On Projections to Linear Subspaces.
Similarity Search and Applications (SISAP)(2022)
摘要
The merit of projecting data onto linear subspaces is well known from, e.g., dimension reduction. One key aspect of subspace projections, the maximum preservation of variance (principal component analysis), has been thoroughly researched and the effect of random linear projections on measures such as intrinsic dimensionality still is an ongoing effort. In this paper, we investigate the less explored depths of linear projections onto explicit subspaces of varying dimensionality and the expectations of variance that ensue. The result is a new family of bounds for Euclidean distances and inner products. We showcase the quality of these bounds as well as investigate the intimate relation to intrinsic dimensionality estimation.
更多查看译文
关键词
projections,linear
AI 理解论文
溯源树
样例
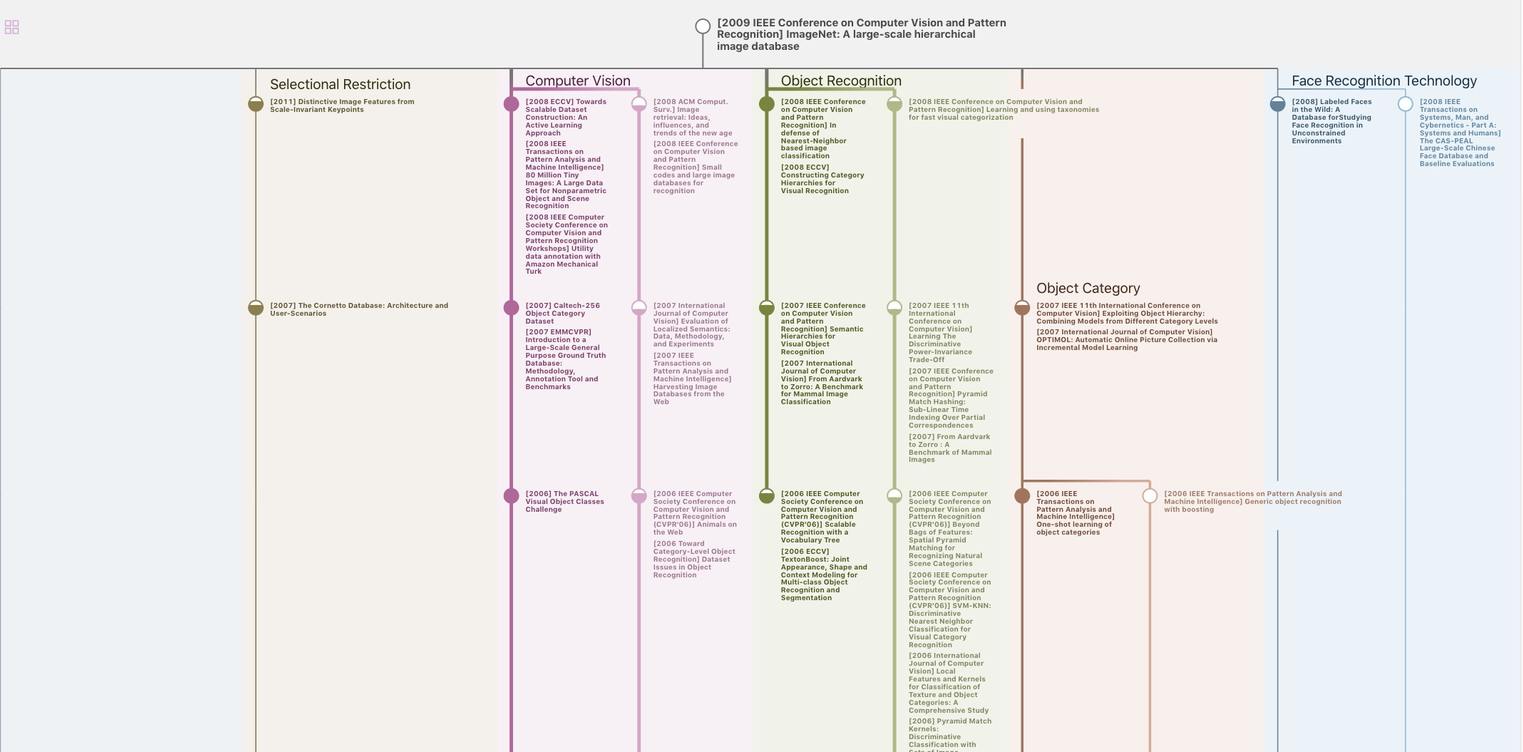
生成溯源树,研究论文发展脉络
Chat Paper
正在生成论文摘要