Knowledge Distillation to Ensemble Global and Interpretable Prototype-Based Mammogram Classification Models
MEDICAL IMAGE COMPUTING AND COMPUTER ASSISTED INTERVENTION, MICCAI 2022, PT III(2022)
Abstract
State-of-the-art (SOTA) deep learning mammogram classifiers, trained with weakly-labelled images, often rely on global models that produce predictions with limited interpretability, which is a key barrier to their successful translation into clinical practice. On the other hand, prototype-based models improve interpretability by associating predictions with training image prototypes, but they are less accurate than global models and their prototypes tend to have poor diversity. We address these two issues with the proposal of BRAIxPro-toPNet++, which adds interpretability to a global model by ensembling it with a prototype-based model. BRAIxProtoPNet++ distills the knowledge of the global model when training the prototype-based model with the goal of increasing the classification accuracy of the ensemble. Moreover, we propose an approach to increase prototype diversity by guaranteeing that all prototypes are associated with different training images. Experiments on weakly-labelled private and public datasets show that BRAIxProtoPNet++ has higher classification accuracy than SOTA global and prototype-based models. Using lesion localisation to assess model interpretability, we show BRAIxProtoPNet++ is more effective than other prototype-based models and post-hoc explanation of global models. Finally, we show that the diversity of the prototypes learned by BRAIxProtoPNet++ is superior to SOTA prototype-based approaches.
MoreTranslated text
Key words
Interpretability,Explainability,Prototype-based model,Mammogram classification,Breast cancer diagnosis,Deep learning
AI Read Science
Must-Reading Tree
Example
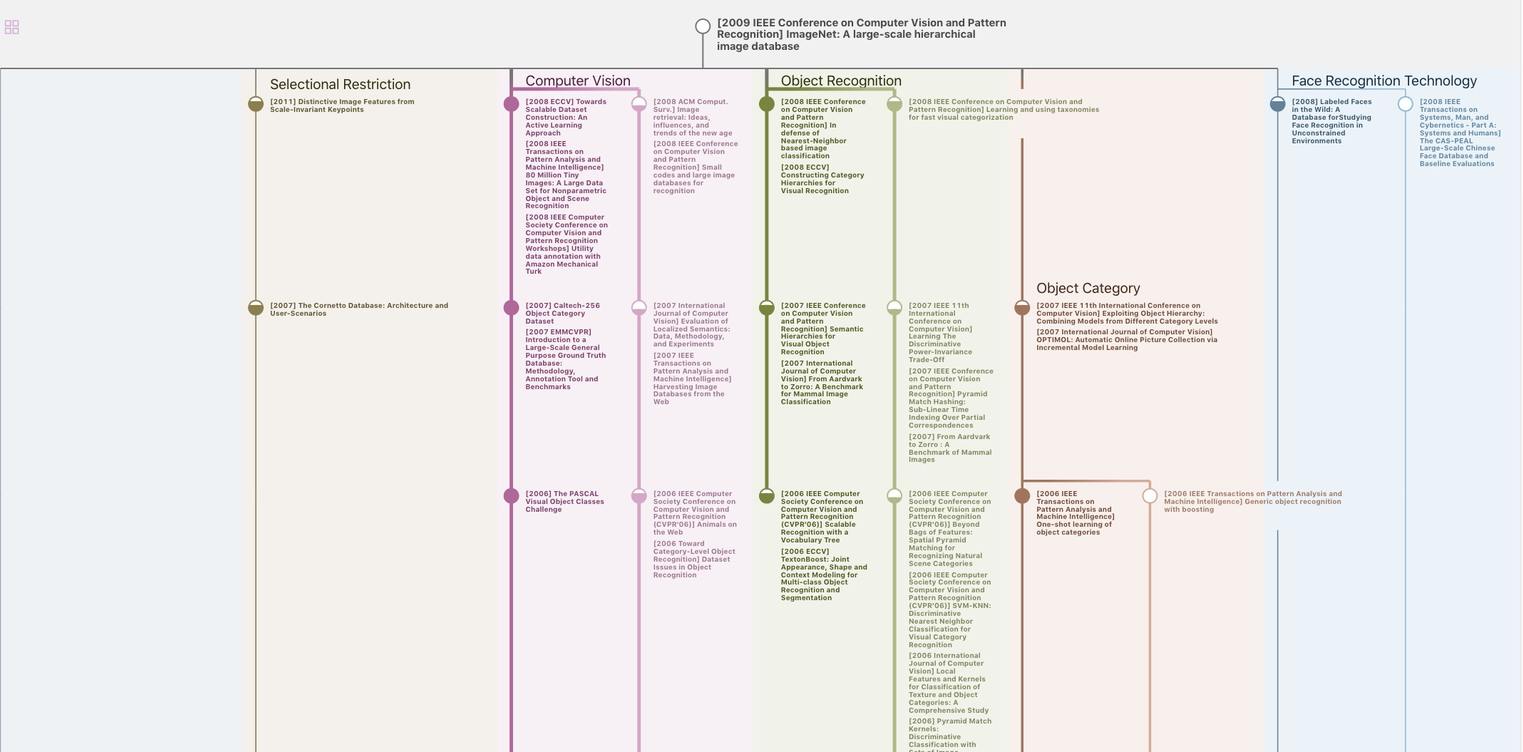
Generate MRT to find the research sequence of this paper
Chat Paper
Summary is being generated by the instructions you defined