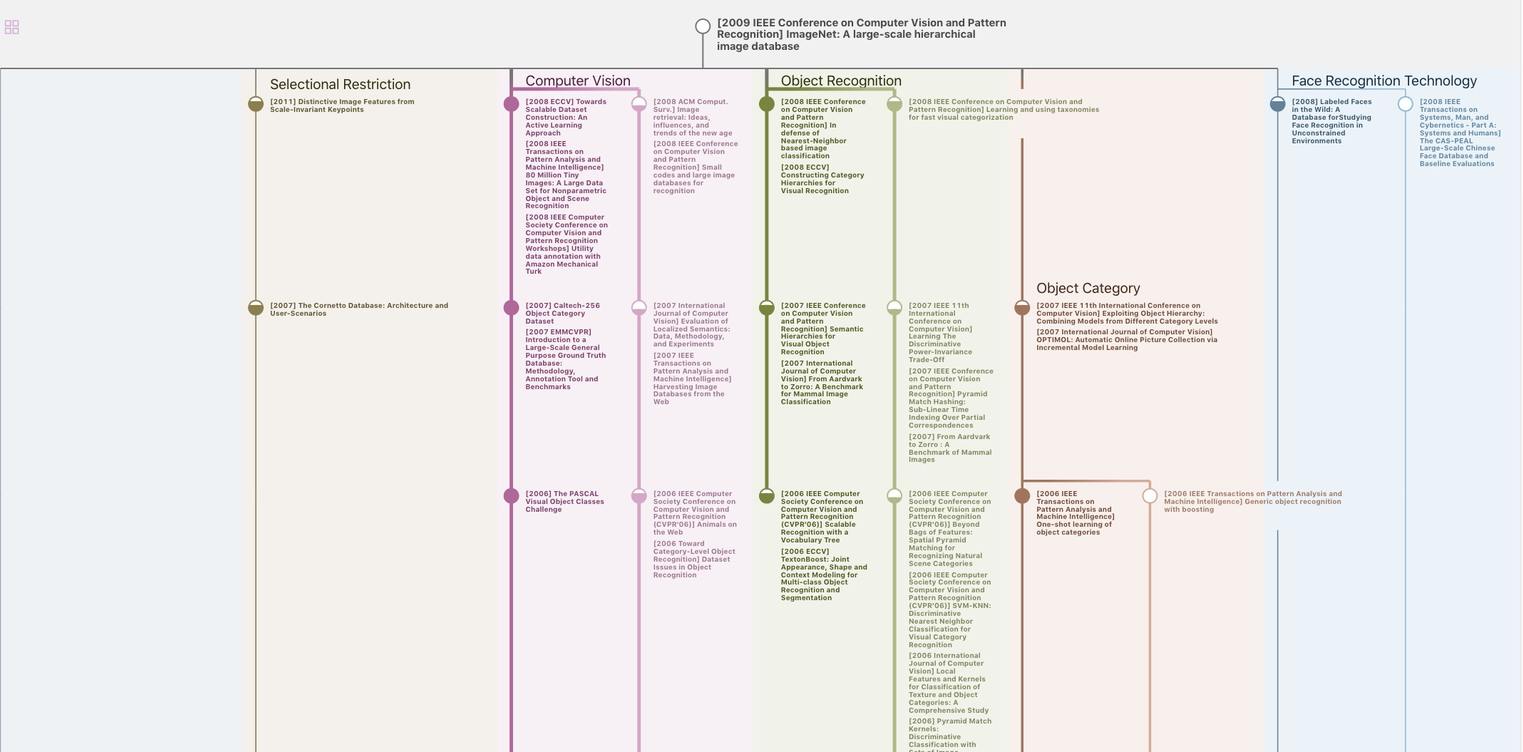
生成溯源树,研究论文发展脉络
Chat Paper
正在生成论文摘要